Continuous and Distribution-Free Probabilistic Wind Power Forecasting: A Conditional Normalizing Flow Approach
IEEE Transactions on Sustainable Energy(2022)
Abstract
We present a data-driven approach for probabilistic wind power forecasting based on conditional normalizing flow (CNF). In contrast with the existing, this approach is distribution-free (as for non-parametric and quantile-based approaches) and can directly yield continuous probability densities, hence avoiding quantile crossing. It relies on a base distribution and a set of bijective mappings. Both the shape parameters of the base distribution and the bijective mappings are approximated with neural networks. Spline-based conditional normalizing flow is considered owing to its non-affine characteristics. Over the training phase, the model sequentially maps input examples onto samples of base distribution, given the conditional contexts, where parameters are estimated through maximum likelihood. To issue probabilistic forecasts, one eventually maps samples of the base distribution into samples of a desired distribution. Case studies based on open datasets validate the effectiveness of the proposed model, and allows us to discuss its advantages and caveats with respect to the state of the art.
MoreTranslated text
Key words
Conditional normalizing flow,deep learning,density estimation,probabilistic forecasting,wind power
AI Read Science
Must-Reading Tree
Example
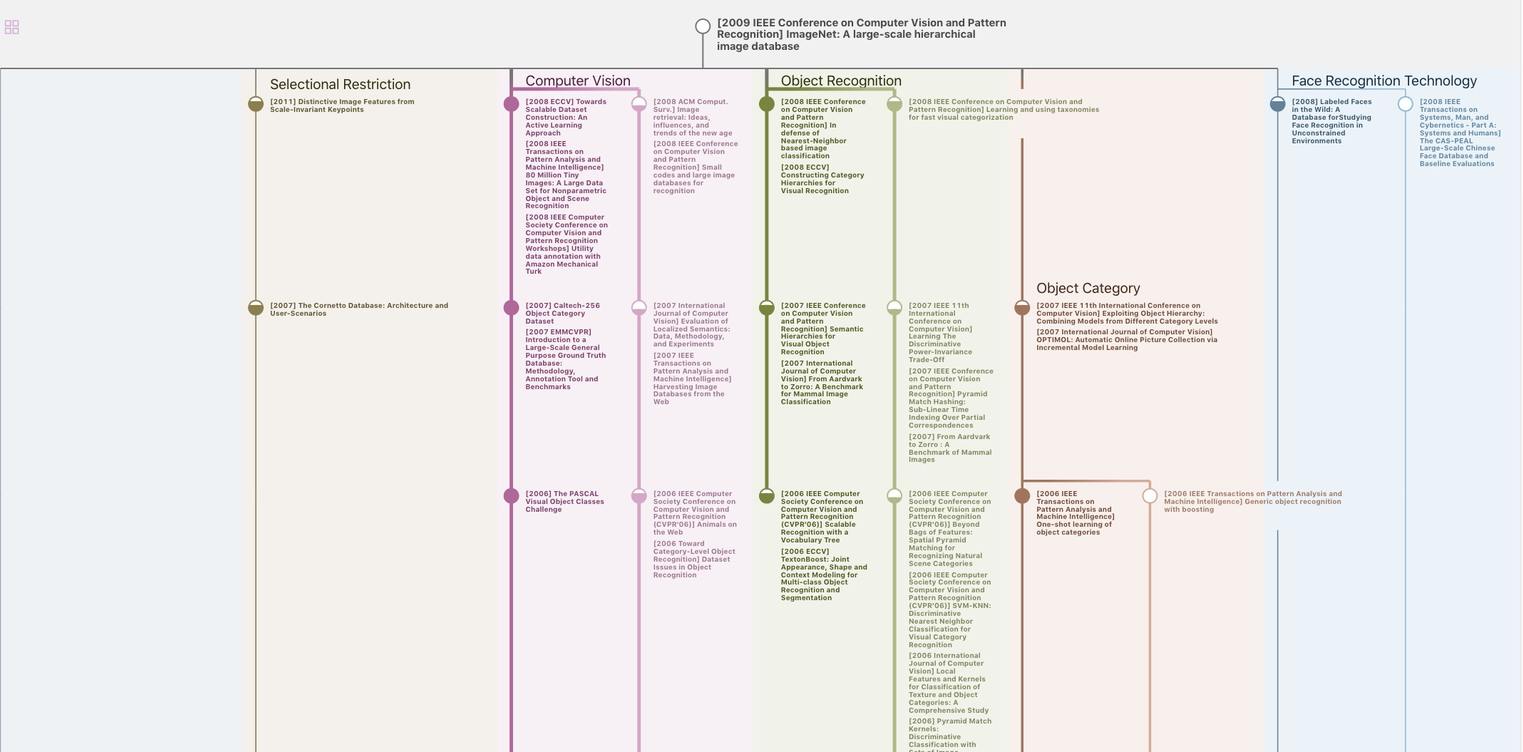
Generate MRT to find the research sequence of this paper
Chat Paper
Summary is being generated by the instructions you defined