Identifying Chirality in Line Drawings of Molecules Using Imbalanced Dataset Sampler for a Multilabel Classification Task.
Molecular informatics(2022)
摘要
Chirality, the ability of some molecules to exist as two non-superimposable mirror images, profoundly influences both chemistry and biology. Advances in deep learning enable the automatic recognition of chemical structure diagrams, however, studies on discovering the molecule chirality are scarce and the machine-readable molecular representations are not always sufficient to fully support the encoding of this important property. Here, we pretrained networks on a ChEMBL+ dataset (79641 molecules) and fine-tuned them for the binary classification of chirality (achiral/chiral) or multilabel chirality type classifications (none/centre/axial/planar). To address the label combination imbalanced problem in the multilabel task, the study proposed a Formulated Imbalanced Dataset Sampler (FIDS) to sample a formulated amount of minority label combinations on top of the training set. On a 10-fold cross validation experiment using our CHIRAL dataset (1142 manually curated molecules), our models achieved up to an accuracy of 90 % in the binary task. In the multilabel task incorporated with FIDS, the overall performance increases from 87 % to 89 % and the accuracy per label combination can attained up to a 50 % increase. Through the study of heatmaps, our work also exemplified the potential of deep neural network to make predictions based on the actual location of chirality elements.
更多查看译文
关键词
Chirality,Classification,Convolutional Neural Network,Deep Learning,Image Recognition
AI 理解论文
溯源树
样例
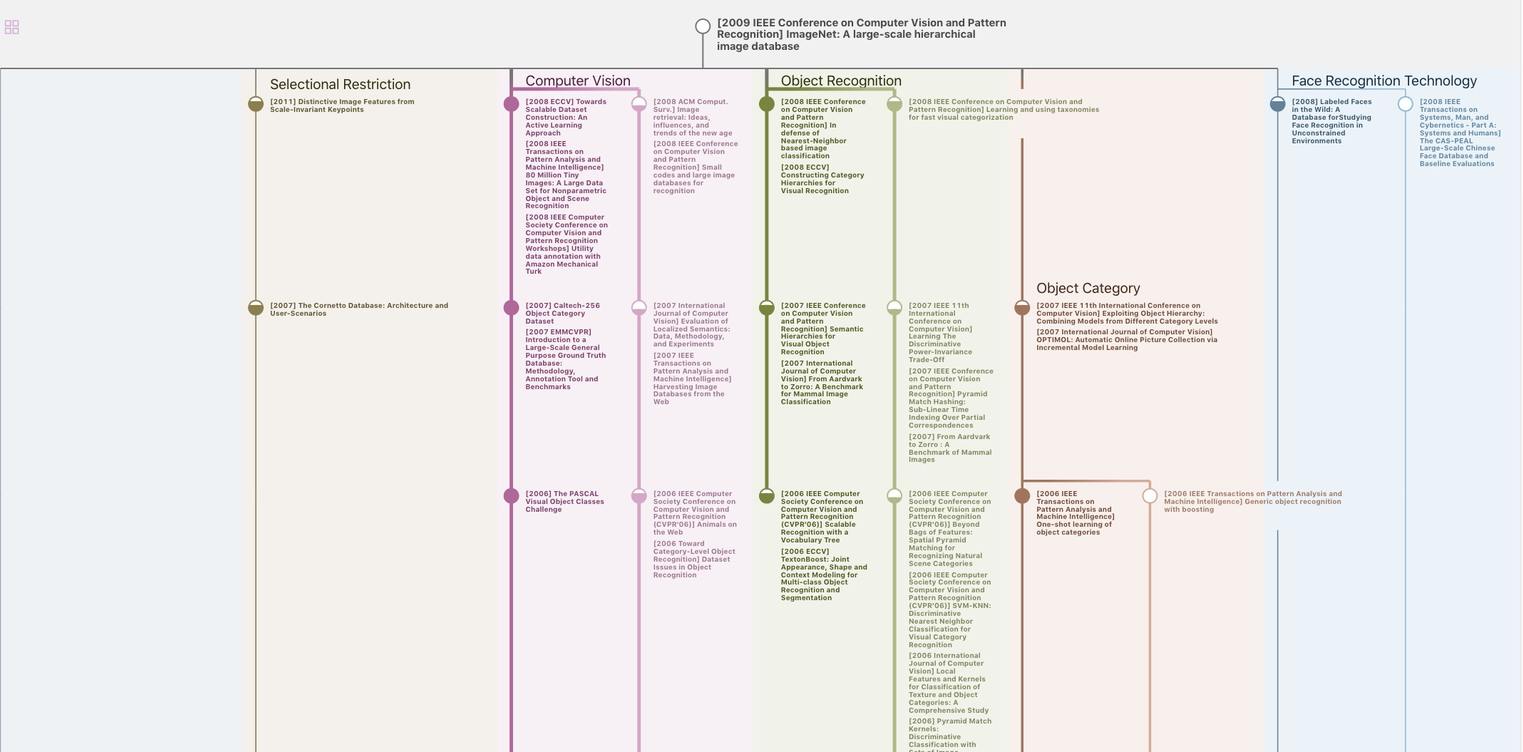
生成溯源树,研究论文发展脉络
Chat Paper
正在生成论文摘要