Explainable Deep Reinforcement Learning: State of the Art and Challenges.
ACM Comput. Surv.(2023)
摘要
Interpretability, explainability and transparency are key issues to introducing Artificial Intelligence methods in many critical domains: This is important due to ethical concerns and trust issues strongly connected to reliability, robustness, auditability and fairness, and has important consequences towards keeping the human in the loop in high levels of automation, especially in critical cases for decision making, where both (human and the machine) play important roles. While the research community has given much attention to explainability of closed (or black) prediction boxes, there are tremendous needs for explainability of closed-box methods that support agents to act autonomously in the real world. Reinforcement learning methods, and especially their deep versions, are such closed-box methods. In this article we aim to provide a review of state of the art methods for explainable deep reinforcement learning methods, taking also into account the needs of human operators - i.e., of those that take the actual and critical decisions in solving real-world problems. We provide a formal specification of the deep reinforcement learning explainability problems, and we identify the necessary components of a general explainable reinforcement learning framework. Based on these, we provide a comprehensive review of state of the art methods, categorizing them in classes according to the paradigm they follow, the interpretable models they use, and the surface representation of explanations provided. The article concludes identifying open questions and important challenges.
更多查看译文
关键词
Deep learning,deep reinforcement learning,interpretability,explainability,transparency
AI 理解论文
溯源树
样例
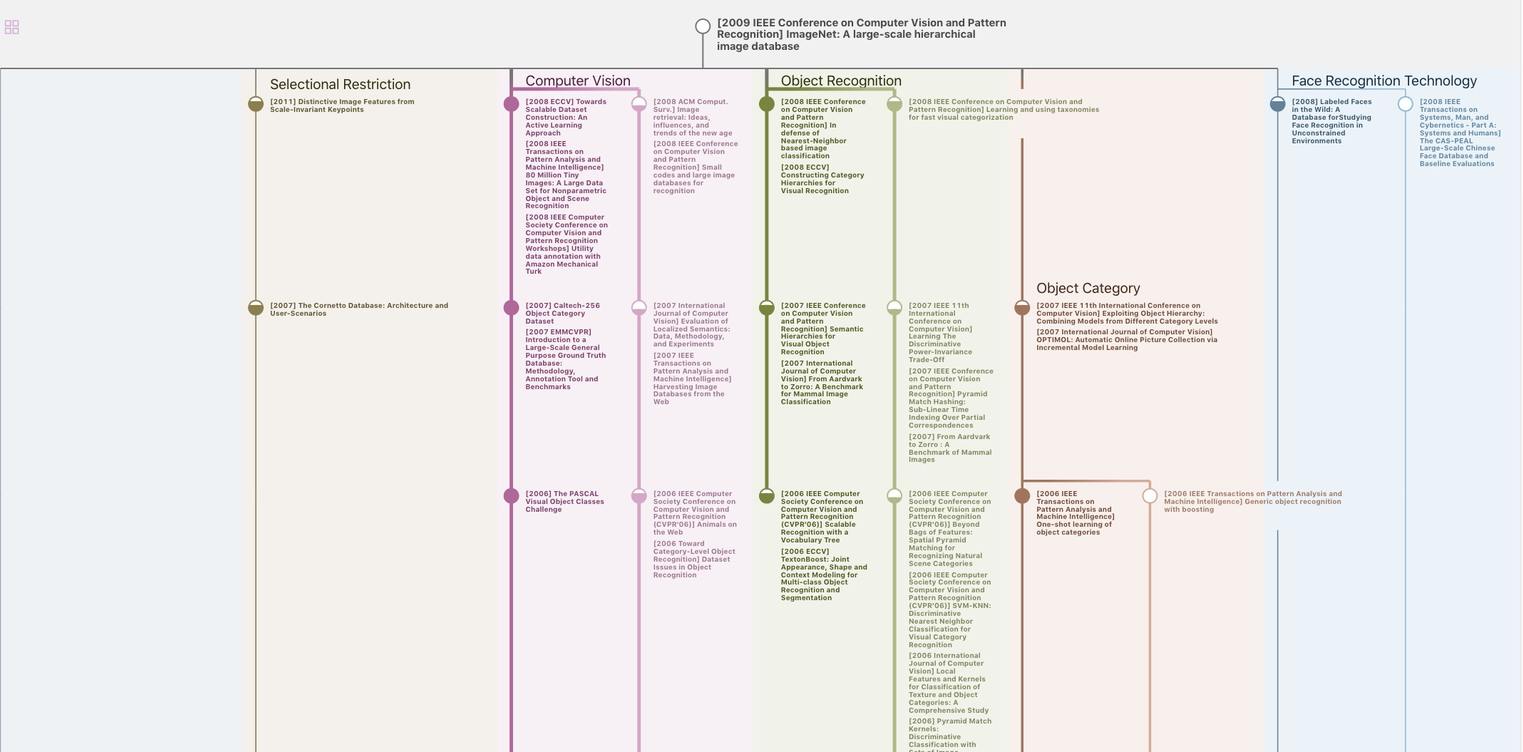
生成溯源树,研究论文发展脉络
Chat Paper
正在生成论文摘要