Anchor-Changing Regularized Natural Policy Gradient for Multi-Objective Reinforcement Learning
NeurIPS 2022(2022)
摘要
We study policy optimization for Markov decision processes (MDPs) with multiple reward value functions, which are to be jointly optimized according to given criteria such as proportional fairness (smooth concave scalarization), hard constraints (constrained MDP), and max-min trade-off. We propose an Anchor-changing Regularized Natural Policy Gradient (ARNPG) framework, which can systematically incorporate ideas from well-performing first-order methods into the design of policy optimization algorithms for multi-objective MDP problems. Theoretically, the designed algorithms based on the ARNPG framework achieve $\tilde{O}(1/T)$ global convergence with exact gradients. Empirically, the ARNPG-guided algorithms also demonstrate superior performance compared to some existing policy gradient-based approaches in both exact gradients and sample-based scenarios.
更多查看译文
关键词
Multi-Objective Markov Decision Process,Constrained Markov Decision Process,Policy Optimization
AI 理解论文
溯源树
样例
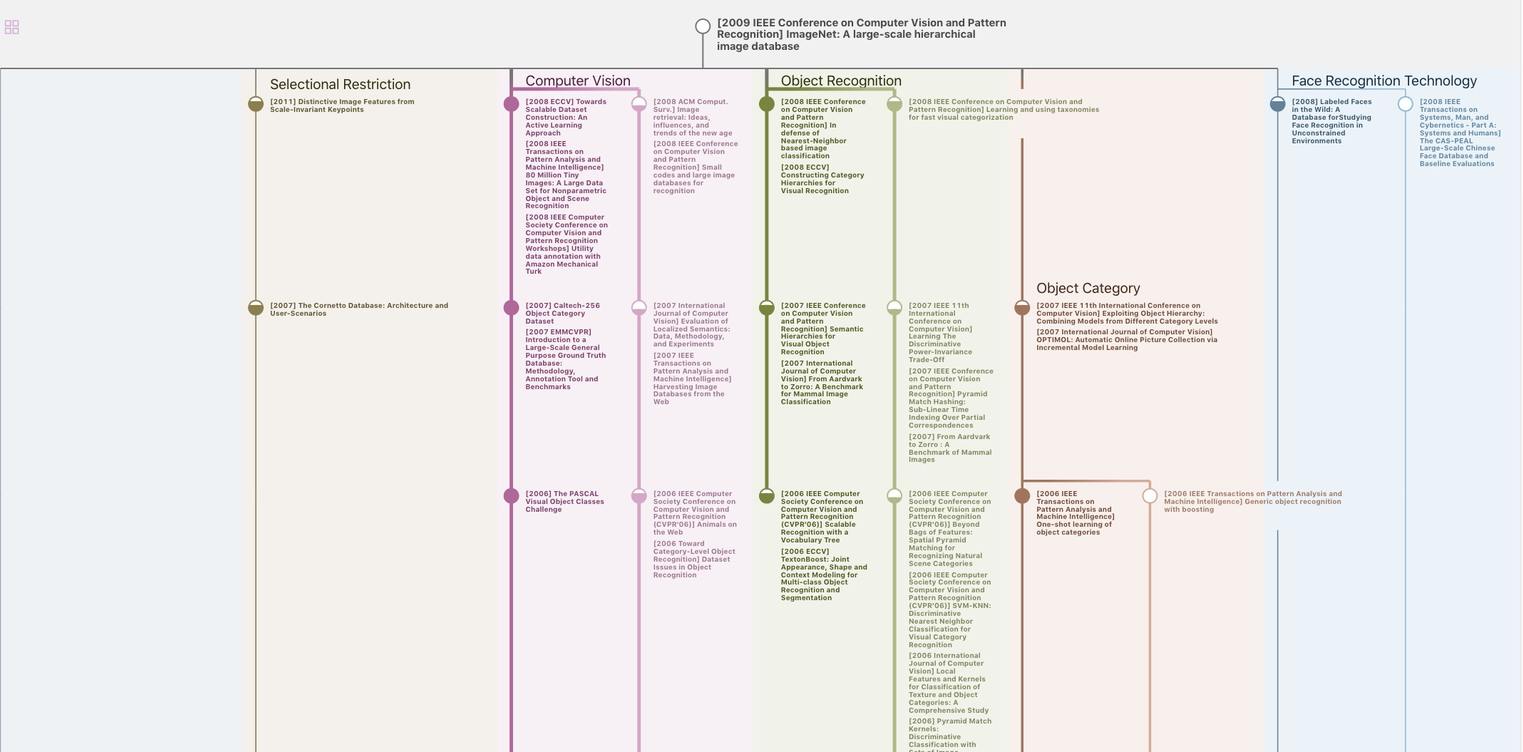
生成溯源树,研究论文发展脉络
Chat Paper
正在生成论文摘要