TriHorn-Net: A Model for Accurate Depth-Based 3D Hand Pose Estimation
arxiv(2022)
摘要
3D hand pose estimation methods have made significant progress recently. However, the estimation accuracy is often far from sufficient for specific real-world applications, and thus there is significant room for improvement. This paper proposes TriHorn-Net, a novel model that uses specific innovations to improve hand pose estimation accuracy on depth images. The first innovation is the decomposition of the 3D hand pose estimation into the estimation of 2D joint locations in the depth image space (UV), and the estimation of their corresponding depths aided by two complementary attention maps. This decomposition prevents depth estimation, which is a more difficult task, from interfering with the UV estimations at both the prediction and feature levels. The second innovation is PixDropout, which is, to the best of our knowledge, the first appearance-based data augmentation method for hand depth images. Experimental results demonstrate that the proposed model outperforms the state-of-the-art methods on three public benchmark datasets. Our implementation is available at https://github.com/mrezaei92/TriHorn-Net.
更多查看译文
关键词
3D hand pose estimation,Depth image,2D joint,Accuracy
AI 理解论文
溯源树
样例
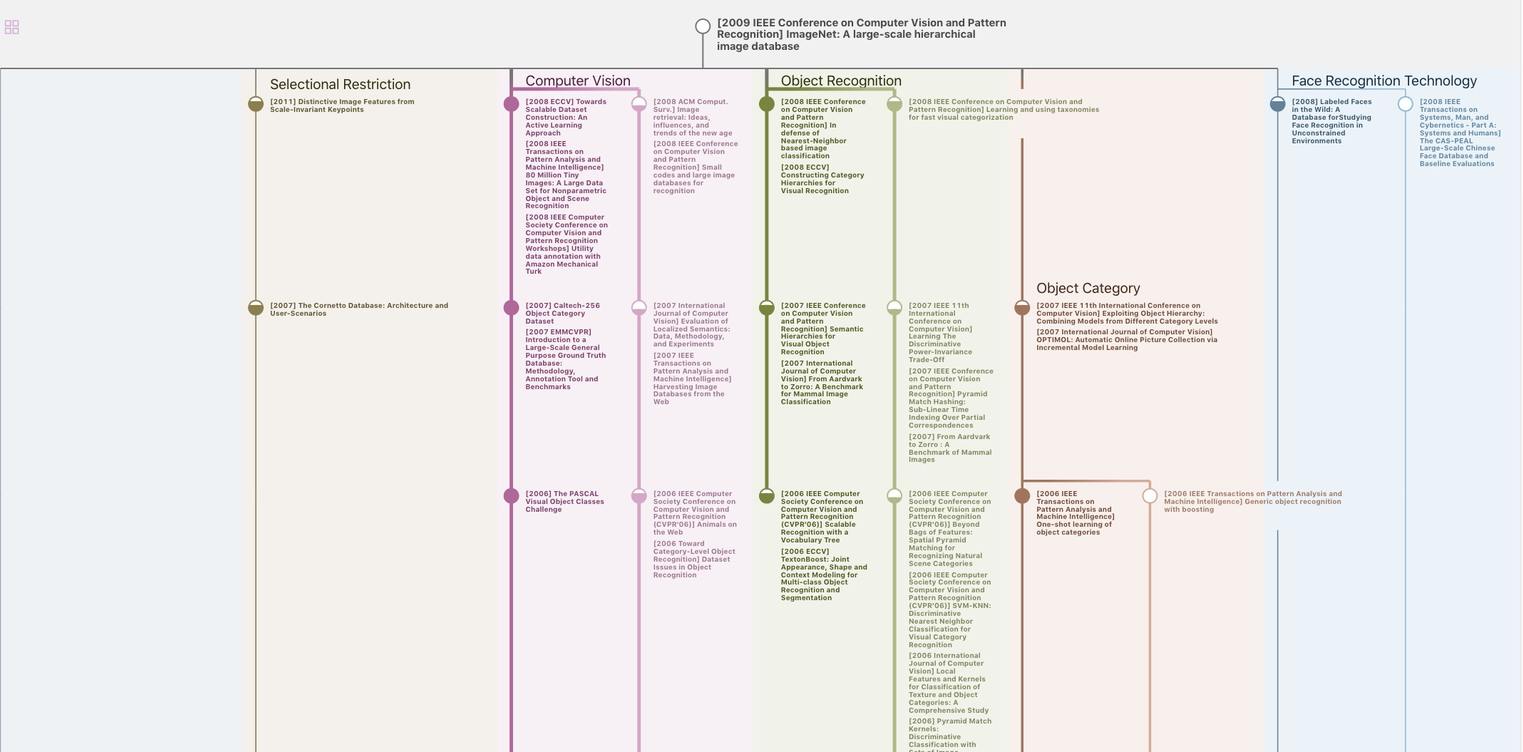
生成溯源树,研究论文发展脉络
Chat Paper
正在生成论文摘要