Hypergraph Collaborative Network on Vertices and Hyperedges
IEEE Transactions on Pattern Analysis and Machine Intelligence(2023)
摘要
In many practical datasets, such as co-citation and co-authorship, relationships across the samples are more complex than pair-wise. Hypergraphs provide a flexible and natural representation for such complex correlations and thus obtain increasing attention in the machine learning and data mining communities. Existing deep learning-based hypergraph approaches seek to learn the latent vertex representations based on either vertices or hyperedges from previous layers and focus on reducing the cross-entropy error over labeled vertices to obtain a classifier. In this paper, we propose a novel model called Hypergraph Collaborative Network (HCoN), which takes the information from both previous vertices and hyperedges into consideration to achieve informative latent representations and further introduces the hypergraph reconstruction error as a regularizer to learn an effective classifier. We evaluate the proposed method on two cases, i.e., semi-supervised vertex and hyperedge classifications. We carry out the experiments on several benchmark datasets and compare our method with several state-of-the-art approaches. Experimental results demonstrate that the performance of the proposed method is better than that of the baseline methods.
更多查看译文
关键词
Edge classification,hypergraph,hypergraph convolution,vertex classification
AI 理解论文
溯源树
样例
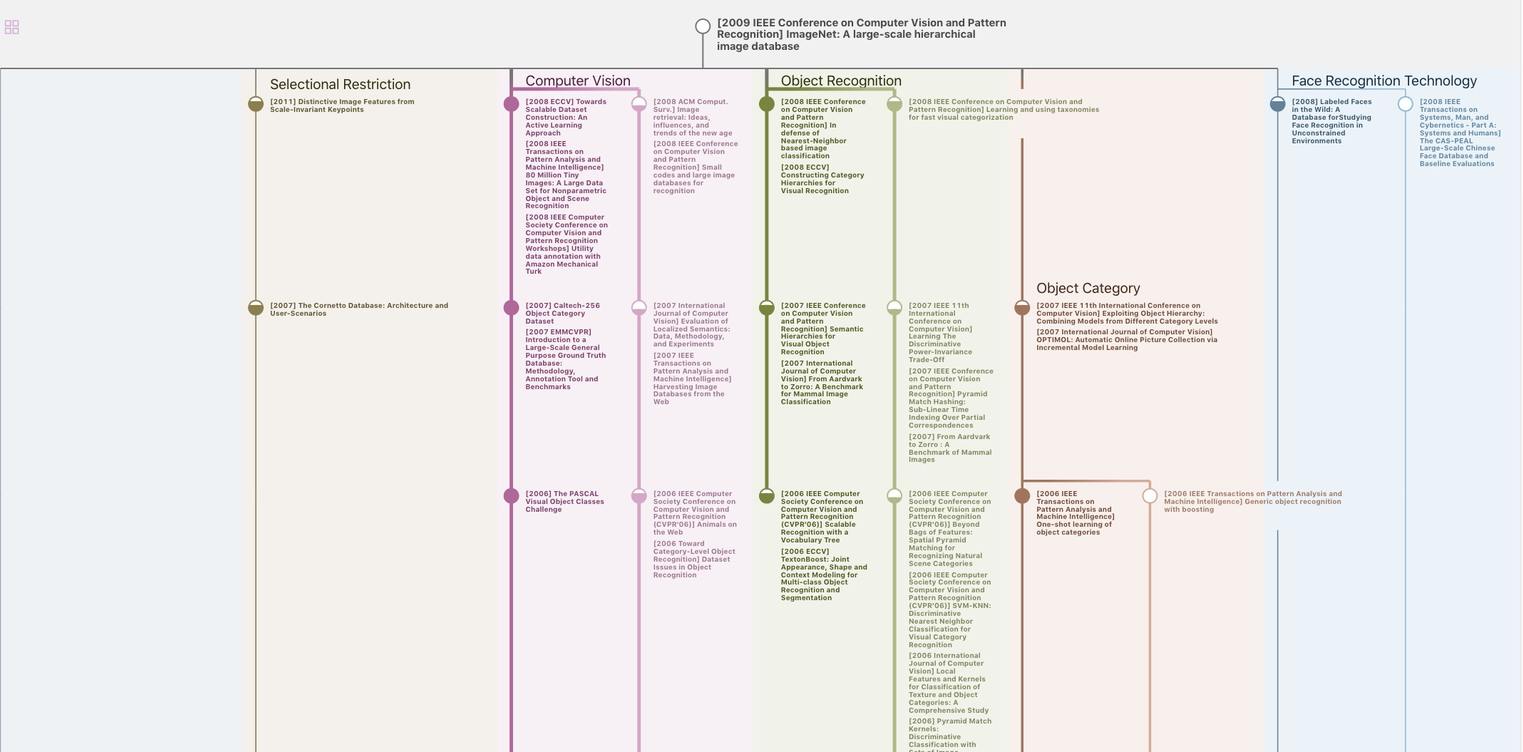
生成溯源树,研究论文发展脉络
Chat Paper
正在生成论文摘要