Subject Membership Inference Attacks in Federated Learning
arxiv(2022)
摘要
Privacy attacks on Machine Learning (ML) models often focus on inferring the existence of particular data points in the training data. However, what the adversary really wants to know is if a particular \emph{individual}'s (\emph{subject}'s) data was included during training. In such scenarios, the adversary is more likely to have access to the distribution of a particular subject, than actual records. Furthermore, in settings like cross-silo Federated Learning (FL), a subject's data can be embodied by multiple data records that are spread across multiple organizations. Nearly all of the existing private FL literature is dedicated to studying privacy at two granularities -- item-level (individual data records), and user-level (participating user in the federation), neither of which apply to data subjects in cross-silo FL. This insight motivates us to shift our attention from the privacy of data records to the privacy of \emph{data subjects}, also known as subject-level privacy. We propose two black-box attacks for \emph{subject membership inference}, of which one assumes access to a model after each training round. Using these attacks, we estimate subject membership inference risk on real-world data for single-party models as well as FL scenarios. We find our attacks to be extremely potent, even without access to exact training records, and using the knowledge of membership for a handful of subjects. To better understand the various factors that may influence subject privacy risk in cross-silo FL settings, we systematically generate several hundred synthetic federation configurations, varying properties of the data, model design and training, and the federation itself. Finally, we investigate the effectiveness of Differential Privacy in mitigating this threat.
更多查看译文
关键词
subject,learning
AI 理解论文
溯源树
样例
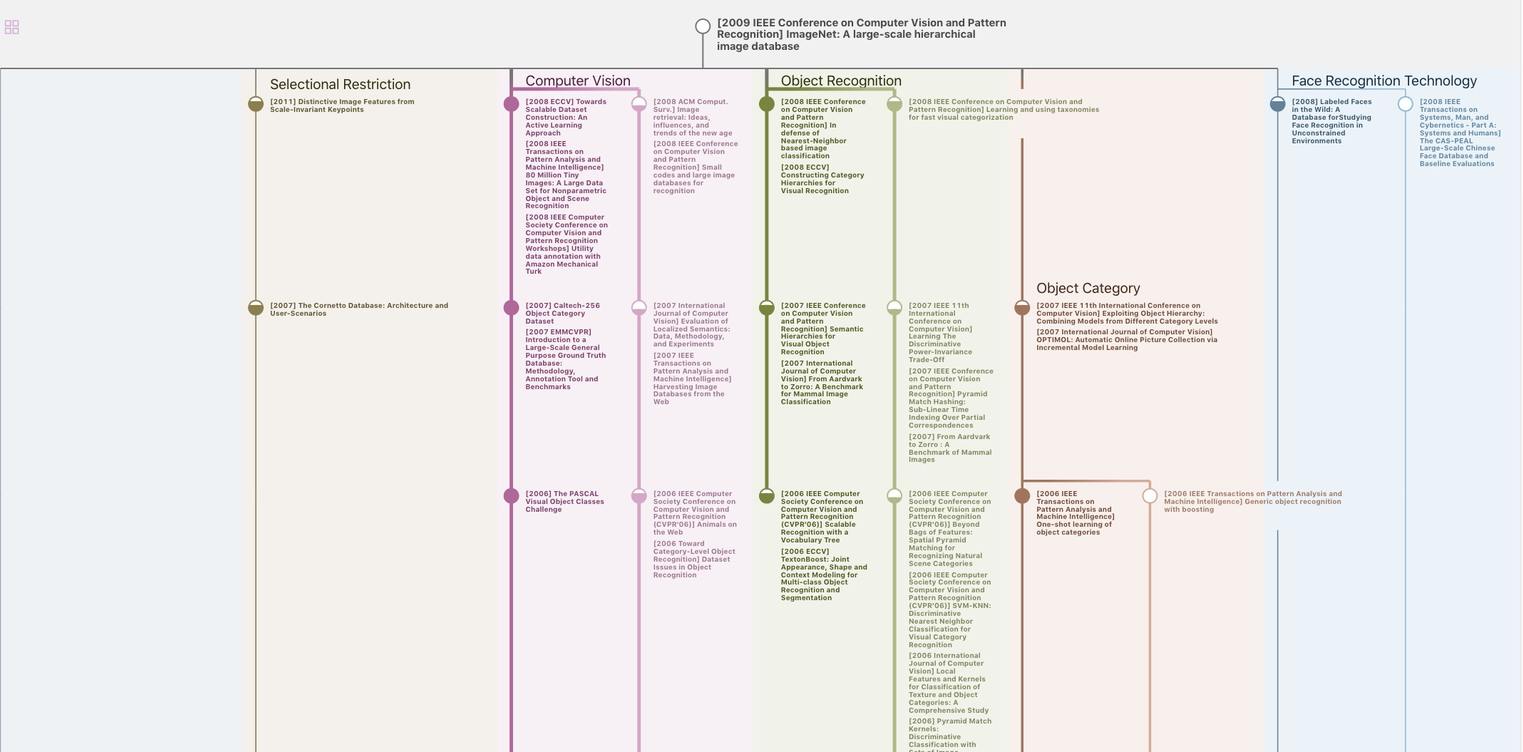
生成溯源树,研究论文发展脉络
Chat Paper
正在生成论文摘要