Blind face restoration: Benchmark datasets and a baseline model
NEUROCOMPUTING(2024)
摘要
Blind Face Restoration (BFR) aims to generate high -quality face images from low -quality inputs. However, existing BFR methods often use private datasets for training and evaluation, making it challenging for future approaches to compare fairly. To address this issue, we introduce two benchmark datasets, BFRBD128 and BFRBD512, for evaluating state-of-the-art methods in five scenarios: blur, noise, low resolution, JPEG compression artifacts, and full degradation. We use seven standard quantitative metrics and two task -specific metrics, AFLD and AFICS. Additionally, we propose an efficient baseline model called Swin Transformer U -Net (STUNet), which outperforms state-of-the-art methods in various BFR tasks. The codes, datasets, and trained models are publicly available at: https://github.com/bitzpy/Blind-Face-Restoration-Benchmark-Datasets-anda-Baseline-Model.
更多查看译文
关键词
Blind face restoration,Benchmark datasets,Comprehensive evaluation,Transformer network
AI 理解论文
溯源树
样例
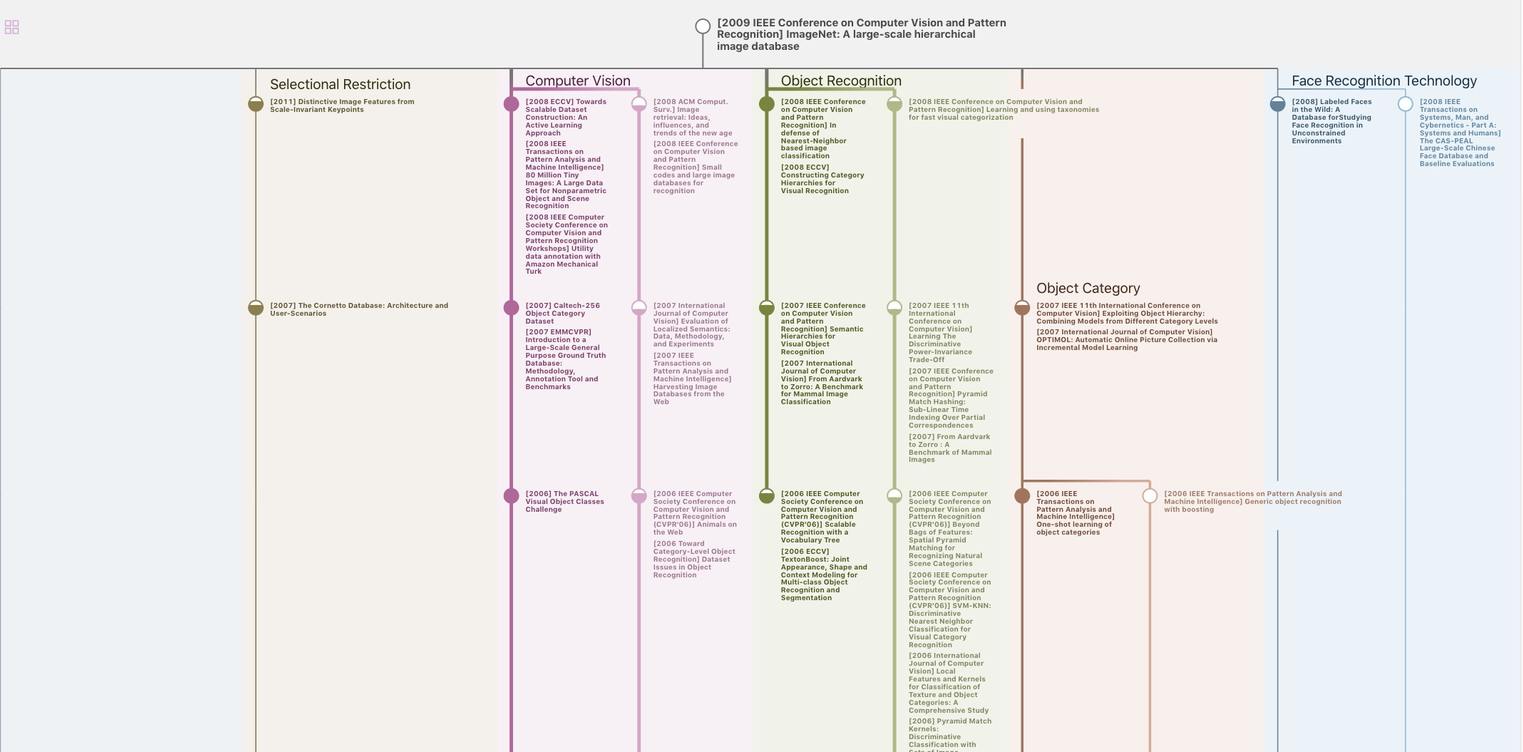
生成溯源树,研究论文发展脉络
Chat Paper
正在生成论文摘要