Predicting Microbe-Disease Association Based on Heterogeneous Network and Global Graph Feature Learning
CHINESE JOURNAL OF ELECTRONICS(2022)
摘要
Numerous microbes inhabit human body, making a vast difference in human health. Hence, discovering associations between microbes and diseases is beneficial to disease prevention and treatment. In this study, we develop a prediction method by learning global graph feature on the heterogeneous network (called HNGFL). Firstly, a heterogeneous network is integrated by known microbe-disease associations and multiple similarities. Based on microbe Gaussian interaction profile (GIP) kernel similarity, we consider different effects of these microbes on organs in the human body to further improve microbe similarity. For disease similarity network, we combine GIP kernel similarity, disease semantic similarity and disease-symptom similarity. And then, an embedding algorithm called GraRep is used to learn global structural information for this network. According to vector feature of every node, we utilize support vector machine classifier to calculate the score for each microbe-disease pair. HNGFL achieves a reliable performance in cross validation, outperforming the compared methods. In addition, we carry out case studies of three diseases. Results show that HNGFL can be considered as a reliable method for microbe-disease association prediction.
更多查看译文
关键词
Microbe-disease association, Heterogeneous network, Global graph feature learning, GraRep
AI 理解论文
溯源树
样例
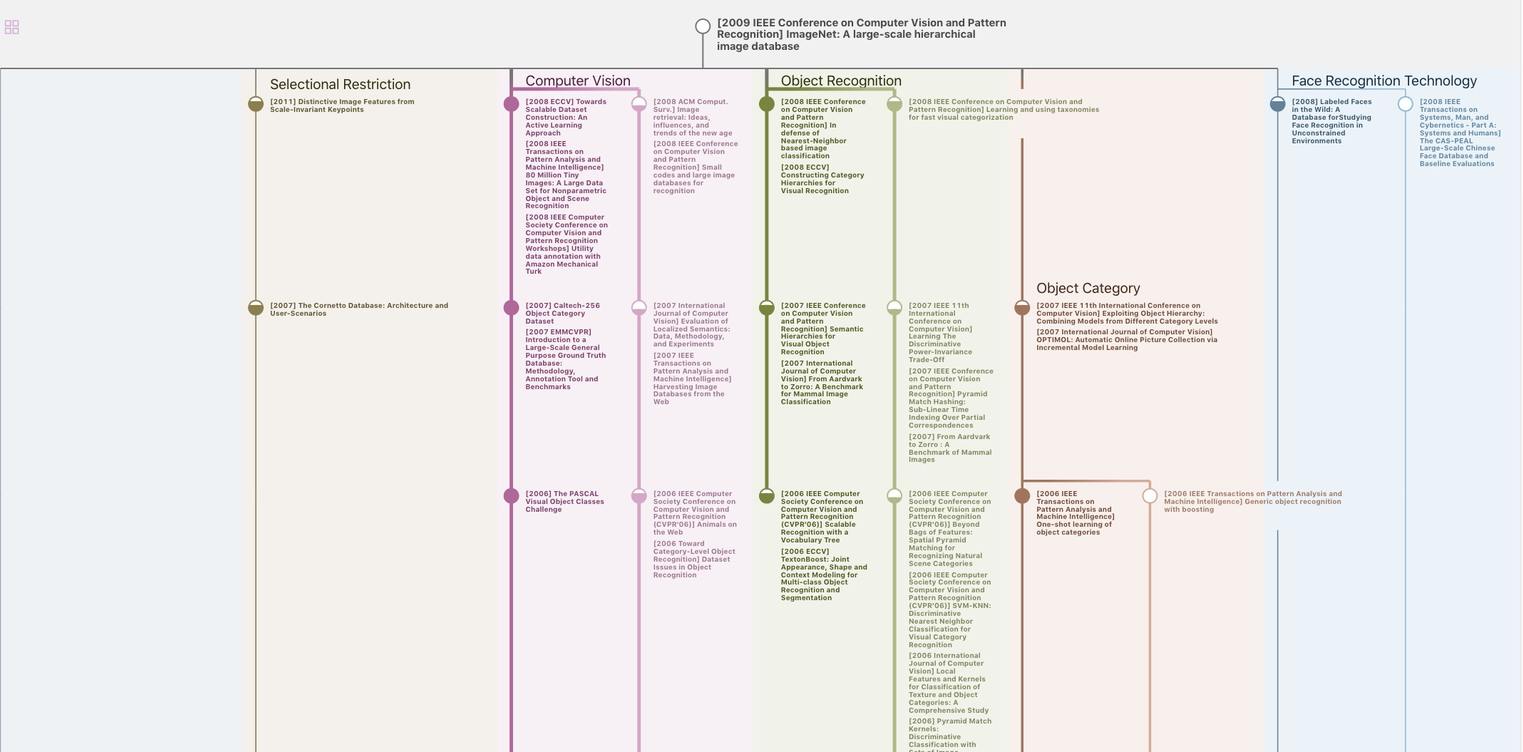
生成溯源树,研究论文发展脉络
Chat Paper
正在生成论文摘要