Learning attention-based representations from multiple patterns for relation prediction in knowledge graphs
Knowledge-Based Systems(2022)
摘要
Knowledge bases, and their representations in the form of knowledge graphs (KGs), are naturally incomplete. Since scientific and industrial applications have extensively adopted them, there is a high demand for solutions that complete their information. Several recent works tackle this challenge by learning embeddings for entities and relations, then employing them to predict new relations among the entities. Despite their aggrandizement, most of those methods focus only on the local neighbors of a relation to learn the embeddings. As a result, they may fail to capture the KGs’ context information by neglecting long-term dependencies and the propagation of entities’ semantics. In this manuscript, we propose ÆMP (Attention-based Embeddings from Multiple Patterns), a novel model for learning contextualized representations by: (i) acquiring entities context information through an attention-enhanced message-passing scheme, which captures the entities local semantics while focusing on different aspects of their neighborhood; and (ii) capturing the semantic context, by leveraging the paths and their relationships between entities. Our empirical findings draw insights into how attention mechanisms can improve entities’ context representation and how combining entities and semantic path contexts improves the general representation of entities and the relation predictions. Experimental results on several large and small knowledge graph benchmarks show that ÆMP either outperforms or competes with state-of-the-art relation prediction methods.
更多查看译文
关键词
Knowledge graphs,Representation learning,Embeddings,Attention mechanism
AI 理解论文
溯源树
样例
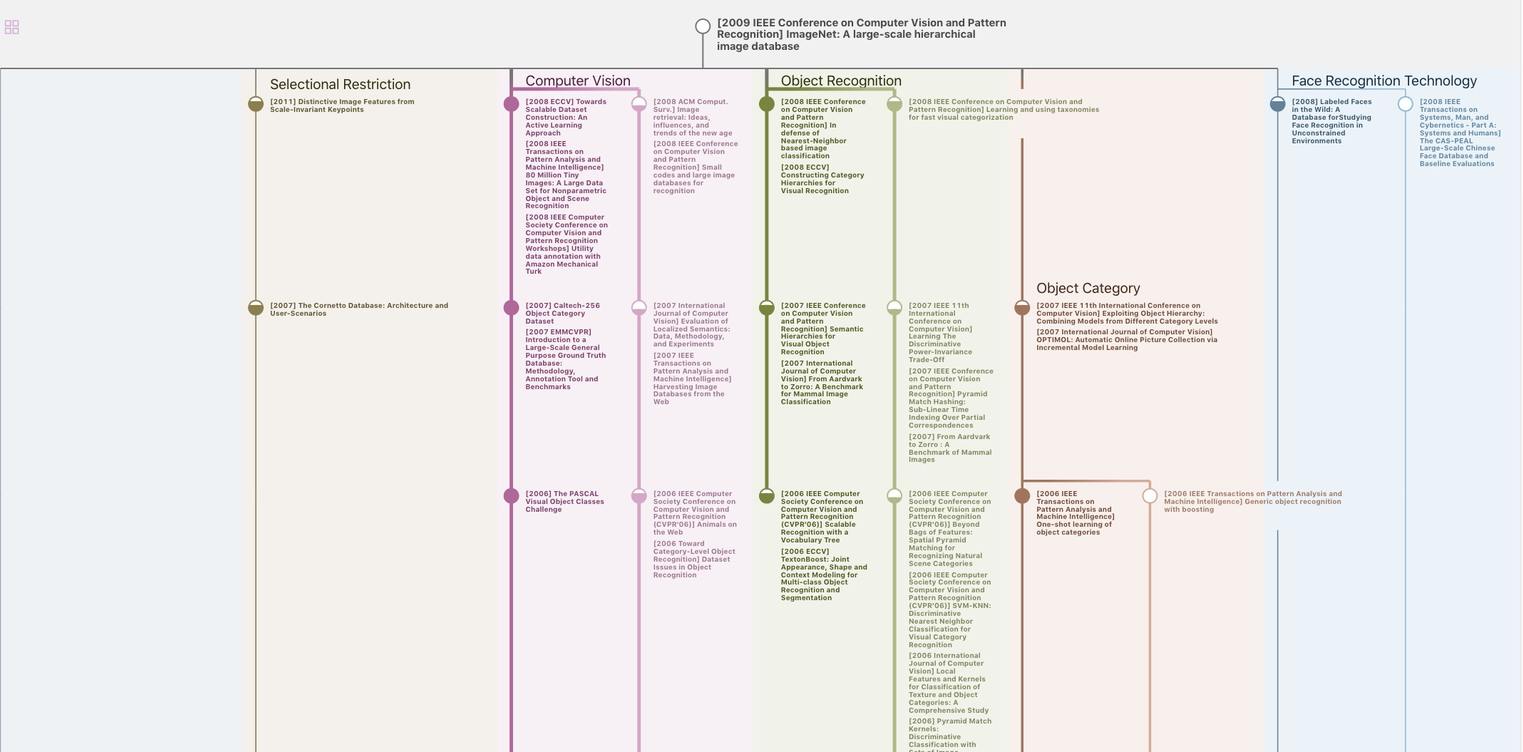
生成溯源树,研究论文发展脉络
Chat Paper
正在生成论文摘要