Feature-aware Diversified Re-ranking with Disentangled Representations for Relevant Recommendation
KDD '22: Proceedings of the 28th ACM SIGKDD Conference on Knowledge Discovery and Data Mining(2022)
摘要
Relevant recommendation is a special recommendation scenario which provides relevant items when users express interests on one target item (e.g., click, like and purchase). Besides considering the relevance between recommendations and trigger item, the recommendations should also be diversified to avoid information cocoons. However, existing diversified recommendation methods mainly focus on item-level diversity which is insufficient when the recommended items are all relevant to the target item. Moreover, redundant or noisy item features might affect the performance of simple feature-aware recommendation approaches. Faced with these issues, we propose a Feature Disentanglement Self-Balancing Re-ranking framework (FDSB) to capture feature- aware diversity. The framework consists of two major modules, namely disentangled attention encoder (DAE) and self-balanced multi-aspect ranker. In DAE, we use multi-head attention to learn disentangled aspects from rich item features. In the ranker, we develop an aspect-specific ranking mechanism that is able to adaptively balance the relevance and diversity for each aspect. In experiments, we conduct offline evaluation on the collected dataset and deploy FDSB on KuaiShou app for online ??/?? test on the function of relevant recommendation. The significant improvements on both recommendation quality and user experience verify the effectiveness of our approach.
更多查看译文
关键词
disentangled representations,feature-aware,re-ranking
AI 理解论文
溯源树
样例
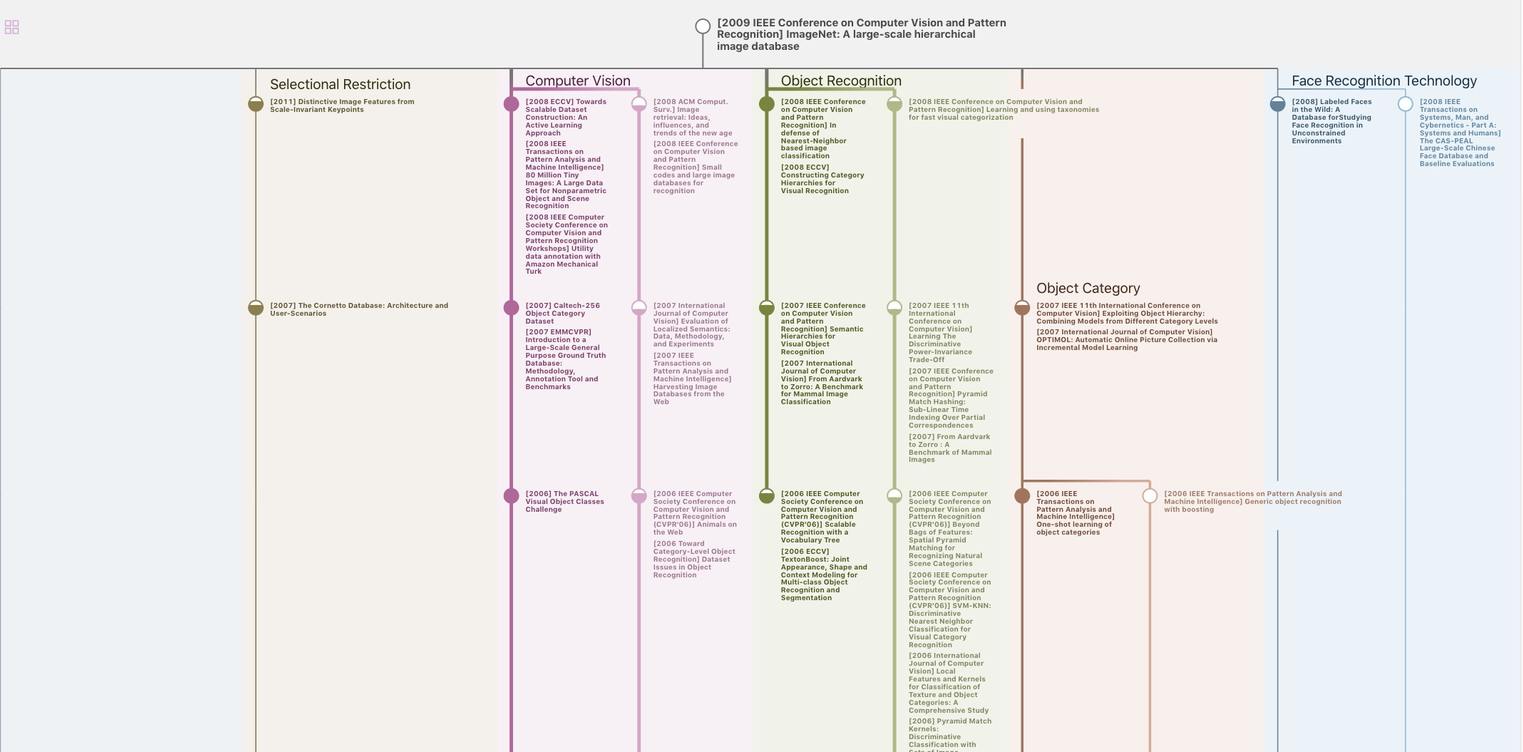
生成溯源树,研究论文发展脉络
Chat Paper
正在生成论文摘要