DETR++: Taming Your Multi-Scale Detection Transformer
arxiv(2022)
摘要
Convolutional Neural Networks (CNN) have dominated the field of detection ever since the success of AlexNet in ImageNet classification [12]. With the sweeping reform of Transformers [27] in natural language processing, Carion et al. [2] introduce the Transformer-based detection method, i.e., DETR. However, due to the quadratic complexity in the self-attention mechanism in the Transformer, DETR is never able to incorporate multi-scale features as performed in existing CNN-based detectors, leading to inferior results in small object detection. To mitigate this issue and further improve performance of DETR, in this work, we investigate different methods to incorporate multi-scale features and find that a Bi-directional Feature Pyramid (BiFPN) works best with DETR in further raising the detection precision. With this discovery, we propose DETR++, a new architecture that improves detection results by 1.9% AP on MS COCO 2017, 11.5% AP on RICO icon detection, and 9.1% AP on RICO layout extraction over existing baselines.
更多查看译文
关键词
transformer,detection,multi-scale
AI 理解论文
溯源树
样例
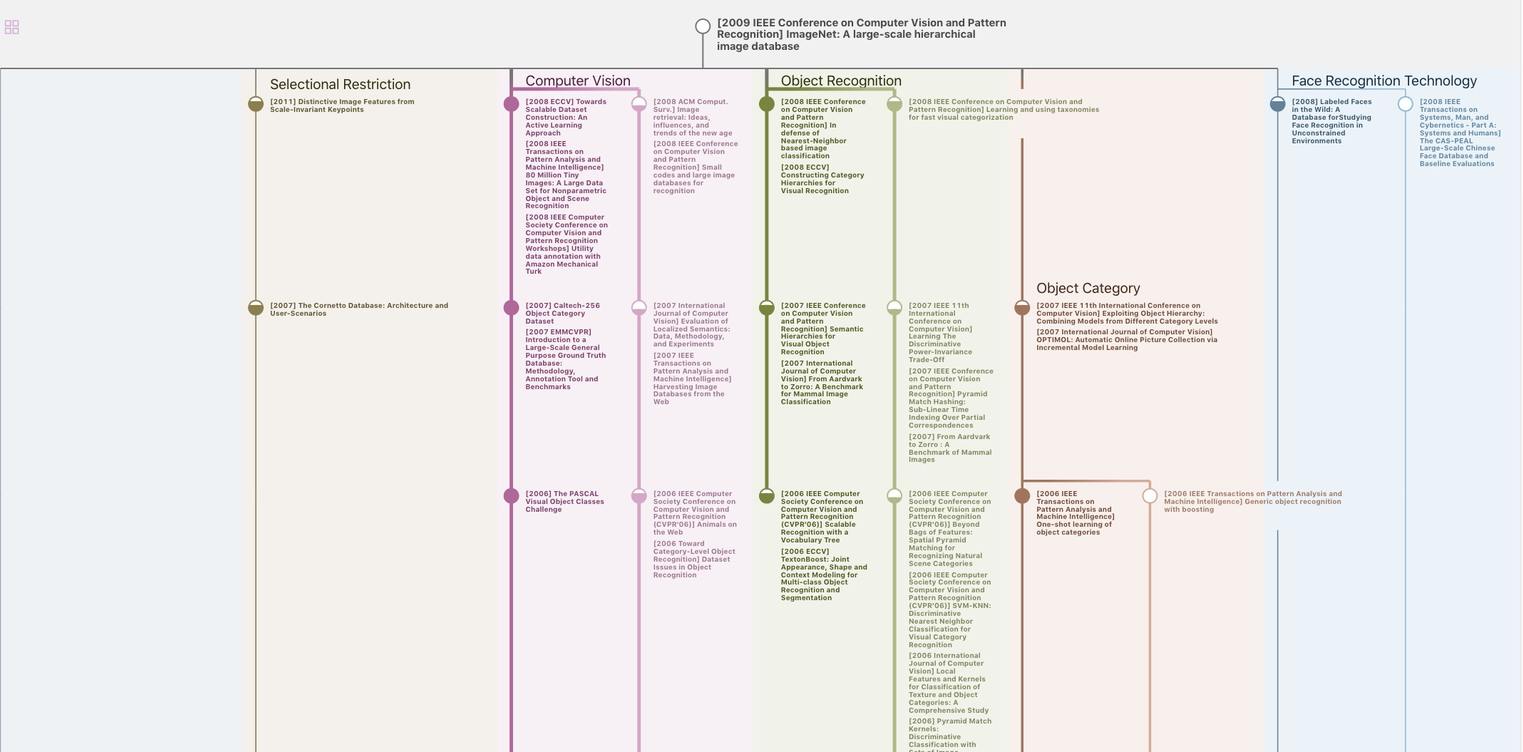
生成溯源树,研究论文发展脉络
Chat Paper
正在生成论文摘要