Learning the Space of Deep Models.
ICPR(2022)
摘要
Embedding of large but redundant data, such as images or text, in a hierarchy of lower-dimensional spaces is one of the key features of representation learning approaches, which nowadays provide state-of-the-art solutions to problems once believed hard or impossible to solve. In this work, in a plot twist with a strong meta aftertaste, we show how trained deep models are as redundant as the data they are optimized to process, and how it is therefore possible to use deep learning models to embed deep learning models. In particular, we show that it is possible to use representation learning to learn a fixed-size, low-dimensional embedding space of trained deep models and that such space can be explored by interpolation or optimization to attain ready-to-use models. We find that it is possible to learn an embedding space of multiple instances of the same architecture and of multiple architectures. We address image classification and neural representation of signals, showing how our embedding space can be learnt so as to capture the notions of performance and 3D shape, respectively. In the Multi-Architecture setting we also show how an embedding trained only on a subset of architectures can learn to generate already-trained instances of architectures it never sees instantiated at training time.
更多查看译文
关键词
3D shape capture,data redundancy,deep learning models,deep model training,image classification,interpolation,low-dimensional embedding space,neural signal representation,optimization,performance notion capture,representation learning
AI 理解论文
溯源树
样例
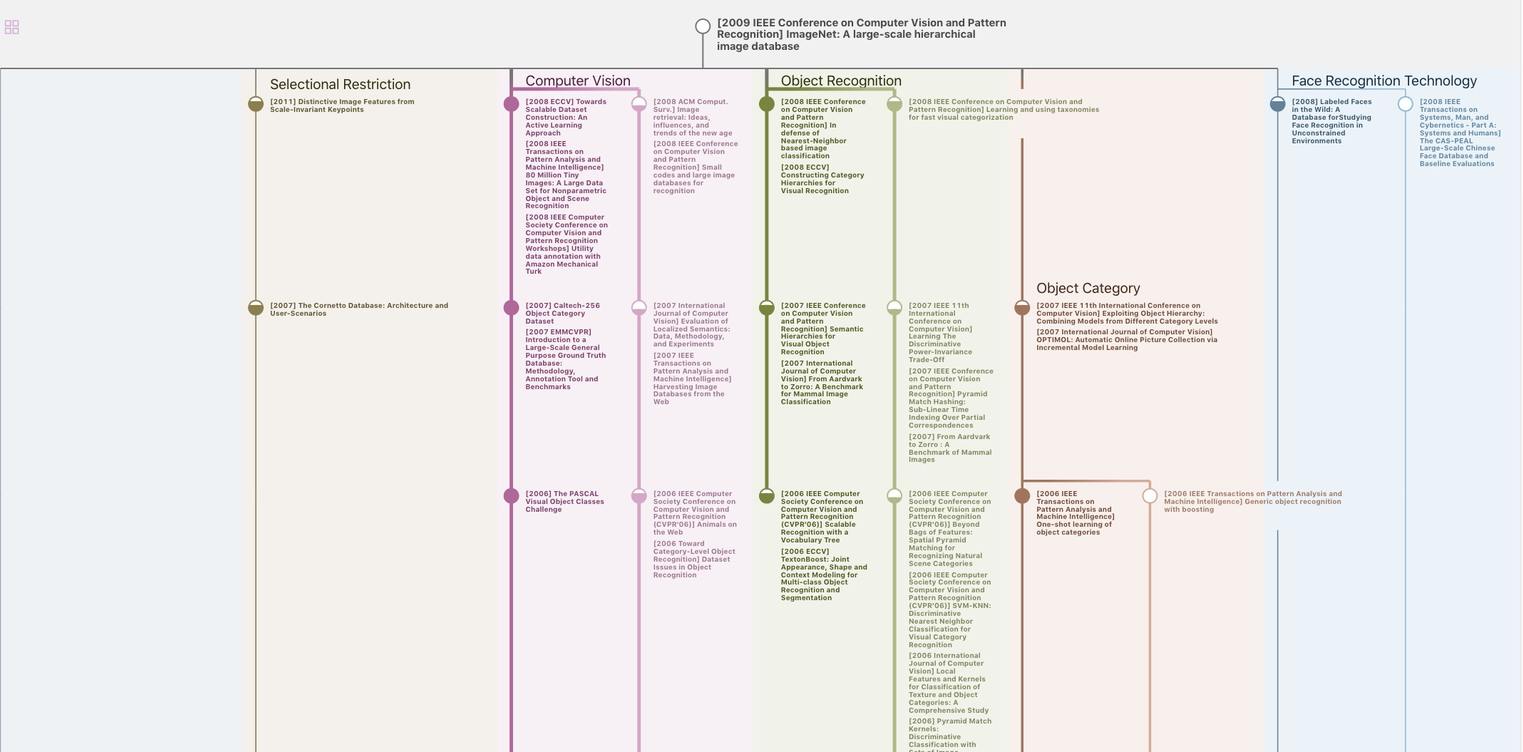
生成溯源树,研究论文发展脉络
Chat Paper
正在生成论文摘要