Imitating Past Successes can be Very Suboptimal
arxiv(2023)
摘要
Prior work has proposed a simple strategy for reinforcement learning (RL): label experience with the outcomes achieved in that experience, and then imitate the relabeled experience. These outcome-conditioned imitation learning methods are appealing because of their simplicity, strong performance, and close ties with supervised learning. However, it remains unclear how these methods relate to the standard RL objective, reward maximization. In this paper, we formally relate outcome-conditioned imitation learning to reward maximization, drawing a precise relationship between the learned policy and Q-values and explaining the close connections between these methods and prior EM-based policy search methods. This analysis shows that existing outcome-conditioned imitation learning methods do not necessarily improve the policy, but a simple modification results in a method that does guarantee policy improvement, under some assumptions.
更多查看译文
关键词
past successes
AI 理解论文
溯源树
样例
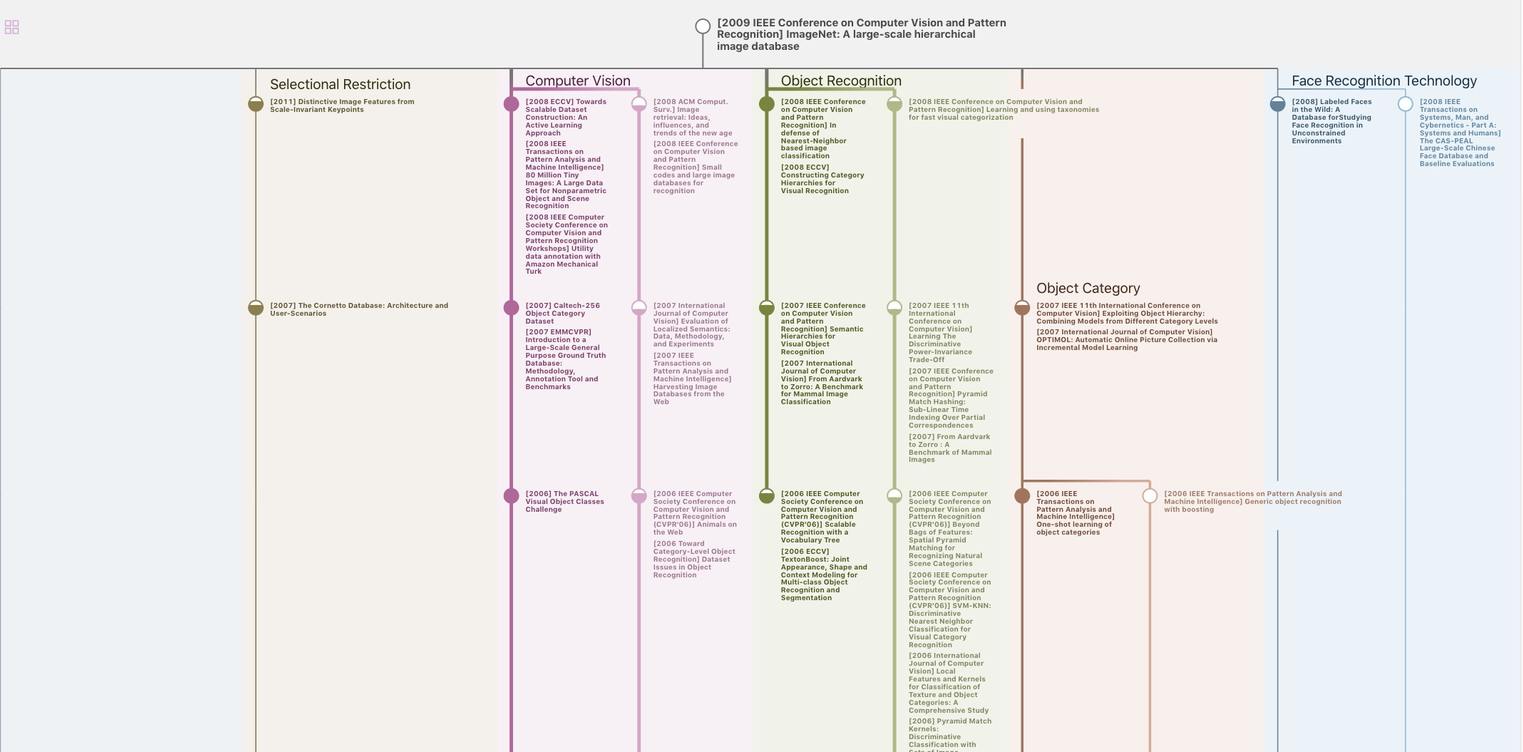
生成溯源树,研究论文发展脉络
Chat Paper
正在生成论文摘要