Switchable Representation Learning Framework With Self-Compatibility
CVPR 2023(2023)
摘要
Real-world visual search systems involve deployments on multiple platforms with different computing and storage resources. Deploying a unified model that suits the minimal-constrain platforms leads to limited accuracy. It is expected to deploy models with different capacities adapting to the resource constraints, which requires features extracted by these models to be aligned in the metric space. The method to achieve feature alignments is called "compatible learning". Existing research mainly focuses on the one-to-one compatible paradigm, which is limited in learning compatibility among multiple models. We propose a Switchable representation learning Framework with Self-Compatibility (SFSC). SFSC generates a series of compatible sub-models with different capacities through one training process. The optimization of sub-models faces gradients conflict, and we mitigate this problem from the perspective of the magnitude and direction. We adjust the priorities of sub-models dynamically through uncertainty estimation to co-optimize sub-models properly. Besides, the gradients with conflicting directions are projected to avoid mutual interference. SFSC achieves state-of-the-art performance on the evaluated datasets.
更多查看译文
关键词
representation learning,self-compatibility
AI 理解论文
溯源树
样例
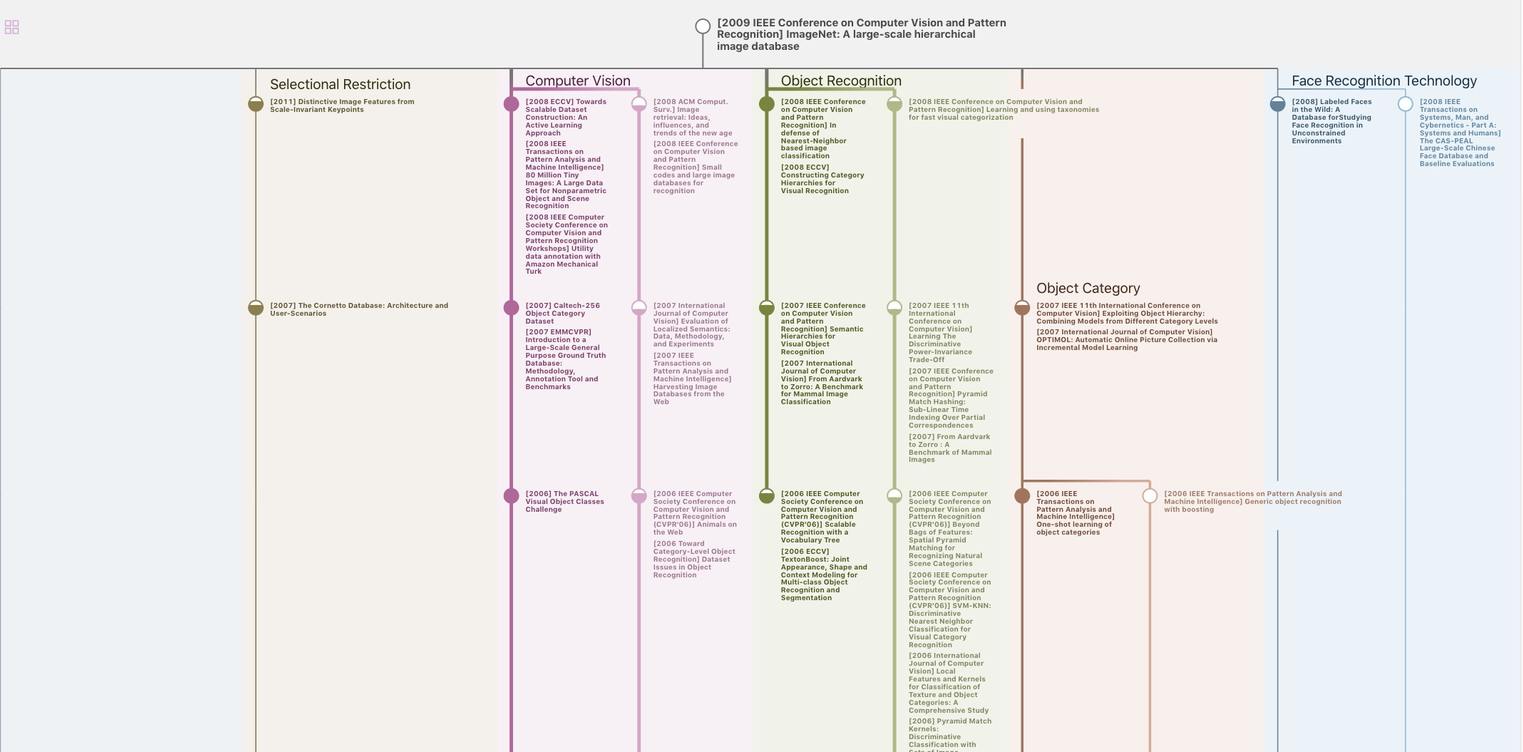
生成溯源树,研究论文发展脉络
Chat Paper
正在生成论文摘要