Balancing Cost and Quality: An Exploration of Human-in-the-Loop Frameworks for Automated Short Answer Scoring.
International Conference on Artificial Intelligence in Education (AIED)(2022)
摘要
Short answer scoring (SAS) is the task of grading short text written by a learner. In recent years, deep-learning-based approaches have substantially improved the performance of SAS models, but how to guarantee high-quality predictions still remains a critical issue when applying such models to the education field. Towards guaranteeing high-quality predictions, we present the first study of exploring the use of human-in-the-loop framework for minimizing the grading cost while guaranteeing the grading quality by allowing a SAS model to share the grading task with a human grader. Specifically, by introducing a confidence estimation method for indicating the reliability of the model predictions, one can guarantee the scoring quality by utilizing only predictions with high reliability for the scoring results and casting predictions with low reliability to human graders. In our experiments, we investigate the feasibility of the proposed framework using multiple confidence estimation methods and multiple SAS datasets. We find that our human-in-the-loop framework allows automatic scoring models and human graders to achieve the target scoring quality.
更多查看译文
关键词
Neural network,Natural language processing,Automated short answer scoring,Confidence estimation
AI 理解论文
溯源树
样例
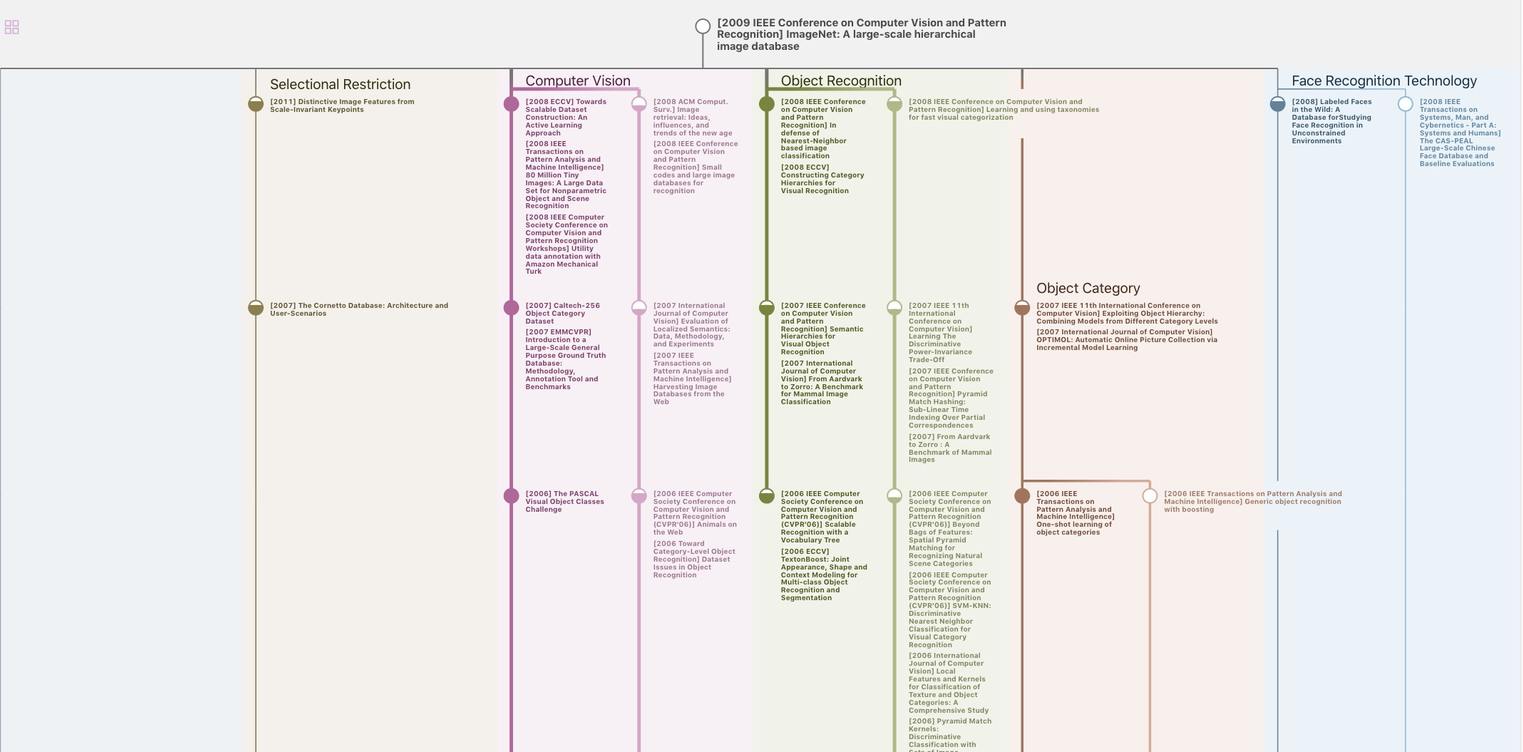
生成溯源树,研究论文发展脉络
Chat Paper
正在生成论文摘要