A Combined Semi-Supervised Deep Learning Method for Oil Leak Detection in Pipelines Using IIoT at the Edge
SENSORS(2022)
摘要
Pipelines are integral components for storing and transporting liquid and gaseous petroleum products. Despite being durable structures, ruptures can still occur, resulting not only in financial losses and energy waste but, most importantly, in immeasurable environmental disasters and possibly in human casualties. The objective of the ESTHISIS project is the development of a low-cost and efficient wireless sensor system for the instantaneous detection of leaks in metallic pipeline networks transporting liquid and gaseous petroleum products in a noisy industrial environment. The implemented methodology is based on processing the spectrum of vibration signals appearing in the pipeline walls due to a leakage effect and aims to minimize interference in the piping system. It is intended to use low frequencies to detect and characterize leakage to increase the range of sensors and thus reduce cost. In the current work, the smart sensor system developed for signal acquisition and data analysis is briefly described. For this matter, two leakage detection methodologies are implemented. A 2D-Convolutional Neural Network (CNN) model undertakes supervised classification in spectrograms extracted by the signals acquired by the accelerometers mounted on the pipeline wall. This approach allows us to supplant large-signal datasets with a more memory-efficient alternative to storing static images. Second, Long Short-Term Memory Autoencoders (LSTM AE) are employed, receiving signals from the accelerometers, and providing an unsupervised leakage detection solution.
更多查看译文
关键词
leakage detection, oil pipeline, deep learning, CNN classifier, LSTM autoencoders, edge computing
AI 理解论文
溯源树
样例
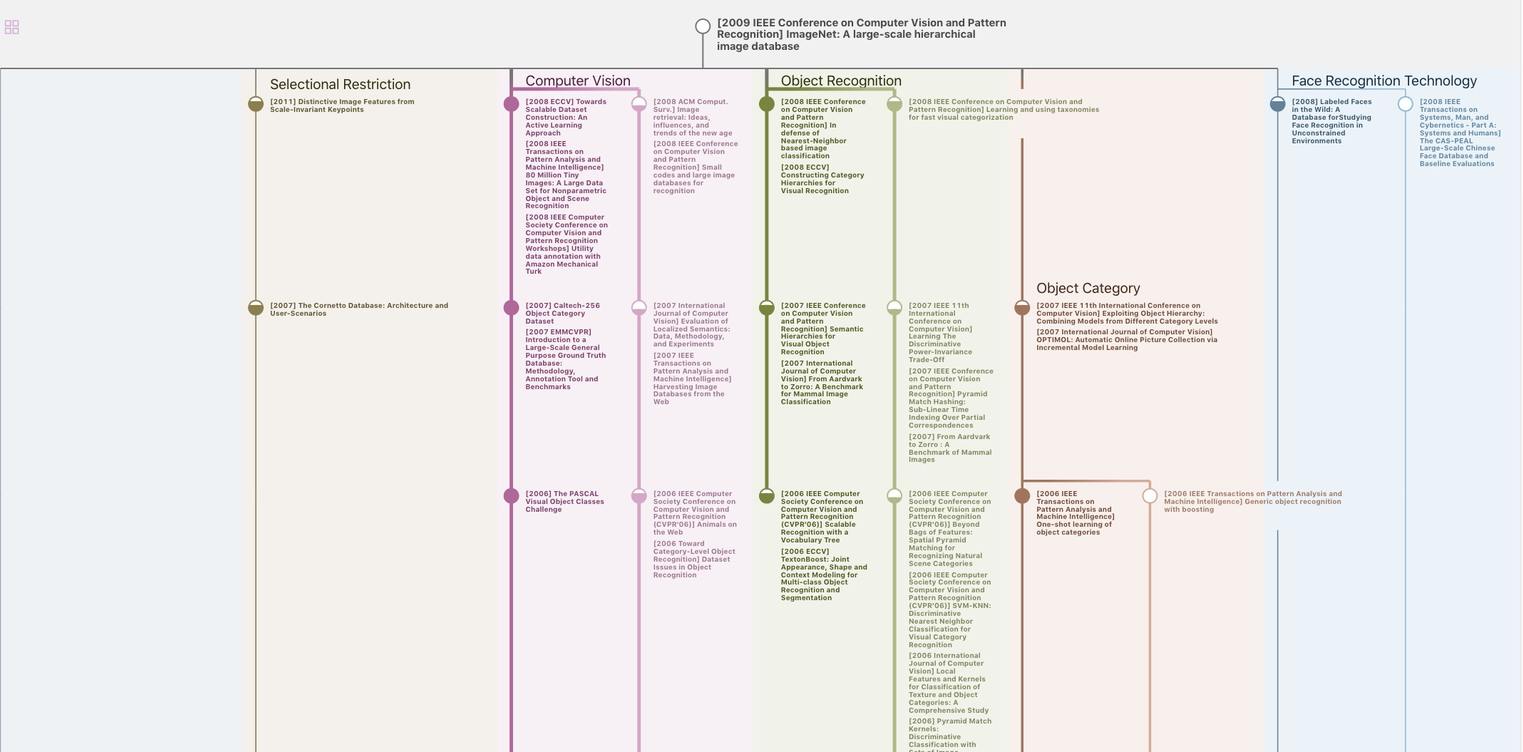
生成溯源树,研究论文发展脉络
Chat Paper
正在生成论文摘要