Deep learning with invariant feature based species classification in underwater environments
Multimedia Tools and Applications(2024)
摘要
Researchers are paying more attention these days to research on the detection and classification of underwater species from images. The main goal of the researchers is to make a pre-processing algorithm that uses an enhancement mechanism to find the exact region of species. It is crucial for marine researchers and scientists to estimate the region of species for classification on a regular basis, but this is a challenging task due to uncleanly captured images. The main causes of such a problem are variation in light of the underwater environment, species concealment, irregular backgrounds, low resolution, and indirect variations between some species patterns. To address these issues, we propose an Invariant Feature-based Species Classification (IFSC) model that employs a pattern-net-based Convolutional Neural Network (CNN) as a deep learning model in an underwater environment. We focused on two types of species: octopus and crabs, each with eight subclasses, and the dataset used was self-collected from the Poppe Image Marine Iconography. To achieve maximum classification accuracy, this study focuses on appropriate segmentation and invariant feature extraction. Following the extraction of invariant features, the concept of a genetic algorithm (GA) is used to select only the most relevant features based on their class. The invariant feature extraction approach known as the Speed Up Robust Feature (SURF) descriptor performed well, and the model achieved an overall accuracy of 95.04%, which is higher than the existing work of 1.71%. As far as we know, the results we got are the best ones that have been published on the collected dataset in the past few years, which shows that our strategy works better than others.
更多查看译文
关键词
Underwater species,Image enhancement,Segmentation,Surf,Genetic algorithm,Deep learning,Convolutional Neural Network
AI 理解论文
溯源树
样例
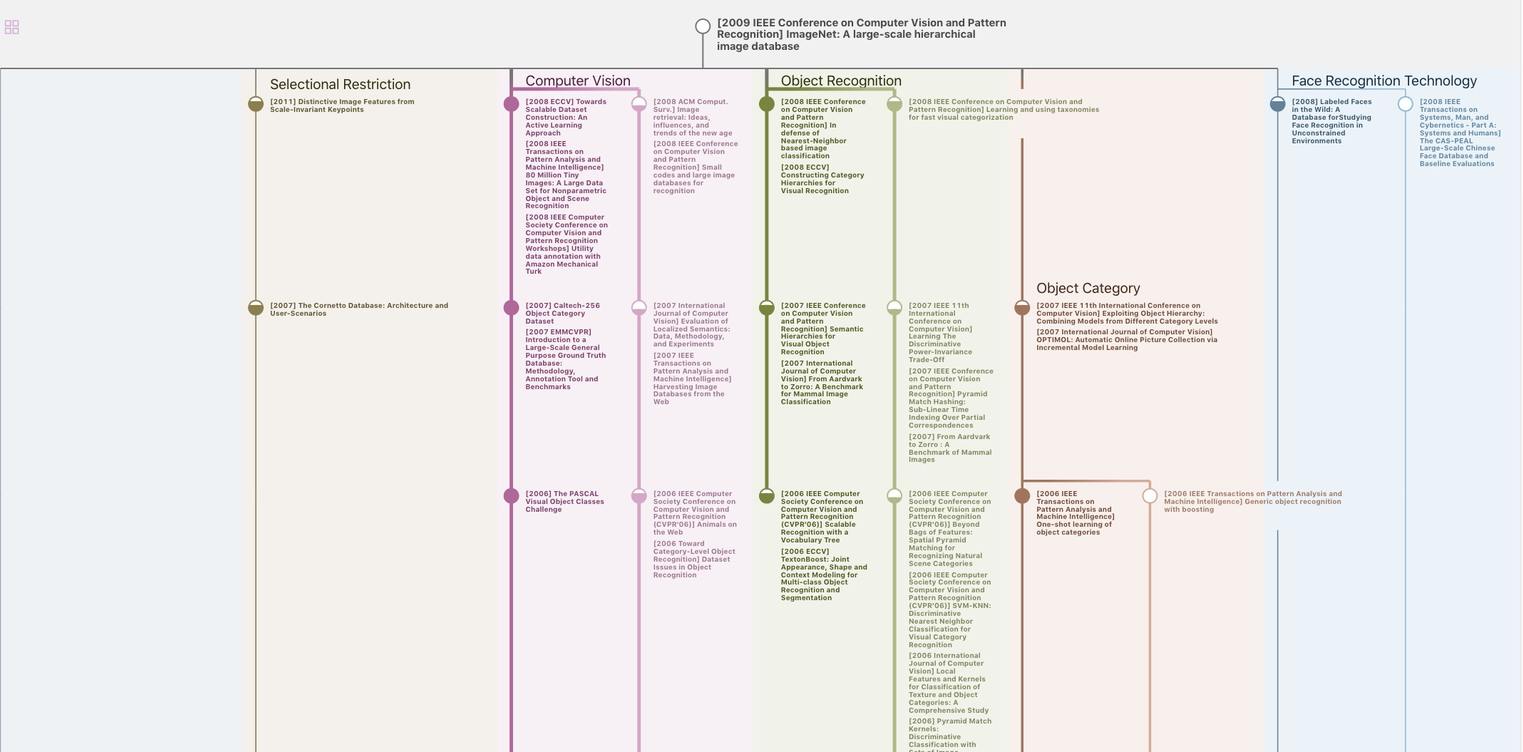
生成溯源树,研究论文发展脉络
Chat Paper
正在生成论文摘要