Efficient Heterogeneous Treatment Effect Estimation With Multiple Experiments and Multiple Outcomes
arxiv(2022)
摘要
Learning heterogeneous treatment effects (HTEs) is an important problem across many fields. Most existing methods consider the setting with a single treatment arm and a single outcome metric. However, in many real world domains, experiments are run consistently - for example, in internet companies, A/B tests are run every day to measure the impacts of potential changes across many different metrics of interest. We show that even if an analyst cares only about the HTEs in one experiment for one metric, precision can be improved greatly by analyzing all of the data together to take advantage of cross-experiment and cross-outcome metric correlations. We formalize this idea in a tensor factorization framework and propose a simple and scalable model which we refer to as the low rank or LR-learner. Experiments in both synthetic and real data suggest that the LR-learner can be much more precise than independent HTE estimation.
更多查看译文
关键词
outcomes,experiments,treatment,multiple
AI 理解论文
溯源树
样例
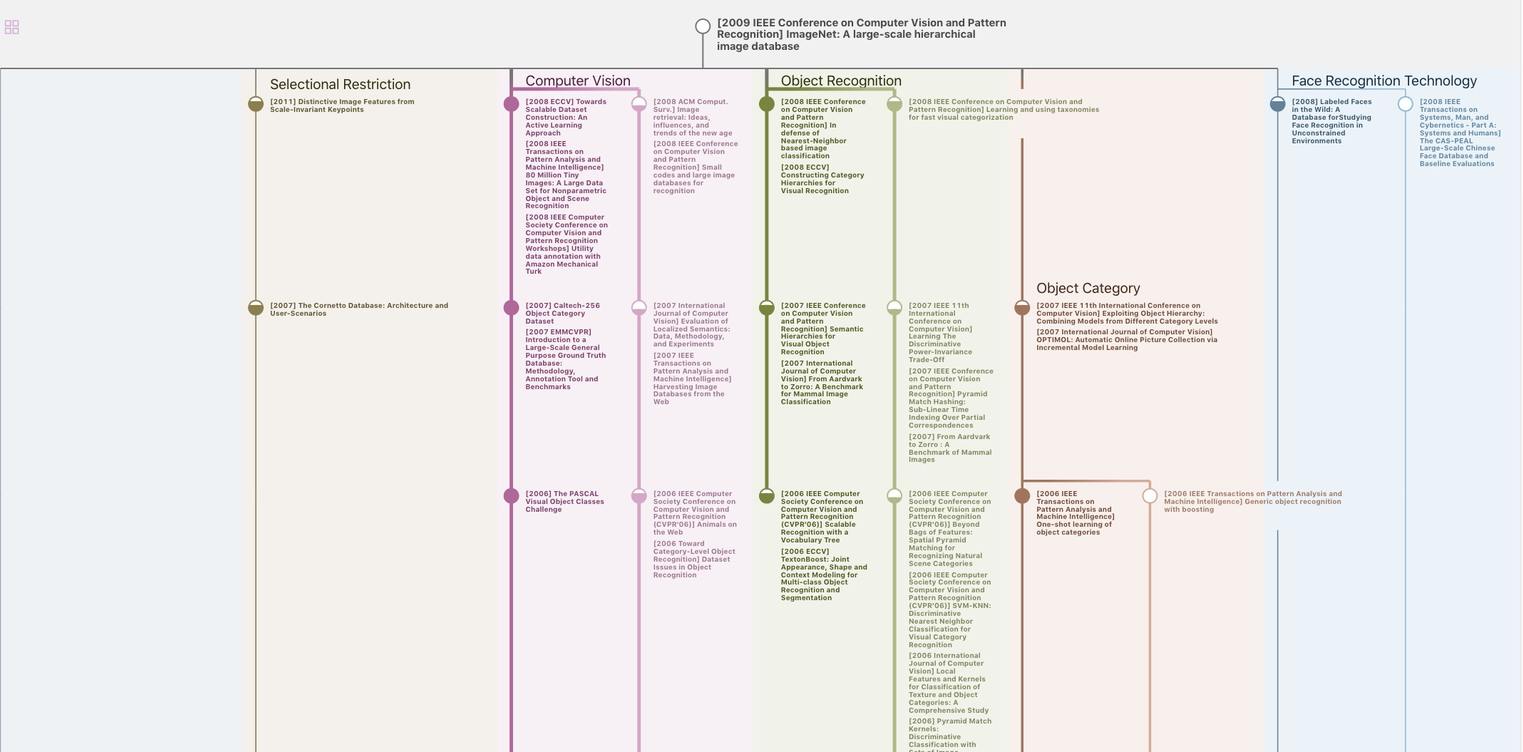
生成溯源树,研究论文发展脉络
Chat Paper
正在生成论文摘要