Machine Learning Classifiers to Evaluate Data From Gait Analysis With Depth Cameras in Patients With Parkinson's Disease
FRONTIERS IN HUMAN NEUROSCIENCE(2022)
摘要
Introduction: The assessments of the motor symptoms in Parkinson's disease (PD) are usually limited to clinical rating scales (MDS UPDRS III), and it depends on the clinician's experience. This study aims to propose a machine learning technique algorithm using the variables from upper and lower limbs, to classify people with PD from healthy people, using data from a portable low-cost device (RGB-D camera). And can be used to support the diagnosis and follow-up of patients in developing countries and remote areas. Methods: We used Kinect (R) eMotion system to capture the spatiotemporal gait data from 30 patients with PD and 30 healthy age-matched controls in three walking trials. First, a correlation matrix was made using the variables of upper and lower limbs. After this, we applied a backward feature selection model using R and Python to determine the most relevant variables. Three further analyses were done using variables selected from backward feature selection model (Dataset A), movement disorders specialist (Dataset B), and all the variables from the dataset (Dataset C). We ran seven machine learning models for each model. Dataset was divided 80% for algorithm training and 20% for evaluation. Finally, a causal inference model (CIM) using the DoWhy library was performed on Dataset B due to its accuracy and simplicity. Results: The Random Forest model is the most accurate for all three variable Datasets (Dataset A: 81.8%; Dataset B: 83.6%; Dataset C: 84.5%) followed by the support vector machine. The CIM shows a relation between leg variables and the arms swing asymmetry (ASA) and a proportional relationship between ASA and the diagnosis of PD with a robust estimator (1,537). Conclusions: Machine learning techniques based on objective measures using portable low-cost devices (Kinect (R) eMotion) are useful and accurate to classify patients with Parkinson's disease. This method can be used to evaluate patients remotely and help clinicians make decisions regarding follow-up and treatment.
更多查看译文
关键词
Parkinson's disease, gait, biomechanics, kinect, depth camera, machine learning
AI 理解论文
溯源树
样例
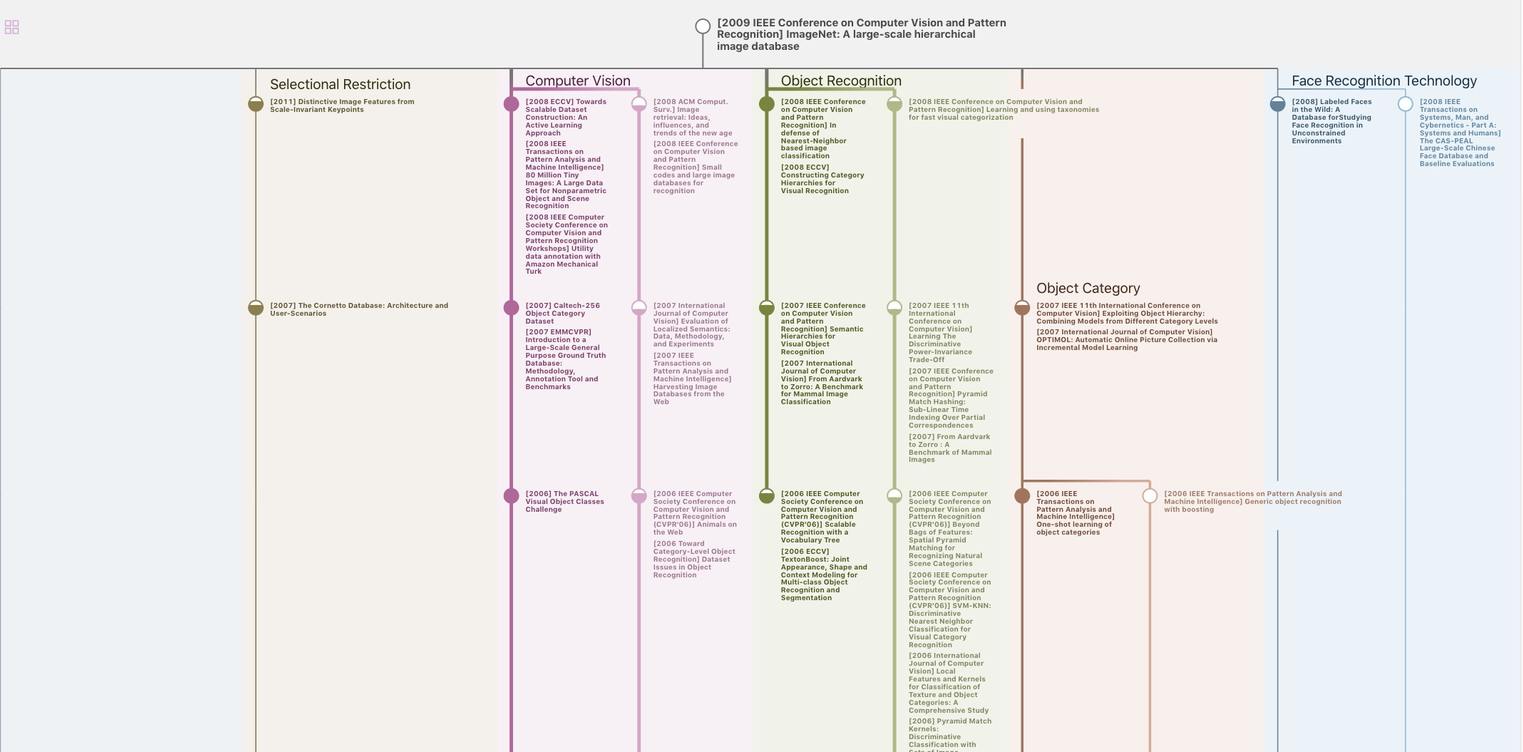
生成溯源树,研究论文发展脉络
Chat Paper
正在生成论文摘要