Siamese Encoder-based Spatial-Temporal Mixer for Growth Trend Prediction of Lung Nodules on CT Scans
MEDICAL IMAGE COMPUTING AND COMPUTER ASSISTED INTERVENTION, MICCAI 2022, PT I(2022)
摘要
In the management of lung nodules, we are desirable to predict nodule evolution in terms of its diameter variation on Computed Tomography (CT) scans and then provide a follow-up recommendation according to the predicted result of the growing trend of the nodule. In order to improve the performance of growth trend prediction for lung nodules, it is vital to compare the changes of the same nodule in consecutive CT scans. Motivated by this, we screened out 4,666 subjects with more than two consecutive CT scans from the National Lung Screening Trial (NLST) dataset to organize a temporal dataset called NLSTt. In specific, we first detect and pair regions of interest (ROIs) covering the same nodule based on registered CT scans. After that, we predict the texture category and diameter size of the nodules through models. Last, we annotate the evolution class of each nodule according to its changes in diameter. Based on the built NLSTt dataset, we propose a siamese encoder to simultaneously exploit the discriminative features of 3D ROIs detected from consecutive CT scans. Then we novelly design a spatial-temporal mixer (STM) to leverage the interval changes of the same nodule in sequential 3D ROIs and capture spatial dependencies of nodule regions and the current 3D ROI. According to the clinical diagnosis routine, we employ hierarchical loss to pay more attention to growing nodules. The extensive experiments on our organized dataset demonstrate the advantage of our proposed method. We also conduct experiments on an in-house dataset to evaluate the clinical utility of our method by comparing it against skilled clinicians. STM code and NLSTt dataset are available at https://github.com/liaw05/STMixer.
更多查看译文
关键词
Lung nodule, Growth trend prediction, Siamese network, Feature fusion, Hierarchical loss, Spatial-temporal information
AI 理解论文
溯源树
样例
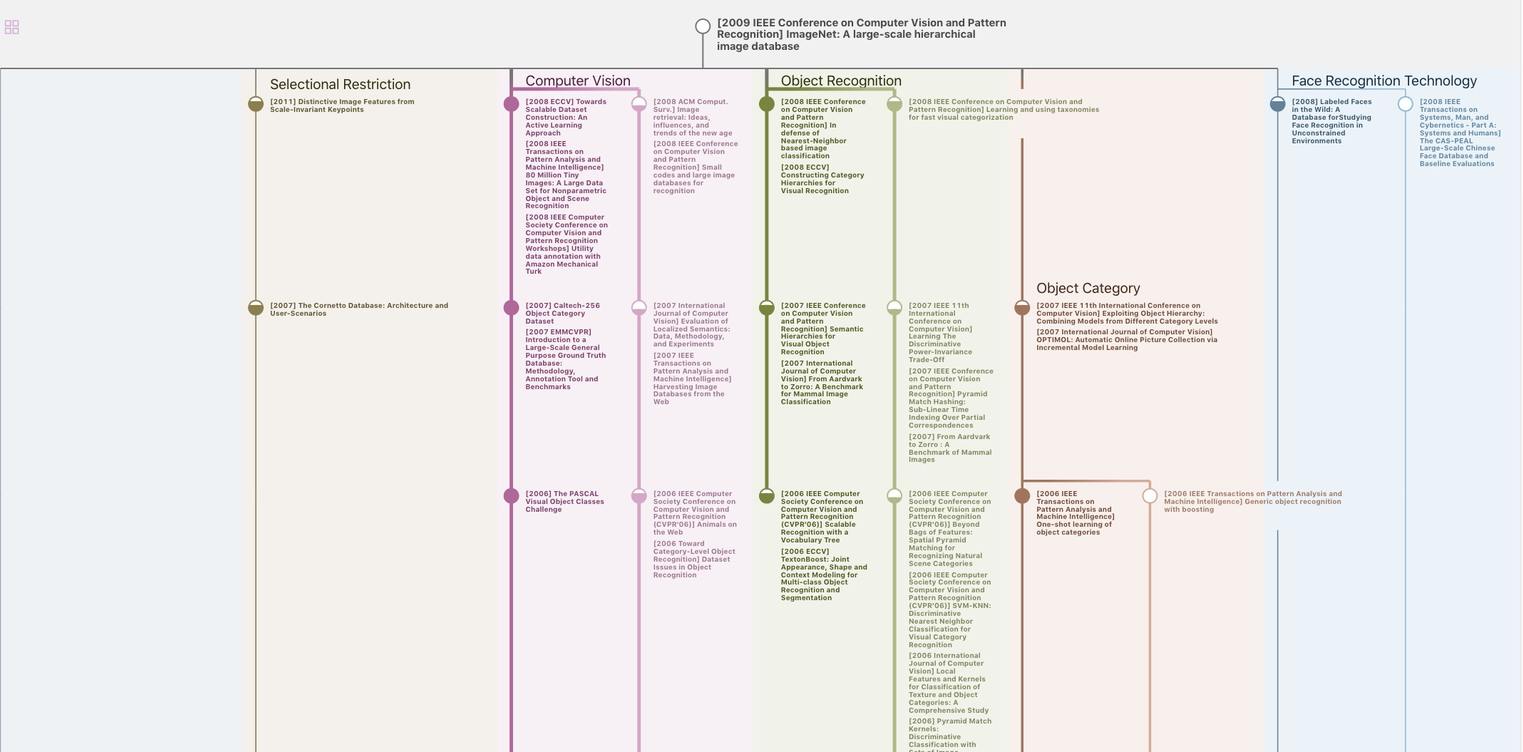
生成溯源树,研究论文发展脉络
Chat Paper
正在生成论文摘要