You Only Train Once: Learning General and Distinctive 3D Local Descriptors
IEEE Transactions on Pattern Analysis and Machine Intelligence(2023)
摘要
Extracting distinctive, robust, and general 3D local features is essential to downstream tasks such as point cloud registration. However, existing methods either rely on noise-sensitive handcrafted features, or depend on rotation-variant neural architectures. It remains challenging to learn robust and general local feature descriptors for surface matching. In this paper, we propose a new, simple yet effective neural network, termed SpinNet, to extract local surface descriptors which are rotation-invariant whilst sufficiently distinctive and general. A Spatial Point Transformer is first introduced to embed the input local surface into an elaborate cylindrical representation (SO(2) rotation-equivariant), further enabling end-to-end optimization of the entire framework. A Neural Feature Extractor, composed of point-based and 3D cylindrical convolutional layers, is then presented to learn representative and general geometric patterns. An invariant layer is finally used to generate rotation-invariant feature descriptors. Extensive experiments on both indoor and outdoor datasets demonstrate that SpinNet outperforms existing state-of-the-art techniques by a large margin. More critically, it has the best generalization ability across unseen scenarios with different sensor modalities.
更多查看译文
关键词
Cross-dataset generalization,local surface descriptor,point cloud registration,rotation invariance
AI 理解论文
溯源树
样例
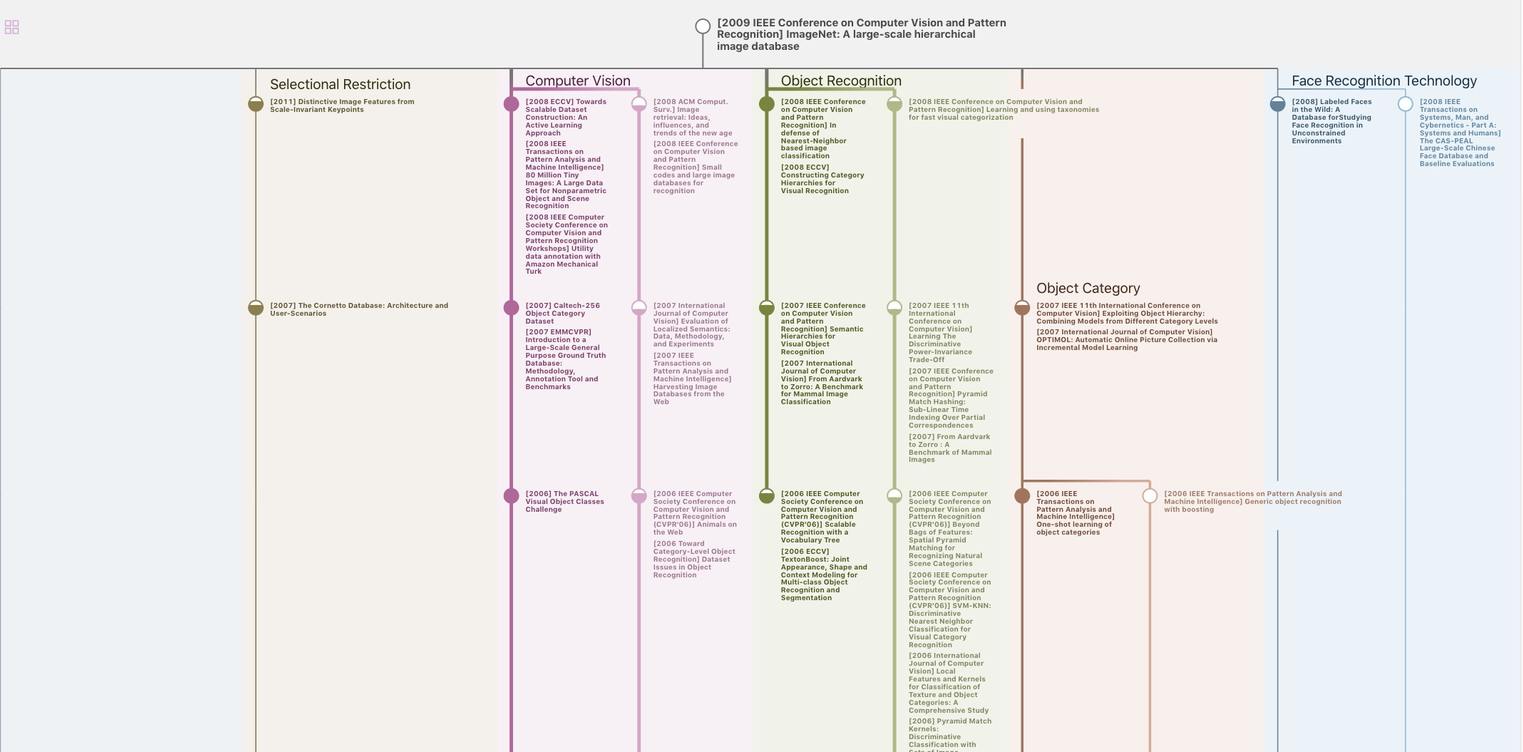
生成溯源树,研究论文发展脉络
Chat Paper
正在生成论文摘要