SmartGD: A GAN-Based Graph Drawing Framework for Diverse Aesthetic Goals.
arxiv(2023)
摘要
While a multitude of studies have been conducted on graph drawing, many existing methods only focus on optimizing a single aesthetic aspect of graph layouts, which can lead to sub-optimal results. There are a few existing methods that have attempted to develop a flexible solution for optimizing different aesthetic aspects measured by different aesthetic criteria. Furthermore, thanks to the significant advance in deep learning techniques, several deep learning-based layout methods were proposed recently. These methods have demonstrated the advantages of deep learning approaches for graph drawing. However, none of these existing methods can be directly applied to optimizing non-differentiable criteria without special accommodation. In this work, we propose a novel Generative Adversarial Network (GAN) based deep learning framework for graph drawing, called, which can optimize different quantitative aesthetic goals, regardless of their differentiability. To demonstrate the effectiveness and efficiency of, we conducted experiments on minimizing stress, minimizing edge crossing, maximizing crossing angle, maximizing shape-based metrics, and a combination of multiple aesthetics. Compared with several popular graph drawing algorithms, the experimental results show that achieves good performance both quantitatively and qualitatively.
更多查看译文
关键词
Deep Learning for Visualization,Graph Visualization,Generative Adversarial Networks
AI 理解论文
溯源树
样例
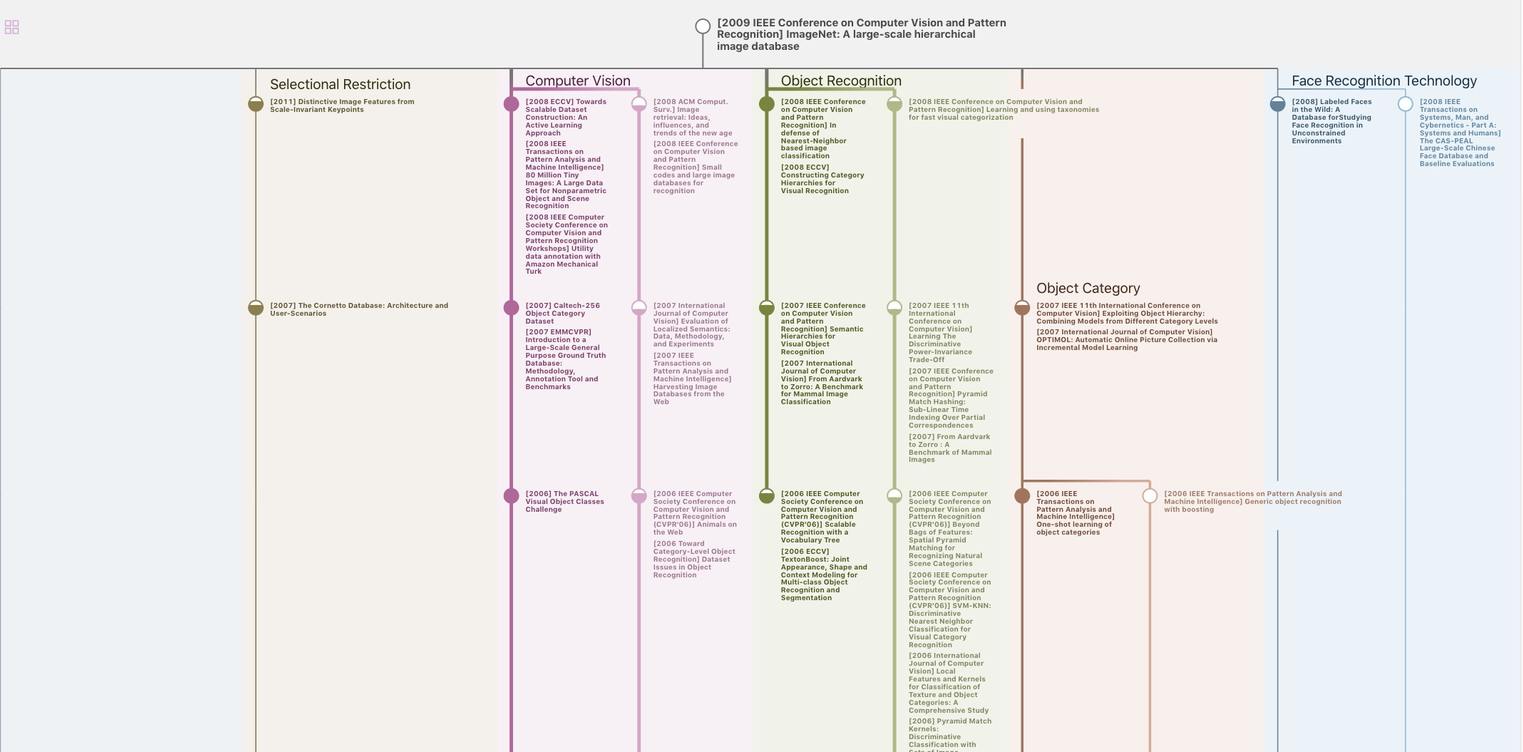
生成溯源树,研究论文发展脉络
Chat Paper
正在生成论文摘要