Evaluating live performance of machine learning based prediction models for different clinical risks: a study of live systems in different hospitals (Preprint)
Journal of Medical Internet Research(2021)
摘要
Machine learning algorithms are currently used in a wide array of clinical domains to produce models that can predict clinical risk events. Most models are developed and evaluated with retrospective data, very few are evaluated in a clinical workflow, and even fewer report performances in different hospitals. We provide detailed evaluations of clinical risk prediction models in live clinical workflows for three different use cases in three different hospitals.The main objective of this study is to evaluate the clinical risk prediction models in live clinical workflows and compare with their performance on retrospective data. We also aimed at generalizing the results by applying our investigation to three different use cases in three different hospitals.We trained clinical risk prediction models for three use cases (delirium, sepsis and acute kidney injury (AKI)) in three different hospitals with retrospective data. We use machine learning and specifically deep learning to train models that are based on the Transformer model. The models are trained using a calibration tool that is common for all hospitals and use cases. The models have a common design but are calibrated using the hospital's specific data. The models are deployed in these three hospitals and used in daily clinical practice. The predictions made by these models are logged and correlated with the diagnosis at discharge. We compared the performance with evaluations on retrospective data and conducted cross-hospital evaluations.The performance of the prediction models with data from live clinical workflows is similar to the performance with retrospective data. The average value of the area under the receiver-operating characteristic curve (AUROC) decreases slightly by 0.6 percentage point (from 94.8% to 94.2% at discharge). The cross-hospital evaluations exhibit severely reduced performance, the averaged AUROC decreased by 8 percentage points (from 94.2% to 86.3% at discharge), which indicates the importance of model calibration with data from the deployment hospital.Calibrating the prediction model with data from different deployment hospitals leads to a good performance in live settings. The performance degradation in the cross-hospital evaluation indicates limitations in developing a generic model for different hospitals. Designing a generic model development process to generate specialized prediction models for each hospital guarantees the model performance in different hospitals.
更多查看译文
AI 理解论文
溯源树
样例
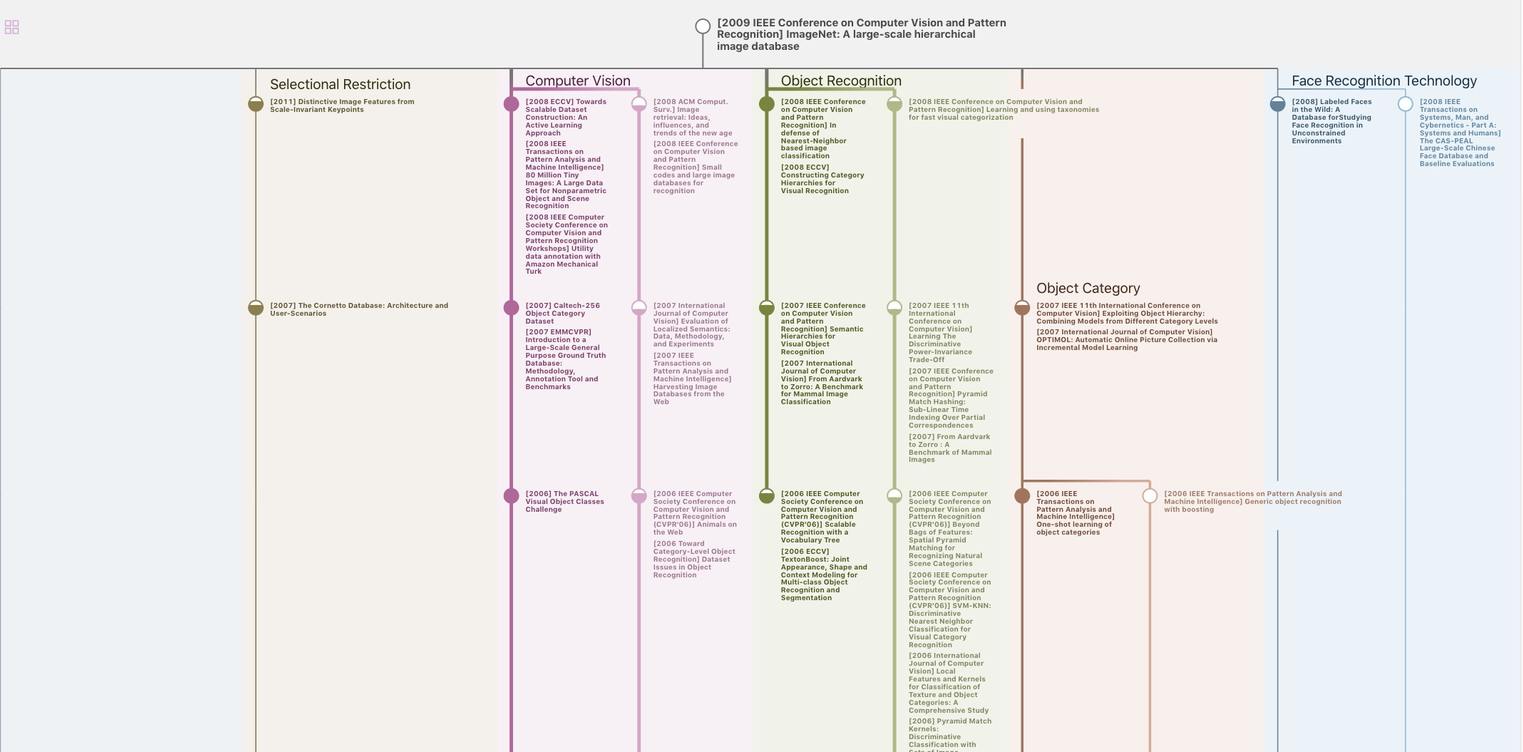
生成溯源树,研究论文发展脉络
Chat Paper
正在生成论文摘要