Effect of biologically-motivated energy constraints on liquid state machine dynamics and classification performance.
Neuromorph. Comput. Eng.(2022)
摘要
Abstract Equipping edge devices with intelligent behavior opens up new possibilities for automating the decision making in extreme size, weight, and power-constrained application domains. To this end, several recent lines of research are aimed at the design of artificial intelligence hardware accelerators that have significantly reduced footprint and power demands compared to conventional CPU/GPU systems. However, despite some key advancements, the majority of work in this area assumes that there is an unlimited supply of energy available for computation, which is not realistic in the case of battery-powered and energy harvesting devices. In this paper, we address this gap by exploring the computational effects of energy constraints on a popular class of brain-inspired spiking neural networks–liquid state machines (LSMs). Energy constraints were applied by limiting the spiking activity in subsets of LSM neurons. We tested our designs on two biosignal processing tasks: epileptic seizure detection and biometric gait identification. For both tasks, we show that energy constraints can significantly improve classification accuracy. This demonstrates that in the design of neuromorphic systems, reducing energy and increasing performance are not always competing goals.
更多查看译文
AI 理解论文
溯源树
样例
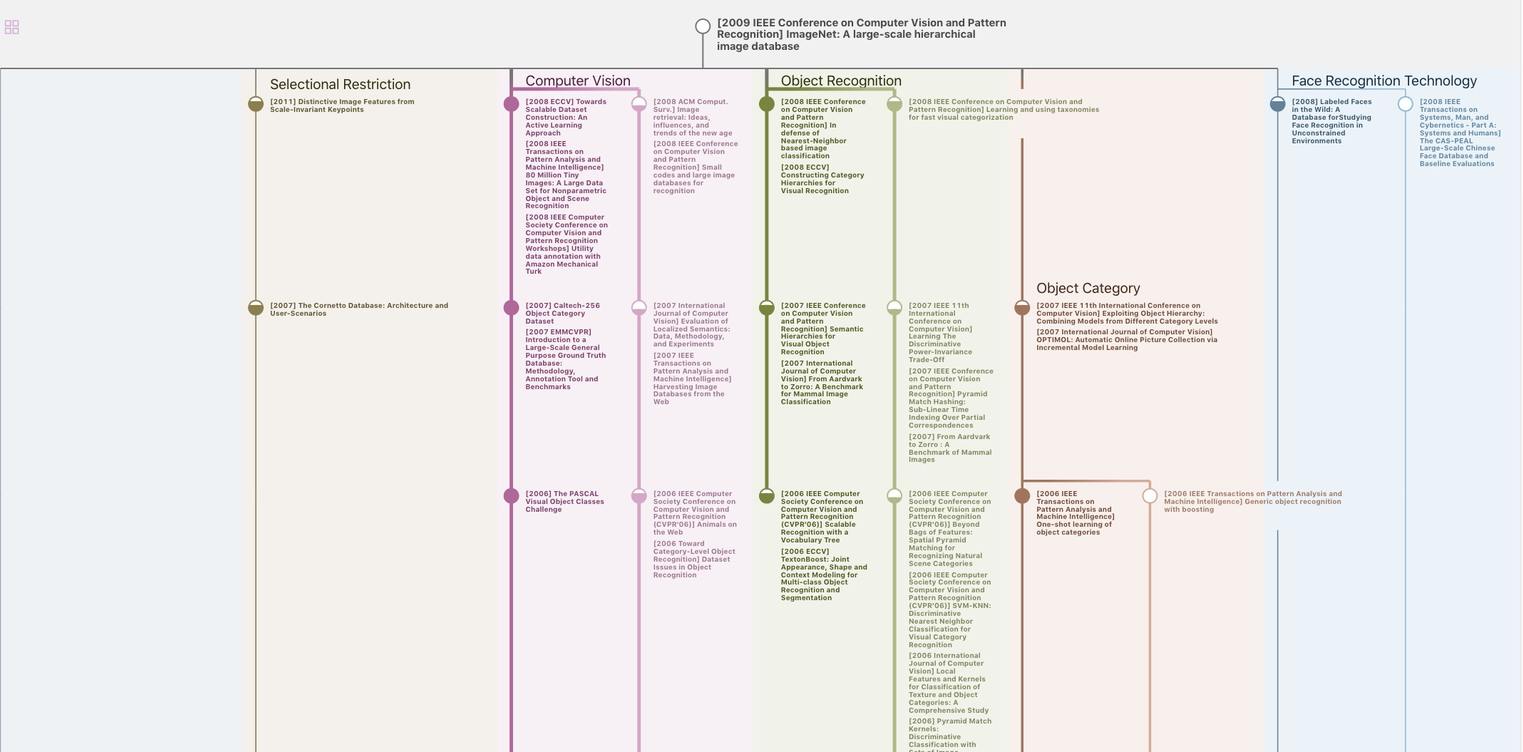
生成溯源树,研究论文发展脉络
Chat Paper
正在生成论文摘要