Graph-in-Graph Network for Automatic Gene Ontology Description Generation
KDD '22: Proceedings of the 28th ACM SIGKDD Conference on Knowledge Discovery and Data Mining(2022)
摘要
Gene Ontology (GO) is the primary gene function knowledge base that enables computational tasks in biomedicine. The basic element of GO is a term, which includes a set of genes with the same function. Existing research efforts of GO mainly focus on predicting gene term associations. Other tasks, such as generating descriptions of new terms, are rarely pursued. In this paper, we propose a novel task: GO term description generation. This task aims to automatically generate a sentence that describes the function of a GO term belonging to one of the three categories, i.e., molecular function, biological process, and cellular component. To address this task, we propose a Graph-in-Graph network that can efficiently leverage the structural information of GO. The proposed network introduces a two-layer graph: the first layer is a graph of GO terms where each node is also a graph (gene graph). Such a Graph-in-Graph network can derive the biological functions of GO terms and generate proper descriptions. To validate the effectiveness of the proposed network, we build three large-scale benchmark datasets. By incorporating the proposed Graph-in-Graph network, the performances of seven different sequence-to-sequence models can be substantially boosted across all evaluation metrics, with up to 34.7%, 14.5%, and 39.1% relative improvements in BLEU, ROUGE-L, and METEOR, respectively.
更多查看译文
关键词
gene,graph-in-graph
AI 理解论文
溯源树
样例
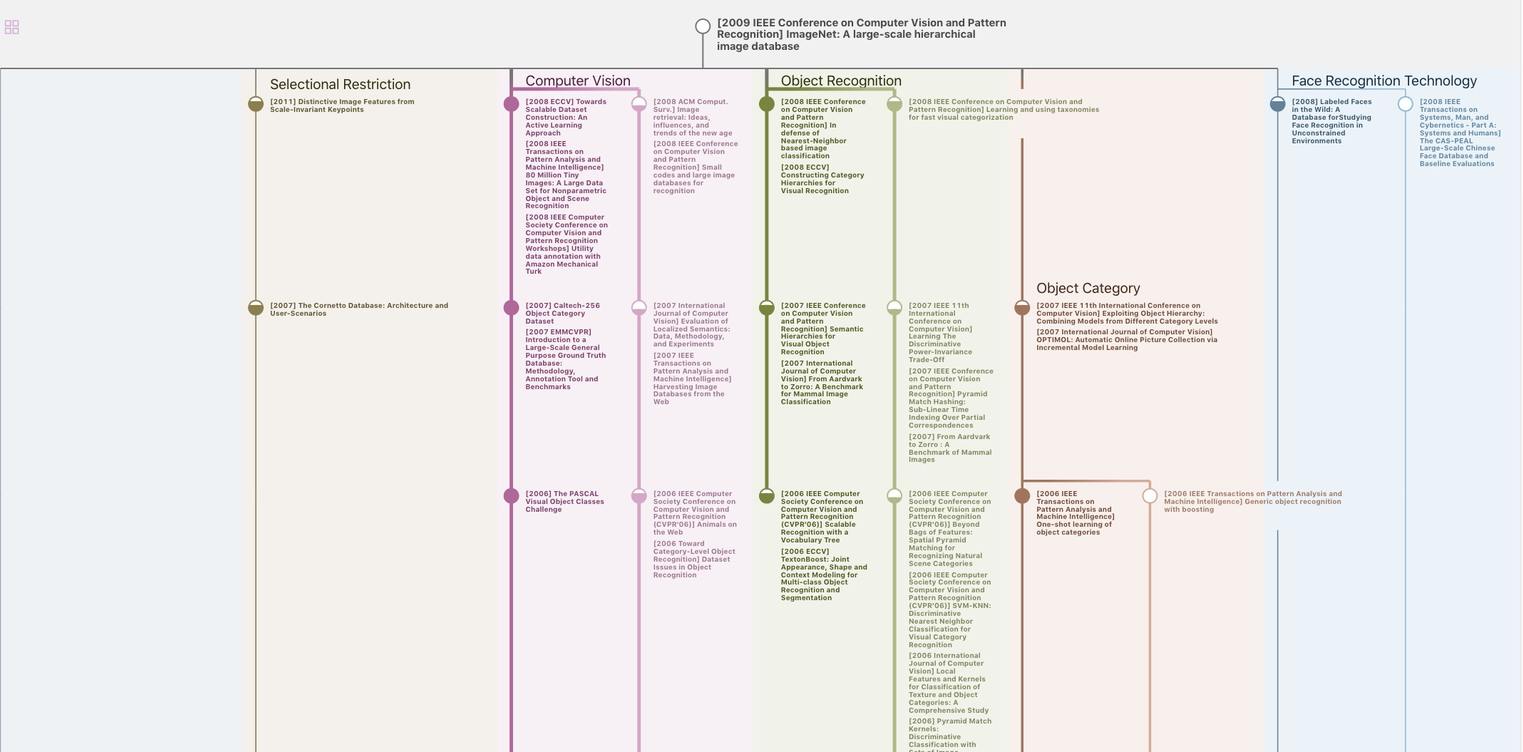
生成溯源树,研究论文发展脉络
Chat Paper
正在生成论文摘要