Classification of Mobile Laser Scanning Point Cloud in an Urban Environment Using kNN and Random Forest
Innovations in Smart Cities Applications Volume 5Lecture Notes in Networks and Systems(2022)
摘要
This study presents the supervised classification of point clouds collected through a vehicle-based Mobile Laser Scanning (MLS) system in an urban environment. A benchmark dataset representing the Technical University of Munich (TUM) City Campus was utilized. The main contribution of this paper is to reveal the performance difference between the 2D and 3D k-nearest neighbor (kNN) information on the point-based classification of an MLS system using local geometric and eigen- based features. Random Forest (RF) classifier is utilized for the supervised classification of 8 classes predefined in the benchmark set: artificial terrain, natural terrain, high vegetation, low vegetation, building, hardscape, artifact, and vehicle. As a result, we achieve an impressive classification performance using the 2D kNN information. We reveal that 2D kNN with 12 features not only improves the processing speed but also provides an improvement of 7.3% compared to the 3D kNN, and at least 2.8% overall accuracy improvement compared to the state-of-the-art approaches previously developed and tested using the benchmark.
更多查看译文
关键词
random forest,laser,urban environment
AI 理解论文
溯源树
样例
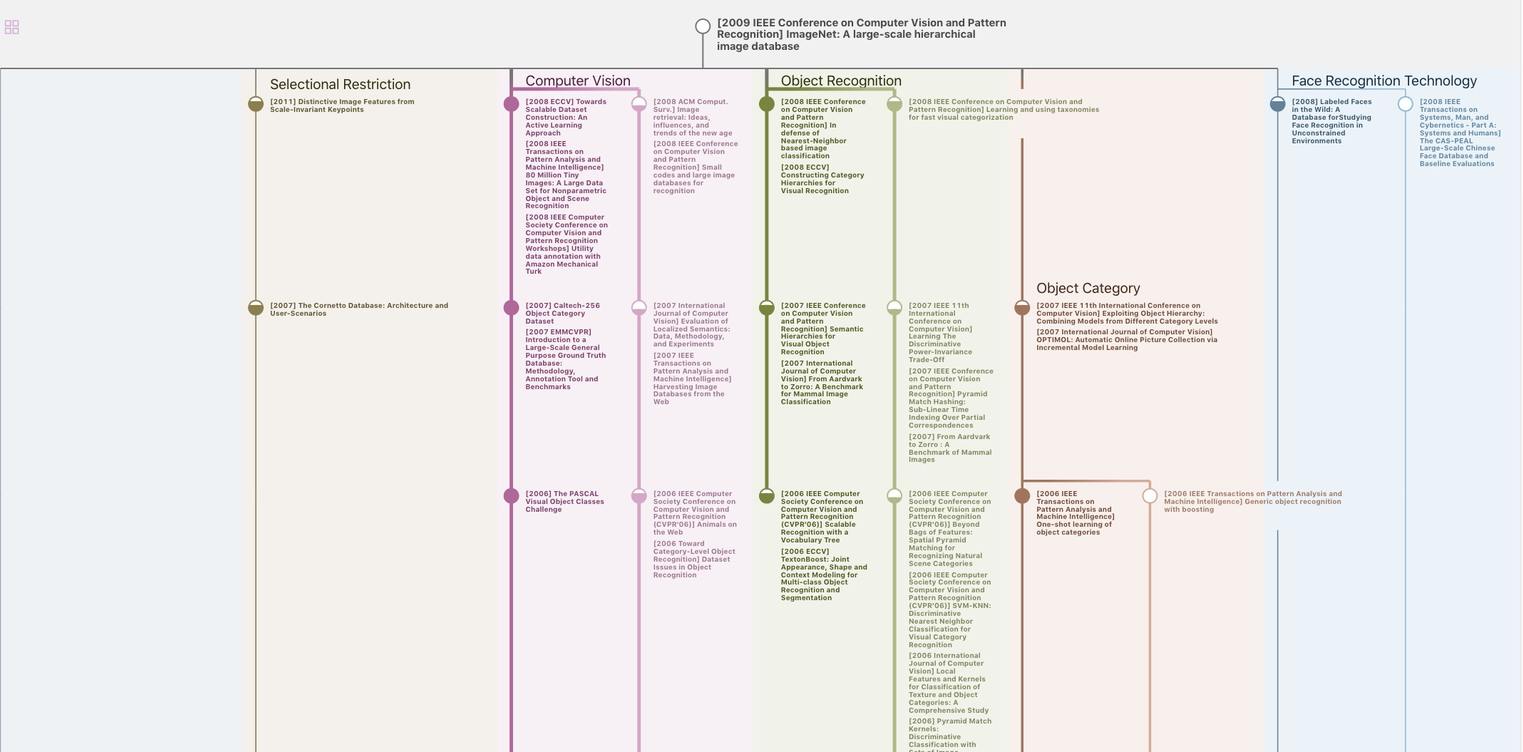
生成溯源树,研究论文发展脉络
Chat Paper
正在生成论文摘要