EPI phase error correction with deep learning (PEC-DL) at 7 T
MAGNETIC RESONANCE IN MEDICINE(2022)
摘要
Purpose The phase mismatch between odd and even echoes in EPI causes Nyquist ghost artifacts. Existing ghost correction methods often suffer from severe residual artifacts and are ineffective with k-space undersampling data. This study proposed a deep learning-based method (PEC-DL) to correct phase errors for DWI at 7 Tesla. Methods The acquired k-space data were divided into 2 independent undersampled datasets according to their readout polarities. Then the proposed PEC-DL network reconstructed 2 ghost-free images using the undersampled data without calibration and navigator data. The network was trained with fully sampled images and applied to two- and fourfold accelerated data. Healthy volunteers and patients with Moyamoya disease were recruited to validate the efficacy of the PEC-DL method. Results The PEC-DL method was capable to mitigate the ghost artifacts in DWI in healthy volunteers as well as patients with Moyamoya disease. The fourfold accelerated results showed much less distortion in the lesions of the Moyamoya patient using high b-value DWI and the corresponding ADC maps. The ghost-to-signal ratios were significantly lower in PEC-DL images compared to conventional linear phase corrections, mini-entropy, and PEC-GRAPPA algorithms. Conclusion The proposed method can effectively eliminate ghost artifacts for full sampled and up to fourfold accelerated EPI data without calibration and navigator data.
更多查看译文
关键词
phase correction, ghost, EPI, deep learning, 7 Tesla
AI 理解论文
溯源树
样例
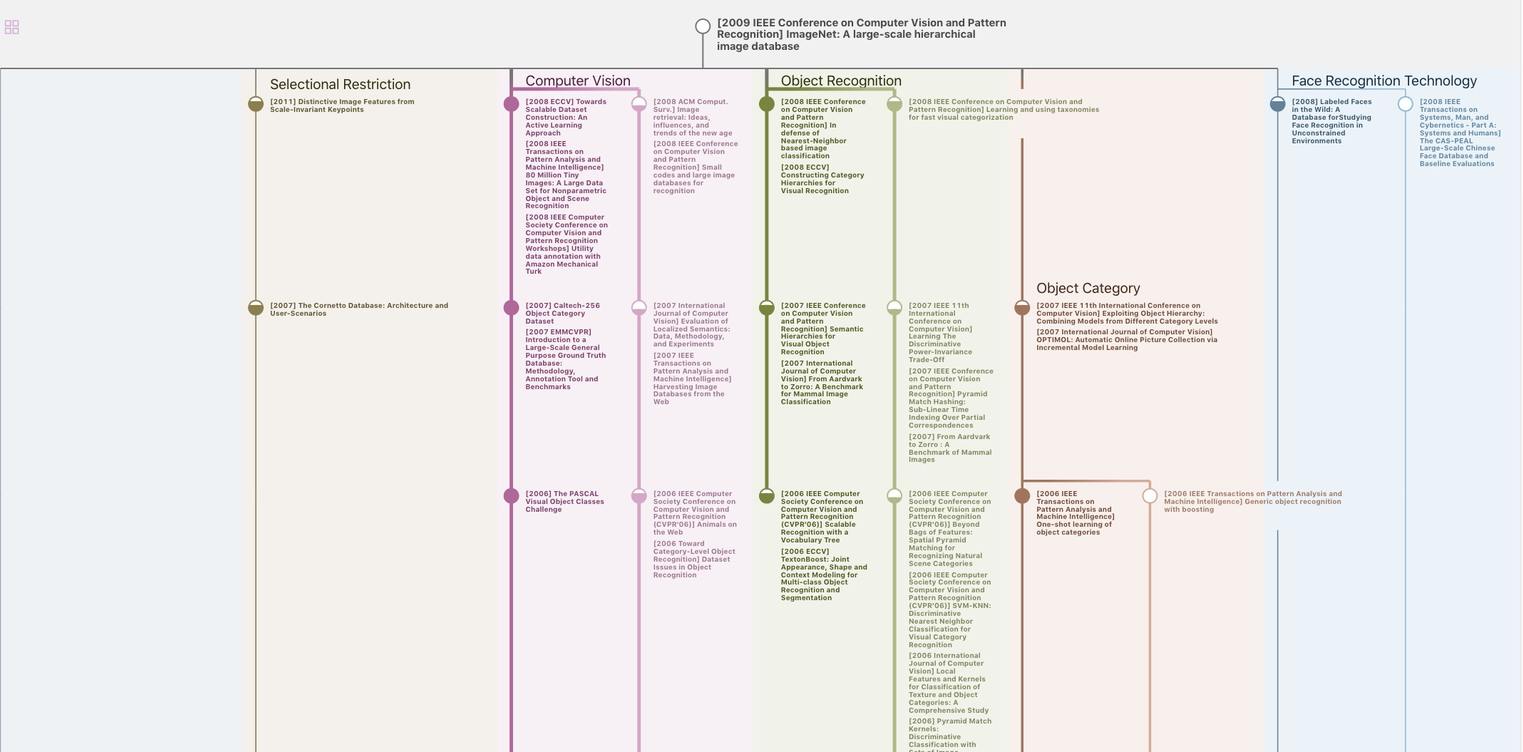
生成溯源树,研究论文发展脉络
Chat Paper
正在生成论文摘要