Analysis of Randomization Effects on Sim2Real Transfer in Reinforcement Learning for Robotic Manipulation Tasks.
IEEE/RJS International Conference on Intelligent RObots and Systems (IROS)(2022)
摘要
Randomization is currently a widely used approach in Sim2Real transfer for data-driven learning algorithms in robotics. Still, most Sim2Real studies report results for a specific randomization technique and often on a highly customized robotic system, making it difficult to evaluate different randomization approaches systematically. To address this problem, we define an easy-to-reproduce experimental setup for a robotic reach-and-balance manipulator task, which can serve as a benchmark for comparison. We compare four randomization strategies with three randomized parameters both in simulation and on a real robot. Our results show that more randomization helps in Sim2Real transfer, yet it can also harm the ability of the algorithm to find a good policy in simulation. Fully randomized simulations and fine-tuning show differentiated results and translate better to the real robot than the other approaches tested.
更多查看译文
关键词
reinforcement learning,robotic manipulation tasks,sim2real transfer,randomization effects
AI 理解论文
溯源树
样例
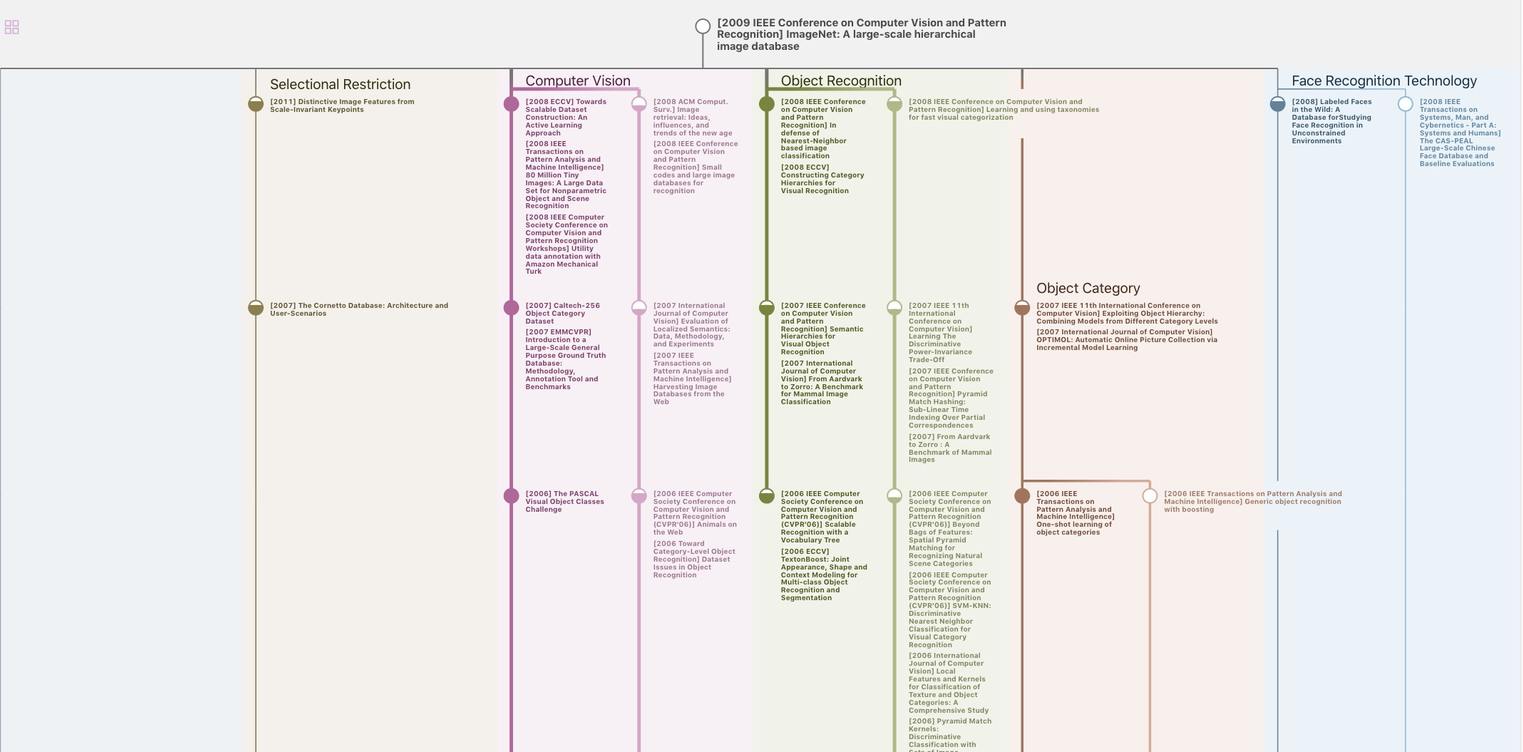
生成溯源树,研究论文发展脉络
Chat Paper
正在生成论文摘要