Multi-parametric MRI-based radiomics signature for preoperative prediction of Ki-67 proliferation status in sinonasal malignancies: a two-centre study
European Radiology(2022)
摘要
Objective To assess the predictive ability of a multi-parametric MRI-based radiomics signature (RS) for the preoperative evaluation of Ki-67 proliferation status in sinonasal malignancies. Methods A total of 128 patients with sinonasal malignancies that underwent multi-parametric MRIs at two medical centres were retrospectively analysed. Data from one medical centre ( n = 77) were used to develop the predictive models and data from the other medical centre ( n = 51) constitute the test dataset. Clinical data and conventional MRI findings were reviewed to identify significant predictors. Radiomics features were determined using maximum relevance minimum redundancy and least absolute shrinkage and selection operator algorithms. Subsequently, RSs were established using a logistic regression (LR) algorithm. The predictive performance of RSs was assessed using calibration, decision curve analysis (DCA), accuracy, and AUC. Results No independent predictors of high Ki-67 proliferation were observed based on clinical data and conventional MRI findings. RS-T1, RS-T2, and RS-T1c (contrast enhancement T1WI) were established based on a single-parametric MRI. RS-Combined (combining T1WI, FS-T2WI, and T1c features) was developed based on multi-parametric MRI and achieved an AUC and accuracy of 0.852 (0.733–0.971) and 86.3%, respectively, on the test dataset. The calibration curve and DCA demonstrated an improved fitness and benefits in clinical practice. Conclusions A multi-parametric MRI-based RS may be used as a non-invasive, dependable, and accurate tool for preoperative evaluation of the Ki-67 proliferation status to overcome the sampling bias in sinonasal malignancies. Key Points • Multi-parametric MRI-based radiomics signatures (RSs) are used to preoperatively evaluate the proliferation status of Ki-67 in sinonasal malignancies. • Radiomics features are determined using maximum relevance minimum redundancy (mRMR) and least absolute shrinkage and selection operator (LASSO) algorithms. • RSs are established using a logistic regression (LR) algorithm.
更多查看译文
关键词
Sinonasal, Malignant, Radiomics, Multiparametric magnetic resonance imaging, Ki-67
AI 理解论文
溯源树
样例
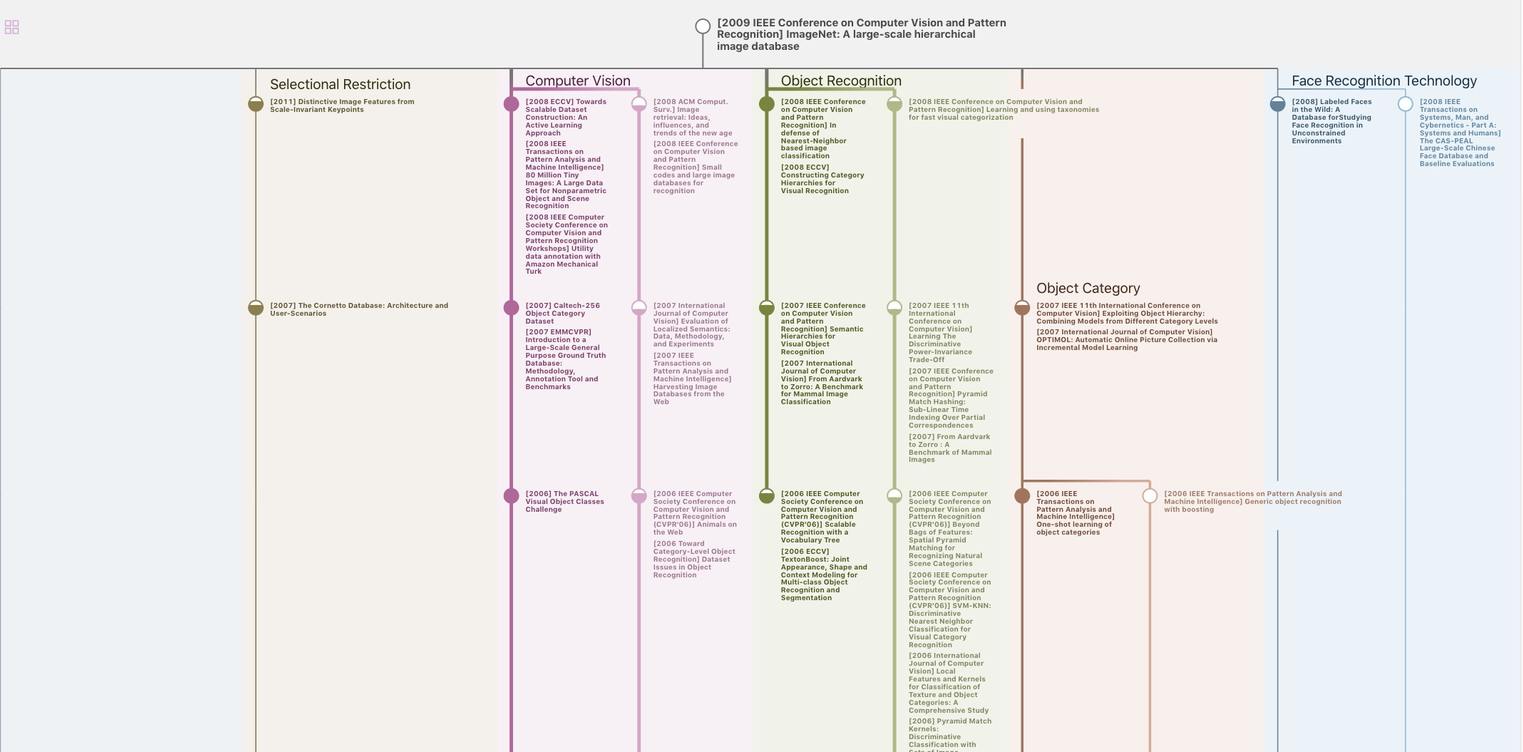
生成溯源树,研究论文发展脉络
Chat Paper
正在生成论文摘要