Analytical Composition of Differential Privacy via the Edgeworth Accountant
arxiv(2022)
摘要
Many modern machine learning algorithms are composed of simple private algorithms; thus, an increasingly important problem is to efficiently compute the overall privacy loss under composition. In this study, we introduce the Edgeworth Accountant, an analytical approach to composing differential privacy guarantees of private algorithms. The Edgeworth Accountant starts by losslessly tracking the privacy loss under composition using the $f$-differential privacy framework, which allows us to express the privacy guarantees using privacy-loss log-likelihood ratios (PLLRs). As the name suggests, this accountant next uses the Edgeworth expansion to the upper and lower bounds the probability distribution of the sum of the PLLRs. Moreover, by relying on a technique for approximating complex distributions using simple ones, we demonstrate that the Edgeworth Accountant can be applied to the composition of any noise-addition mechanism. Owing to certain appealing features of the Edgeworth expansion, the $(\epsilon, \delta)$-differential privacy bounds offered by this accountant are non-asymptotic, with essentially no extra computational cost, as opposed to the prior approaches in, wherein the running times increase with the number of compositions. Finally, we demonstrate that our upper and lower $(\epsilon, \delta)$-differential privacy bounds are tight in federated analytics and certain regimes of training private deep learning models.
更多查看译文
关键词
Differential Privacy,f-Differential Privacy,Edgeworth Expansion,PLLR,Edgeworth Accountant
AI 理解论文
溯源树
样例
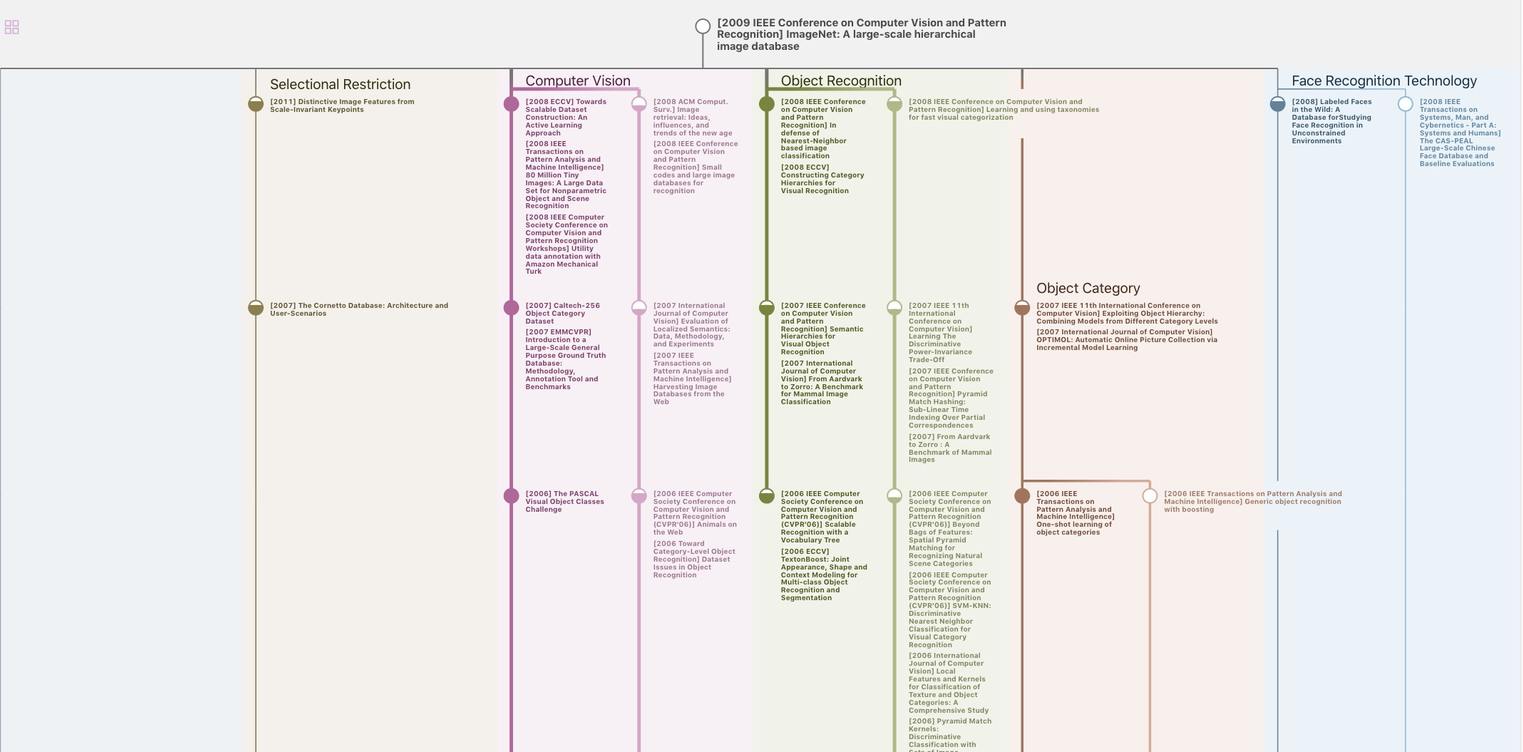
生成溯源树,研究论文发展脉络
Chat Paper
正在生成论文摘要