DriveIRL: Drive in Real Life with Inverse Reinforcement Learning
2023 IEEE INTERNATIONAL CONFERENCE ON ROBOTICS AND AUTOMATION, ICRA(2023)
摘要
In this paper, we introduce the first published planner to drive a car in dense, urban traffic using Inverse Reinforcement Learning (IRL). Our planner, DriveIRL, generates a diverse set of trajectory proposals and scores them with a learned model. The best trajectory is tracked by our self-driving vehicle's low-level controller. We train our trajectory scoring model on a 500+ hour real-world dataset of expert driving demonstrations in Las Vegas within the maximum entropy IRL framework. DriveIRL's benefits include: a simple design due to only learning the trajectory scoring function, a flexible and relatively interpretable feature engineering approach, and strong real-world performance. We validated DriveIRL on the Las Vegas Strip and demonstrated fully autonomous driving in heavy traffic, including scenarios involving cut-ins, abrupt braking by the lead vehicle, and hotel pickup/dropoff zones. Our dataset, a part of nuPlan, has been released to the public to help further research in this area.
更多查看译文
关键词
ML-based Planning,Inverse Reinforcement Learning,Real-World Deployment,Self-Driving,Autonomous Vehicles,Urban Driving,Learning from Human Driving
AI 理解论文
溯源树
样例
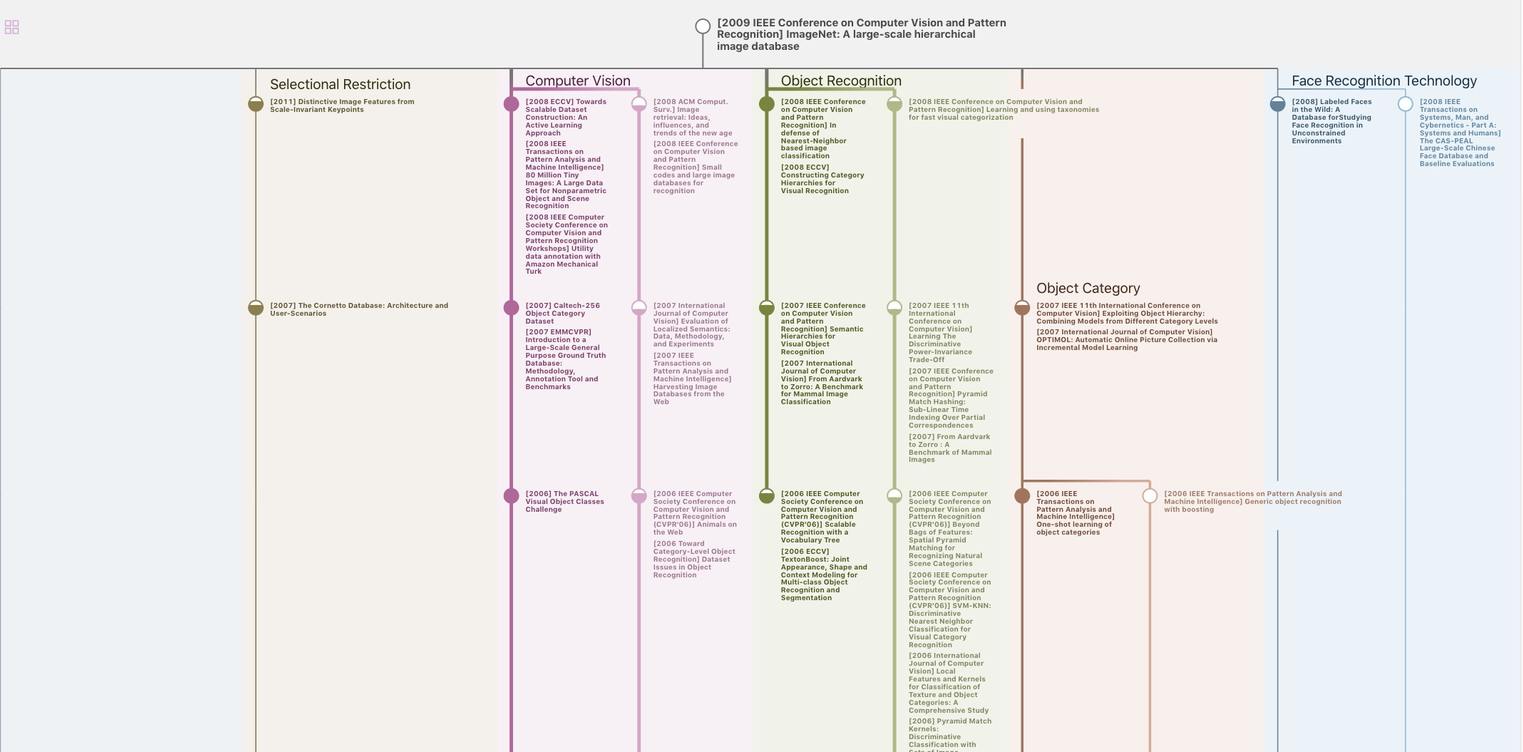
生成溯源树,研究论文发展脉络
Chat Paper
正在生成论文摘要