COLD Fusion: Calibrated and Ordinal Latent Distribution Fusion for Uncertainty-Aware Multimodal Emotion Recognition
IEEE TRANSACTIONS ON PATTERN ANALYSIS AND MACHINE INTELLIGENCE(2024)
摘要
Automatically recognising apparent emotions from face and voice is hard, in part because of various sources of uncertainty, including in the input data and the labels used in a machine learning framework. This paper introduces an uncertainty-aware multimodal fusion approach that quantifies modality-wise aleatoric or data uncertainty towards emotion prediction. We propose a novel fusion framework, in which latent distributions over unimodal temporal context are learned by constraining their variance. These variance constraints, Calibration and Ordinal Ranking, are designed such that the variance estimated for a modality can represent how informative the temporal context of that modality is w.r.t. emotion recognition. When well-calibrated, modality-wise uncertainty scores indicate how much their corresponding predictions are likely to differ from the ground truth labels. Well-ranked uncertainty scores allow the ordinal ranking of different frames across different modalities. To jointly impose both these constraints, we propose a softmax distributional matching loss. Our evaluation on AVEC 2019 CES, CMU-MOSEI, and IEMOCAP datasets shows that the proposed multimodal fusion method not only improves the generalisation performance of emotion recognition models and their predictive uncertainty estimates, but also makes the models robust to novel noise patterns encountered at test time.
更多查看译文
关键词
Dimensional affect recognition,multimodal fusion,uncertainty modeling,categorical emotion recognition
AI 理解论文
溯源树
样例
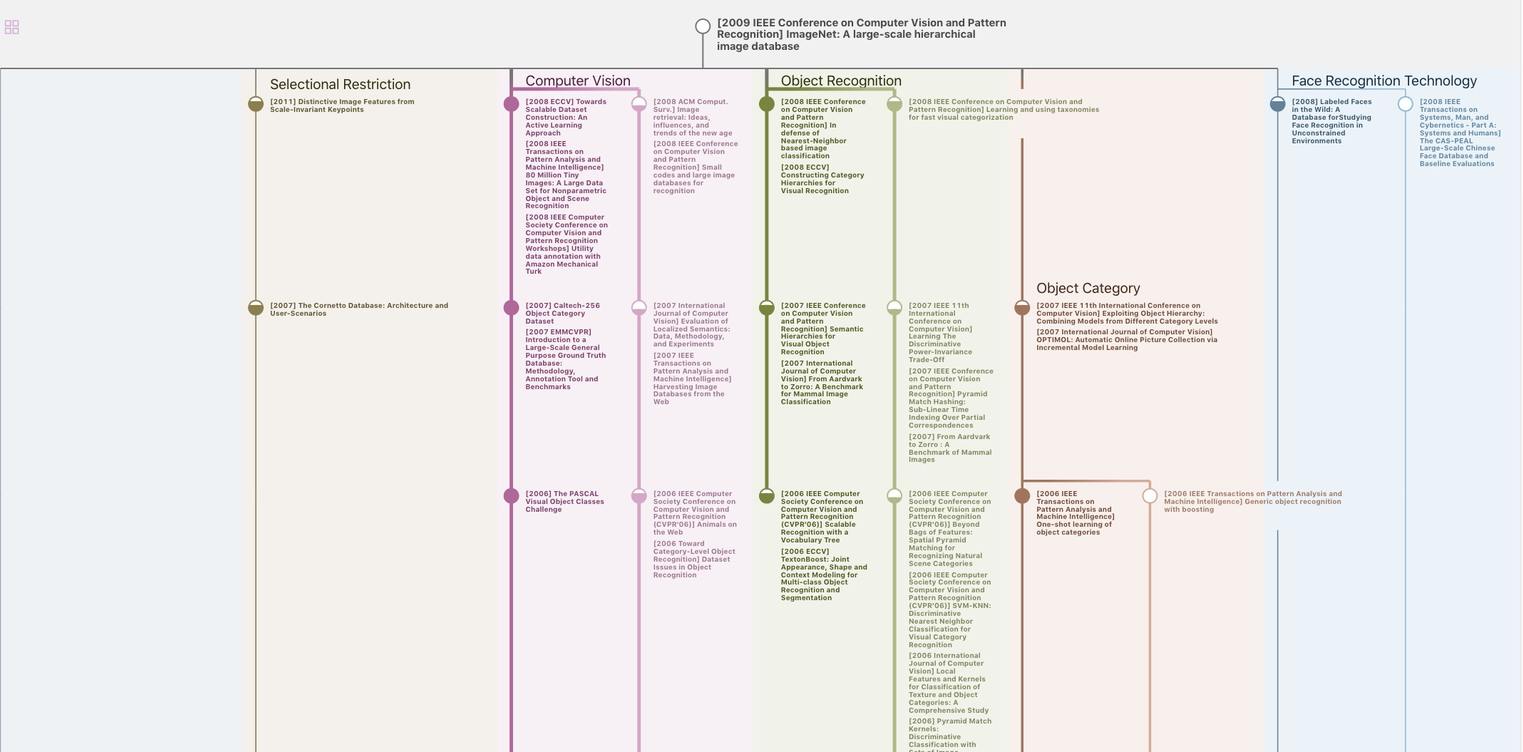
生成溯源树,研究论文发展脉络
Chat Paper
正在生成论文摘要