Characterization of the continuous transcriptional heterogeneity in Wilms tumors using unsupervised machine learning
biorxiv(2022)
摘要
Wilms′ tumors are pediatric malignancies that are thought to arise from faulty kidney development. To date, the course of treatment depends on manual histopathological classification which is difficult since the tumors differ between patients in a continuous manner. Here, we used three computational approaches to characterize the continuous heterogeneity of Wilms′ tumors. We first chose a published dataset of microarray gene expression measurements from high-risk blastemal-type Wilms′ tumors. Then, we used Pareto Task Inference to show that the tumors form a triangle-shaped continuum in latent space that is bounded by three tumor archetypes with ″stromal″, ″epithelial″, and ″blastemal″ characteristics, that resemble the un-induced mesenchyme, the Cap mesenchyme, and early epithelial structures of the fetal kidney. We confirmed this by fitting a generative probabilistic ″grade of membership″ model whereby each tumor is represented as a unique mixture of three hidden ″topics″ with blastemal, stromal, and epithelial characteristics. Finally, we used cellular deconvolution to show that each tumor is composed of a unique mixture of cell populations resembling the un-induced mesenchyme, the cap mesenchyme, and the early epithelial structures of the fetal kidney. We anticipate that these methodologies will pave the way for more quantitative strategies for tumor stratification and classification.
### Competing Interest Statement
The authors have declared no competing interest.
更多查看译文
关键词
continuous transcriptional heterogeneity,wilms,machine learning,tumors
AI 理解论文
溯源树
样例
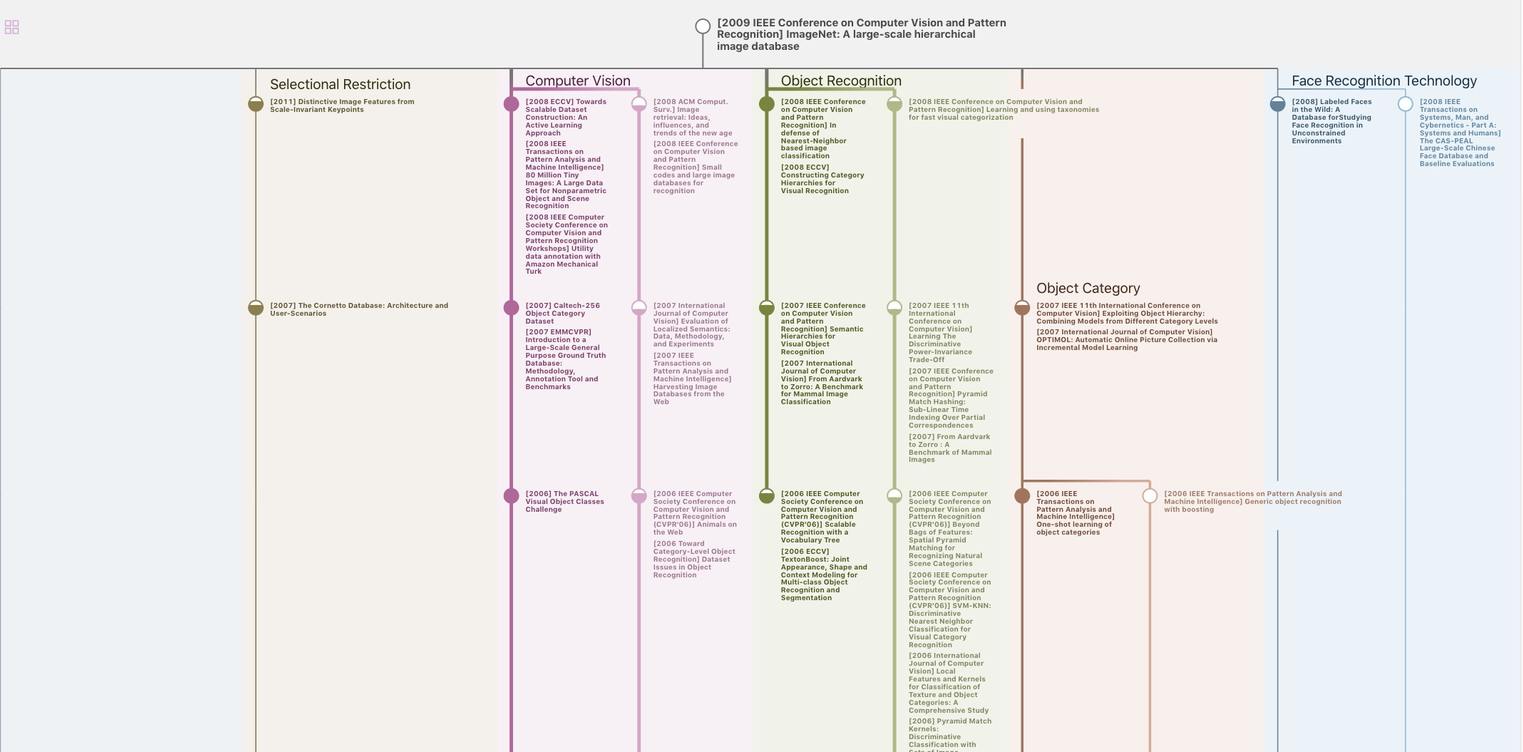
生成溯源树,研究论文发展脉络
Chat Paper
正在生成论文摘要