Generating Long Videos of Dynamic Scenes
NeurIPS 2022(2022)
摘要
We present a video generation model that accurately reproduces object motion, changes in camera viewpoint, and new content that arises over time. Existing video generation methods often fail to produce new content as a function of time while maintaining consistencies expected in real environments, such as plausible dynamics and object persistence. A common failure case is for content to never change due to over-reliance on inductive biases to provide temporal consistency, such as a single latent code that dictates content for the entire video. On the other extreme, without long-term consistency, generated videos may morph unrealistically between different scenes. To address these limitations, we prioritize the time axis by redesigning the temporal latent representation and learning long-term consistency from data by training on longer videos. To this end, we leverage a two-phase training strategy, where we separately train using longer videos at a low resolution and shorter videos at a high resolution. To evaluate the capabilities of our model, we introduce two new benchmark datasets with explicit focus on long-term temporal dynamics.
更多查看译文
关键词
Video generation,GAN,generative model,dynamics,long videos
AI 理解论文
溯源树
样例
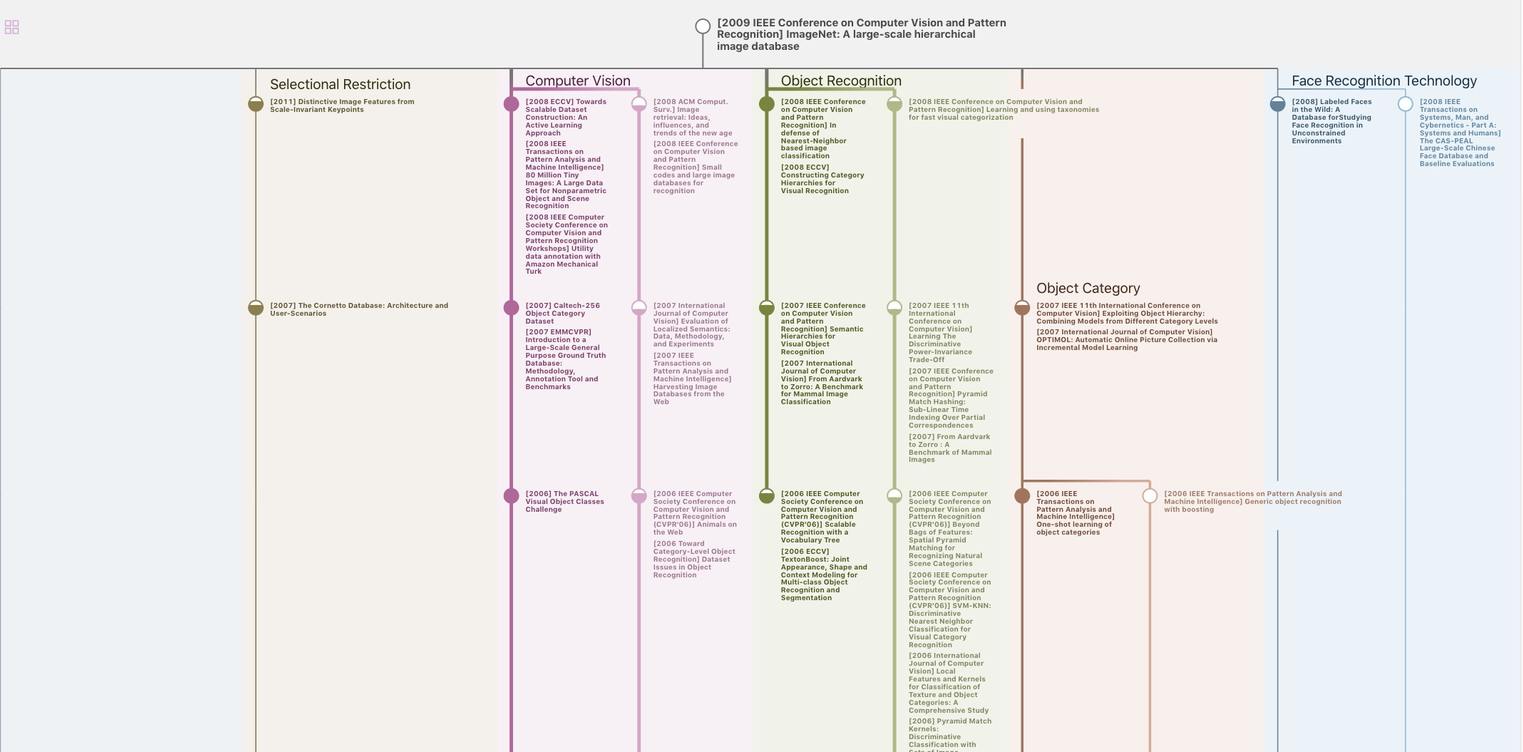
生成溯源树,研究论文发展脉络
Chat Paper
正在生成论文摘要