A cost-sensitive Imprecise Credal Decision Tree based on Nonparametric Predictive Inference
Applied Soft Computing(2022)
摘要
Classifiers sometimes return a set of values of the class variable since there is not enough information to point to a single class value. These classifiers are known as imprecise classifiers. Decision Trees for Imprecise Classification were proposed and adapted to consider the error costs when classifying new instances. In this work, we present a new cost-sensitive Decision Tree for Imprecise Classification that considers the error costs by weighting instances, also considering such costs in the tree building process. Our proposed method uses the Nonparametric Predictive Inference Model, a nonparametric model that does not assume previous knowledge about the data, unlike previous imprecise probabilities models. We show that our proposal might give more informative predictions than the existing cost-sensitive Decision Tree for Imprecise Classification. Experimental results reveal that, in Imprecise Classification, our proposed cost-sensitive Decision Tree significantly outperforms the one proposed so far; even though the cost of erroneous classifications is higher with our proposal, it tends to provide more informative predictions.
更多查看译文
关键词
Cost-sensitive classifiers,Imprecise Classification,Error costs,Cost-sensitive Imprecise Credal Decision Tree,Nonparametric Predictive Inference
AI 理解论文
溯源树
样例
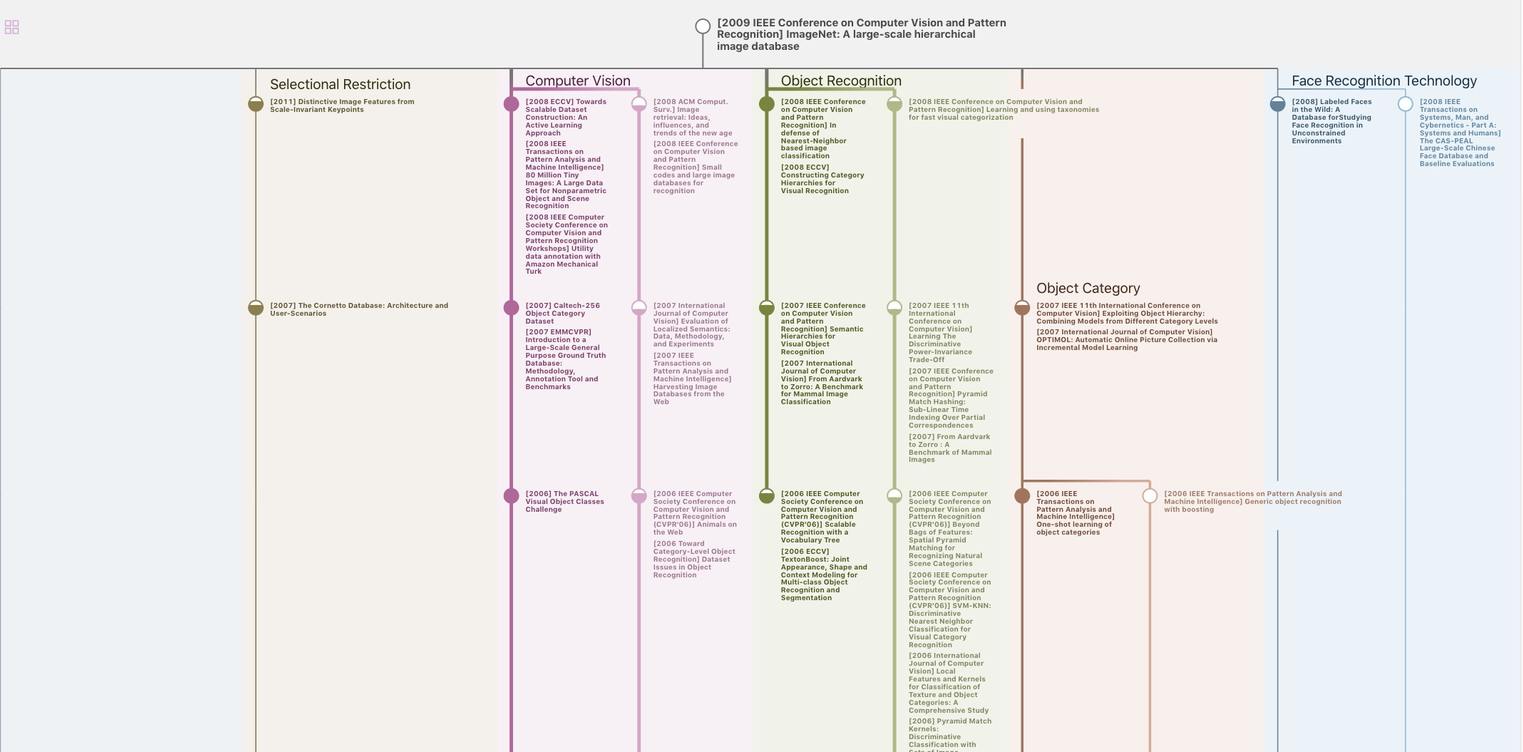
生成溯源树,研究论文发展脉络
Chat Paper
正在生成论文摘要