Machine learning-derived integer-based score and prediction of tertiary hyperparathyroidism among kidney transplant recipients
CLINICAL JOURNAL OF THE AMERICAN SOCIETY OF NEPHROLOGY(2023)
摘要
Background and objectives Tertiary hyperparathyroidism in kidney allograft recipients is associated with boneloss, allograft dysfunction, and cardiovascular mortality. Accurate pretransplant risk prediction of tertiaryhyperparathyroidism may support individualized treatment decisions. We aimed to develop an integer scoresystem that predicts the risk of tertiary hyperparathyroidism using machine learning algorithms. Design, setting, participants, & measurements We used two separate cohorts: a derivation cohort with the dataof kidney allograft recipients (n5669) who underwent kidney transplantation at Severance Hospital, Seoul, Koreabetween January 2009 and December 2015 and a multicenter registry dataset (the Korean Cohort Studyfor Outcome in Patients with Kidney Transplantation) as an external validation cohort (n5542). Tertiaryhyperparathyroidism was defined as post-transplant parathyroidectomy. The derivation cohort wassplit into 75% training set (n5501) and 25% holdout test set (n5168) to develop prediction models andinteger-based score. Results Tertiary hyperparathyroidism requiring parathyroidectomy occurred in 5% and 2% of the derivation andvalidation cohorts, respectively. Three top predictors (dialysis duration, pretransplant intact parathyroidhormone, and serum calcium level measured at the time of admission for kidney transplantation) were identifiedto create an integer score system (dialysis duration, pretransplant serum parathyroid hormone level, andpretransplant calcium level score [DPC]; 0-15 points) to predict tertiary hyperparathyroidism. The median DPCscore was higher in participants with post-transplant parathyroidectomy than in those without (13 versus three inderivation; 13 versus four in external validation;P,0.001 for all). Pretransplant dialysis duration, pretransplantserum parathyroid hormone level, and pretransplant calcium level score predicted post-transplantparathyroidectomy with comparable performance with the best-performing machine learning model in the testset (area under the receiver operating characteristic curve: 0.94 versus 0.92; area under the precision-recall curve:0.52 versus 0.47). Serial measurement of DPC scores ($13 at least two or more times, 3-month interval) during 12months prior to kidney transplantation improved risk classification for post-transplant parathyroidectomycompared with single-time measurement (net reclassification improvement, 0.28; 95% confidence interval, 0.02 to0.54;P50.03). Conclusions A simple integer-based score predicted the risk of tertiary hyperparathyroidism in kidney allograftrecipients, with improved classification by serial measurement compared with single-time measurement.
更多查看译文
关键词
transplantation, hyperparathyroidism, artificial intelligence, calcium, parathyroid hormone, machine learning, transplant recipients
AI 理解论文
溯源树
样例
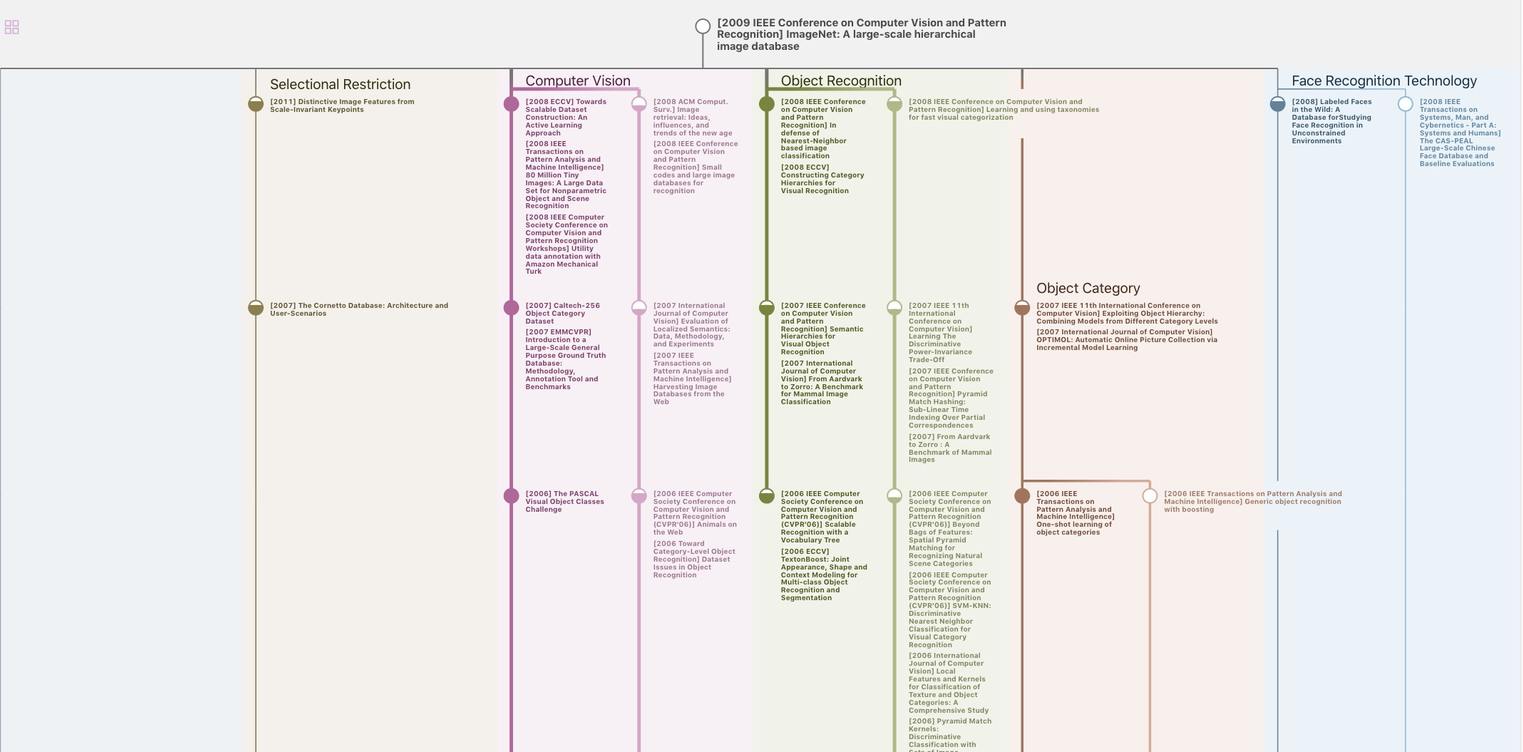
生成溯源树,研究论文发展脉络
Chat Paper
正在生成论文摘要