Exploratory Decision Trees to Predict Obesity Intervention Response among Hispanic American Youth.
Childhood obesity (Print)(2023)
摘要
Individual variability in weight-related outcomes from obesity intervention is widely acknowledged, yet infrequently addressed. This study takes a first step to address individual variation by determining characteristics that distinguish responsive (improvements in BMI) from unresponsive individuals. Classification regression tree (CRT) analysis grouped 185 low-income, Hispanic American middle school students who received a school-based obesity intervention. Predictors included baseline age, gender, standardized BMI, health-related quality of life (PedsQL), minutes of moderate-vigorous physical activity (MVPA; accelerometry), energy consumption, and dietary quality (Block Kids 2004 Food Frequency Questionnaire). Response regarding weight-related outcomes to the intervention was defined according to the American Academy of Pediatrics (AAP) guidelines. Six trees were produced, one for 3- and one for 6-month outcomes among all participants, participants with healthy weight status, and participants with overweight/obesity at baseline. The AAP criteria for response were met by 57.3% and 35.1% of participants at 3 and 6 months, respectively. CRT produced six unique trees. Notably, minutes of MVPA appeared twice (the first time at the top of the tree) in most 3-month models. In addition, response at 3 months consistently appeared as the first variable in all the 6-month models. Overall, the number of distinct pathways and the repeated appearance of the same variable within a pathway illustrate the complex, interactive nature of factors predicting an intervention response. Initially unresponsive individuals were unlikely to respond later in the intervention. More complex modeling is needed to better understand how to best predict who will be responsive to interventions.
更多查看译文
关键词
Hispanic,adolescent,classification regression tree analysis,individual variation,school based
AI 理解论文
溯源树
样例
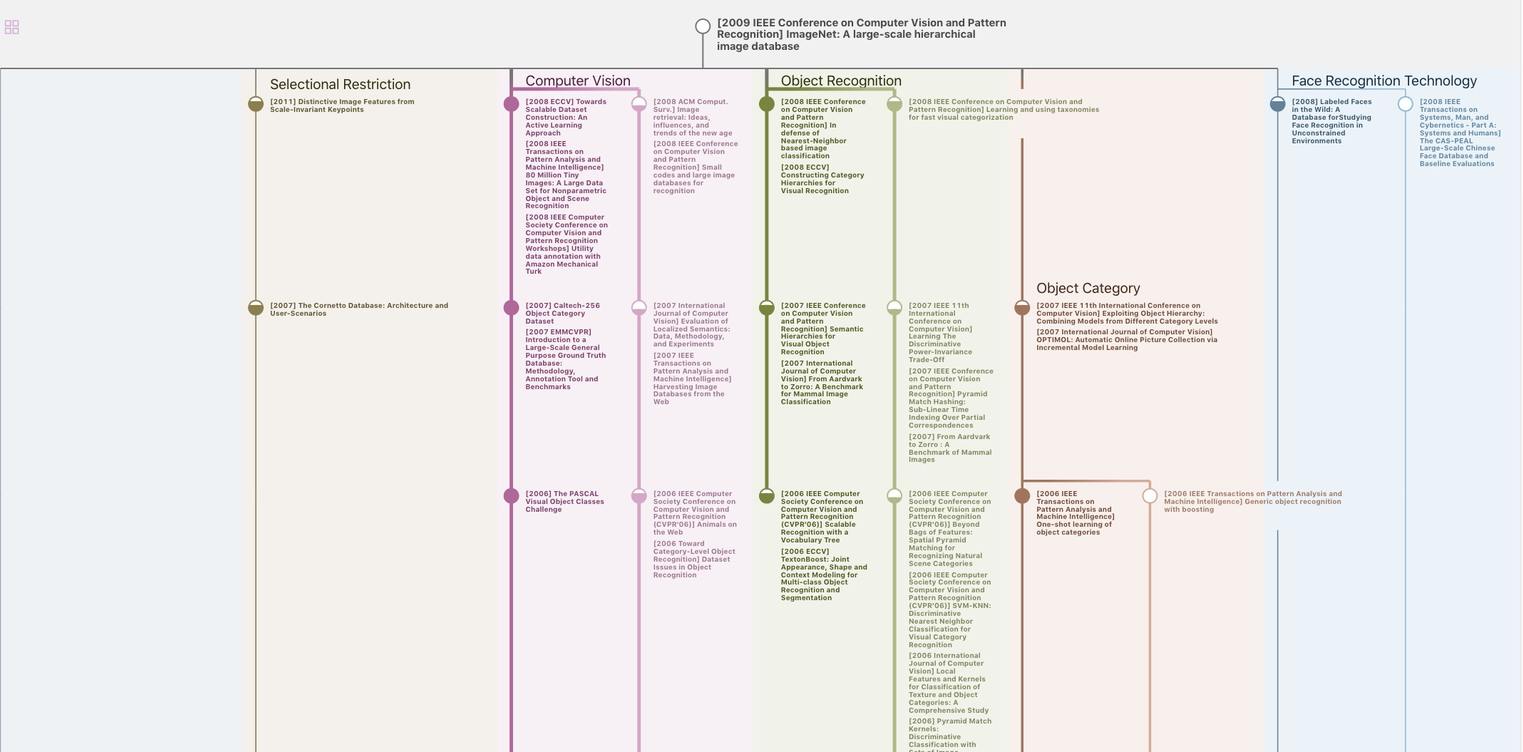
生成溯源树,研究论文发展脉络
Chat Paper
正在生成论文摘要