JNMR: Joint Non-linear Motion Regression for Video Frame Interpolation
arxiv(2023)
摘要
Video frame interpolation (VFI) aims to generate predictive frames by warping learnable motions from the bidirectional historical references. Most existing works utilize spatio-temporal semantic information extractor to realize motion estimation and interpolation modeling. However, they insufficiently consider the real mechanistic rationality of generated middle motions. In this paper, we reformulate VFI as a Joint Non-linear Motion Regression (JNMR) strategy to model the complicated motions of inter-frame. Specifically, the motion trajectory between the target frame and the multiple reference frames is regressed by a temporal concatenation of multi-stage quadratic models. ConvLSTM is adopted to construct this joint distribution of complete motions in temporal dimension. Moreover, the feature learning network is designed to optimize for the joint regression modeling. A coarse-to-fine synthesis enhancement module is also conducted to learn visual dynamics at different resolutions through repetitive regression and interpolation. Experimental results on VFI show that the effectiveness and significant improvement of joint motion regression compared with the state-of-the-art methods.
更多查看译文
关键词
motion,frame,video,non-linear
AI 理解论文
溯源树
样例
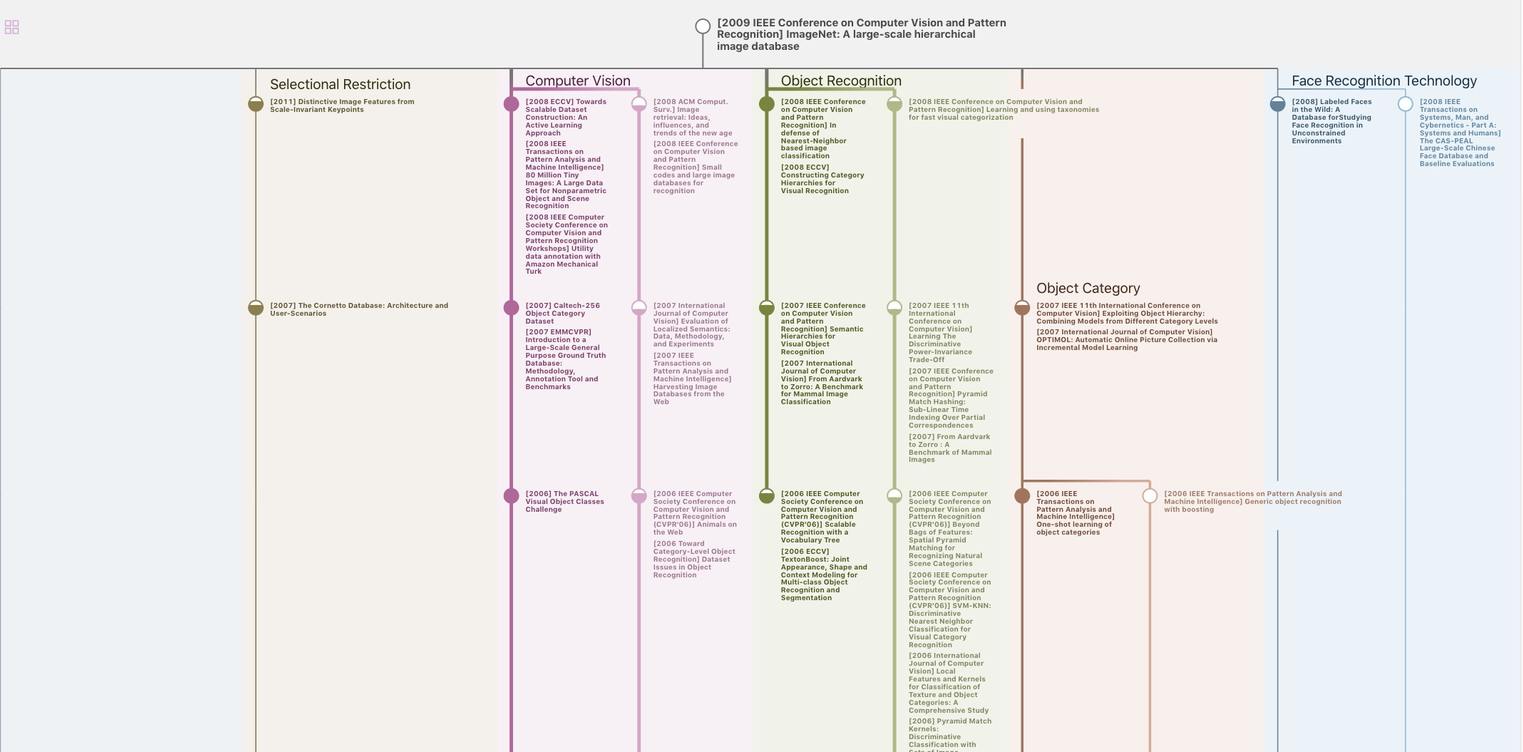
生成溯源树,研究论文发展脉络
Chat Paper
正在生成论文摘要