Local Distance Preserving Auto-encoders using Continuous k-Nearest Neighbours Graphs
arxiv(2022)
摘要
Auto-encoder models that preserve similarities in the data are a popular tool in representation learning. In this paper we introduce several auto-encoder models that preserve local distances when mapping from the data space to the latent space. We use a local distance preserving loss that is based on the continuous k-nearest neighbours graph which is known to capture topological features at all scales simultaneously. To improve training performance, we formulate learning as a constraint optimisation problem with local distance preservation as the main objective and reconstruction accuracy as a constraint. We generalise this approach to hierarchical variational auto-encoders thus learning generative models with geometrically consistent latent and data spaces. Our method provides state-of-the-art performance across several standard datasets and evaluation metrics.
更多查看译文
关键词
manifold learning,representational learning,generative models
AI 理解论文
溯源树
样例
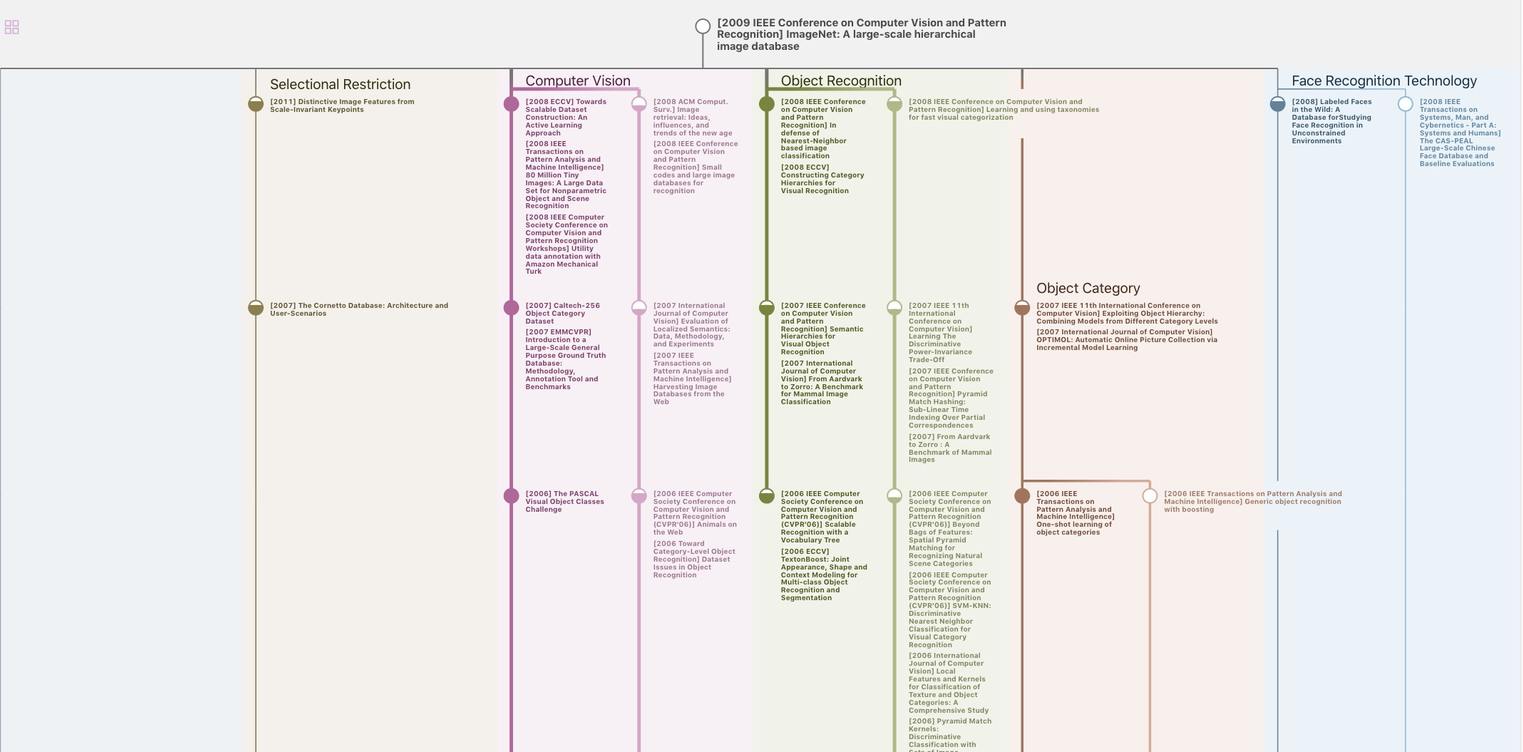
生成溯源树,研究论文发展脉络
Chat Paper
正在生成论文摘要