Towards Better Understanding with Uniformity and Explicit Regularization of Embeddings in Embedding-based Neural Topic Models
IEEE International Joint Conference on Neural Network (IJCNN)(2022)
摘要
Embedding-based neural topic models could explicitly represent words and topics by embedding them to a homogeneous feature space, which shows higher interpretability. However, there are no explicit constraints for the training of embeddings, leading to a larger optimization space. Also, a clear description of the changes in embeddings and the impact on model performance is still lacking. In this paper, we propose an embedding regularized neural topic model, which applies the specially designed training constraints on word embedding and topic embedding to reduce the optimization space of parameters. To reveal the changes and roles of embeddings, we introduce \textbf{uniformity} into the embedding-based neural topic model as the evaluation metric of embedding space. On this basis, we describe how embeddings tend to change during training via the changes in the uniformity of embeddings. Furthermore, we demonstrate the impact of changes in embeddings in embedding-based neural topic models through ablation studies. The results of experiments on two mainstream datasets indicate that our model significantly outperforms baseline models in terms of the harmony between topic quality and document modeling. This work is the first attempt to exploit uniformity to explore changes in embeddings of embedding-based neural topic models and their impact on model performance to the best of our knowledge.
更多查看译文
关键词
neural topic model,word embedding,topic embedding,interpretability,neural network
AI 理解论文
溯源树
样例
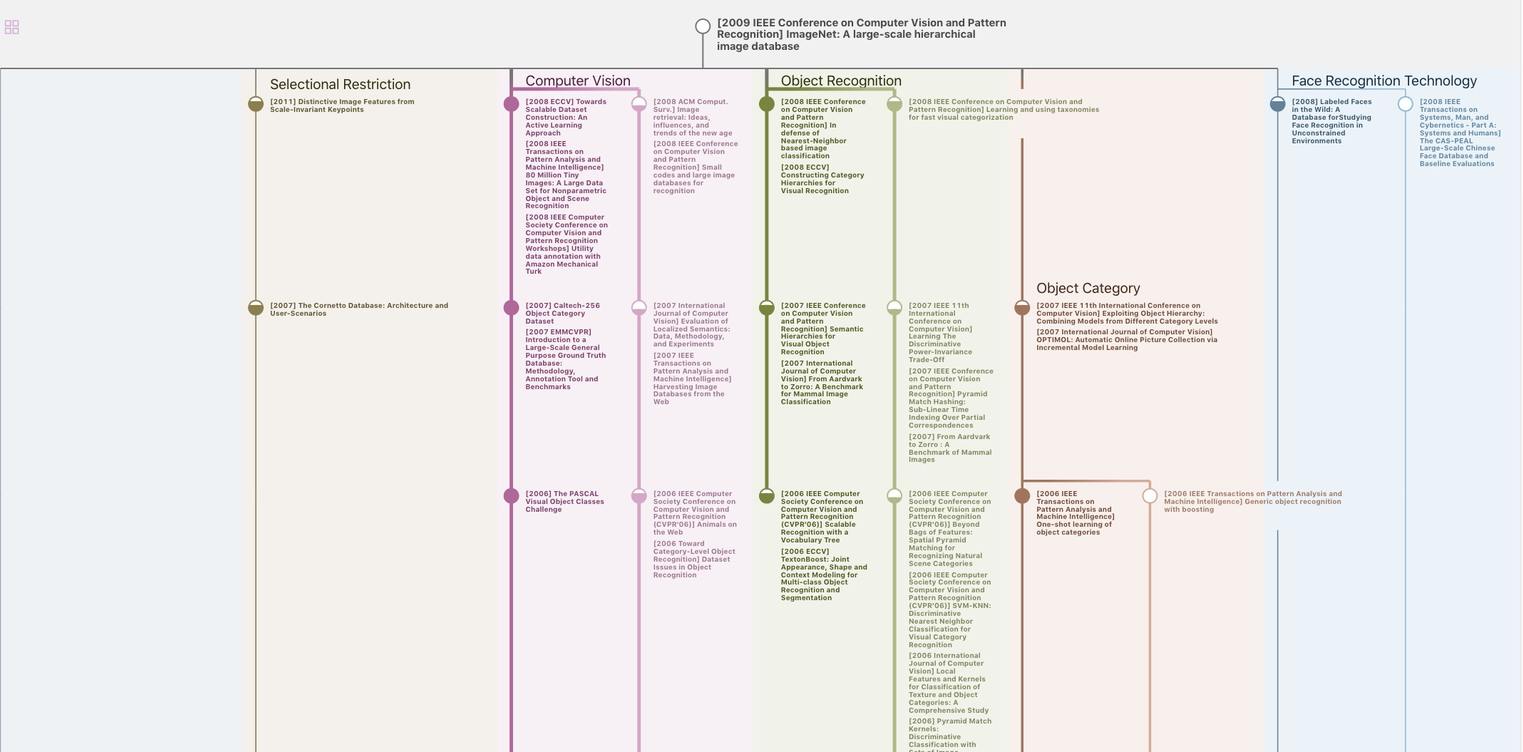
生成溯源树,研究论文发展脉络
Chat Paper
正在生成论文摘要