Reconstructing Training Data from Trained Neural Networks
NeurIPS 2022(2022)
摘要
Understanding to what extent neural networks memorize training data is an intriguing question with practical and theoretical implications. In this paper we show that in some cases a significant fraction of the training data can in fact be reconstructed from the parameters of a trained neural network classifier. We propose a novel reconstruction scheme that stems from recent theoretical results about the implicit bias in training neural networks with gradient-based methods. To the best of our knowledge, our results are the first to show that reconstructing a large portion of the actual training samples from a trained neural network classifier is generally possible. This has negative implications on privacy, as it can be used as an attack for revealing sensitive training data. We demonstrate our method for binary MLP classifiers on a few standard computer vision datasets.
更多查看译文
关键词
implicit bias,dataset reconstruction,privacy attacks
AI 理解论文
溯源树
样例
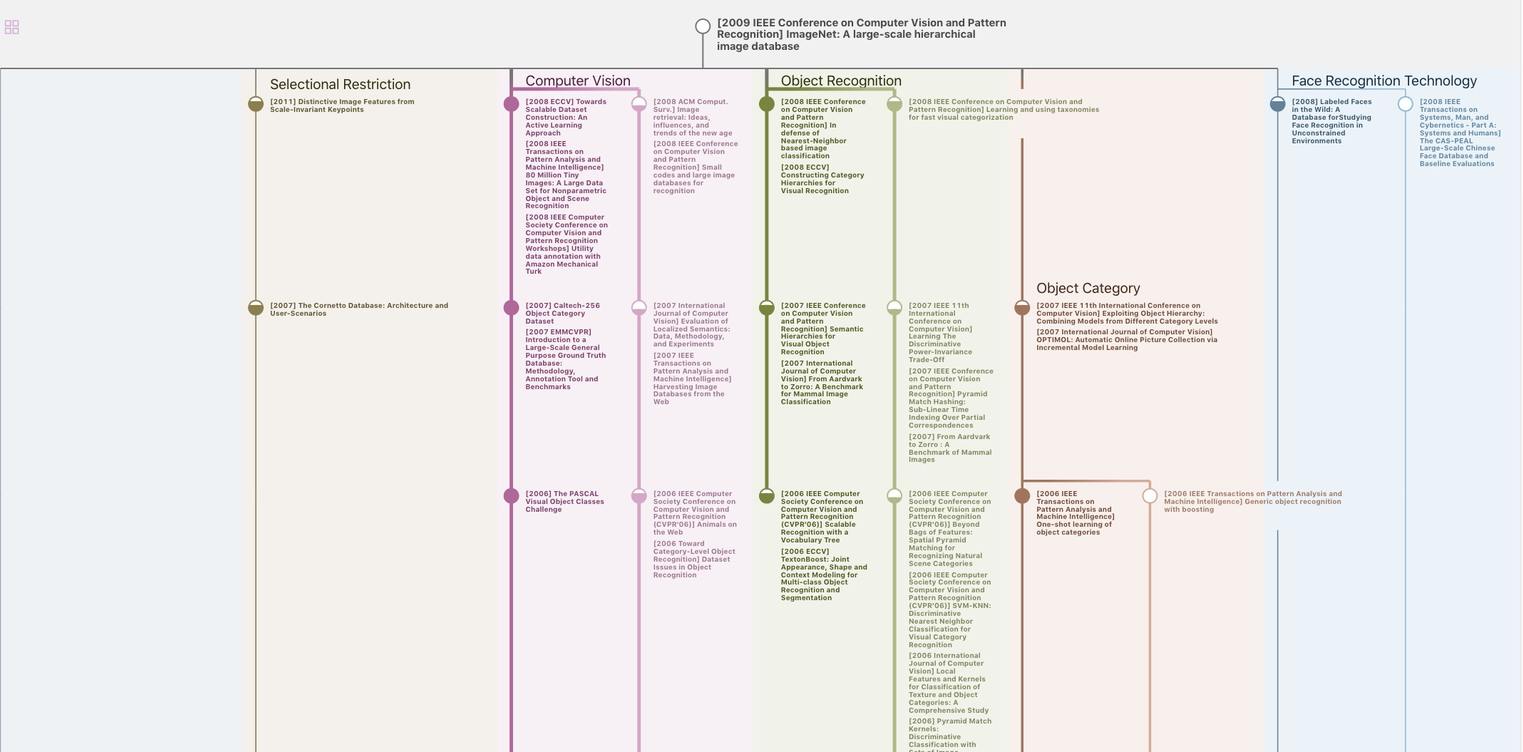
生成溯源树,研究论文发展脉络
Chat Paper
正在生成论文摘要