Knowledge Graph Construction and Its Application in Automatic Radiology Report Generation from Radiologist's Dictation
arxiv(2022)
摘要
Conventionally, the radiologist prepares the diagnosis notes and shares them with the transcriptionist. Then the transcriptionist prepares a preliminary formatted report referring to the notes, and finally, the radiologist reviews the report, corrects the errors, and signs off. This workflow causes significant delays and errors in the report. In current research work, we focus on applications of NLP techniques like Information Extraction (IE) and domain-specific Knowledge Graph (KG) to automatically generate radiology reports from radiologist's dictation. This paper focuses on KG construction for each organ by extracting information from an existing large corpus of free-text radiology reports. We develop an information extraction pipeline that combines rule-based, pattern-based, and dictionary-based techniques with lexical-semantic features to extract entities and relations. Missing information in short dictation can be accessed from the KGs to generate pathological descriptions and hence the radiology report. Generated pathological descriptions evaluated using semantic similarity metrics, which shows 97% similarity with gold standard pathological descriptions. Also, our analysis shows that our IE module is performing better than the OpenIE tool for the radiology domain. Furthermore, we include a manual qualitative analysis from radiologists, which shows that 80-85% of the generated reports are correctly written, and the remaining are partially correct.
更多查看译文
关键词
automatic radiology
AI 理解论文
溯源树
样例
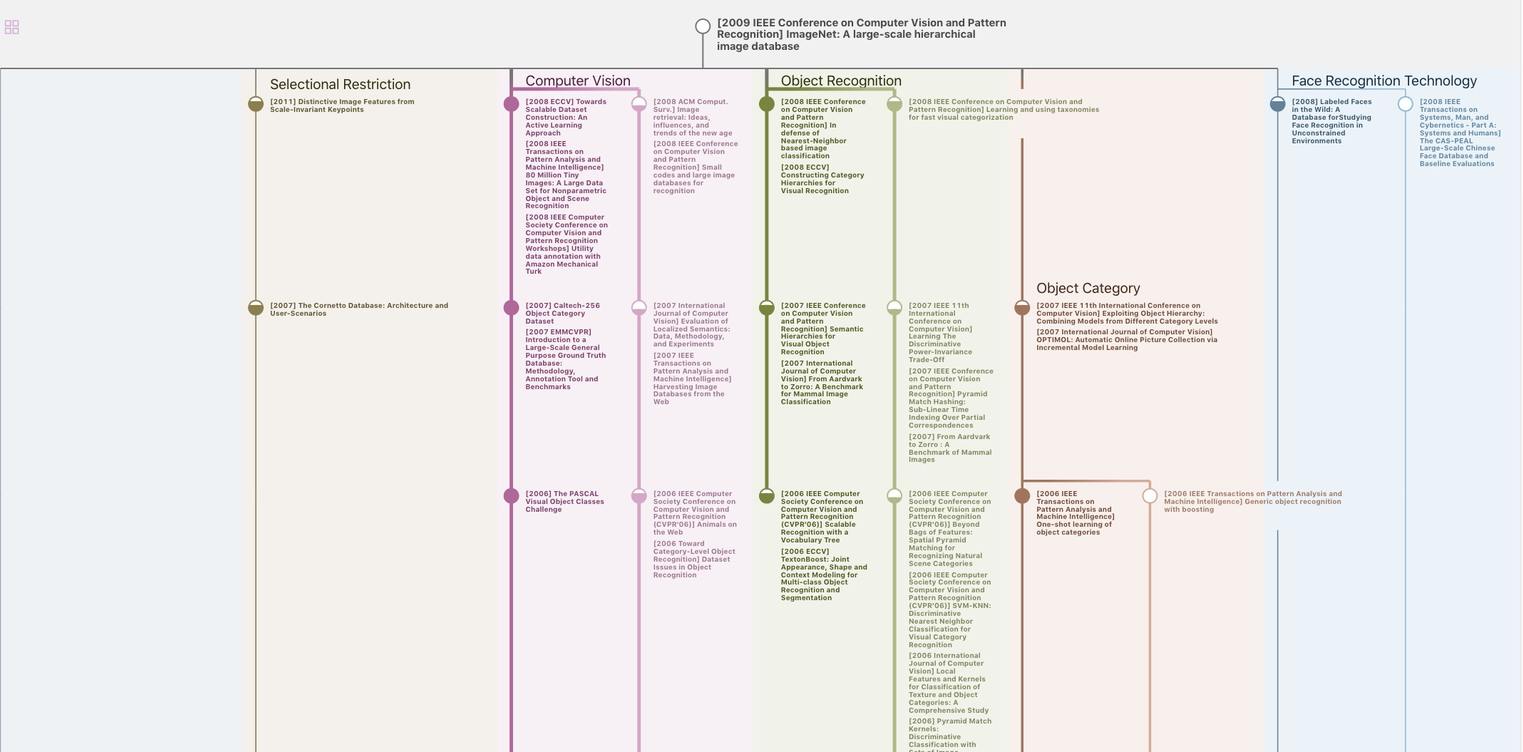
生成溯源树,研究论文发展脉络
Chat Paper
正在生成论文摘要