A Privacy-Preserving Querying Mechanism with High Utility for Electric Vehicles
IEEE OPEN JOURNAL OF VEHICULAR TECHNOLOGY(2024)
摘要
Electric vehicles (EVs) are becoming more popular due to environmental consciousness. The limited availability of charging stations (CSs), compared to the number of EVs on the road, has led to increased range anxiety and a higher frequency of CS queries during trips. Simultaneously, personal data use for analytics is growing at an unprecedented rate, raising concerns for privacy. One standard for formalising location privacy is geo-indistinguishability as a generalisation of local differential privacy. However, the noise must be tuned properly, considering the implications of potential utility losses. In this paper, we introduce the notion of approximate geo-indistinguishability (AGeoI), which allows EVs to obfuscate their query locations while remaining within their area of interest. It is vital because journeys are often sensitive to a sharp drop in quality of service (QoS). Our method applies AGeoI with dummy data generation to provide two-fold privacy protection for EVs while preserving a high QoS. Analytical insights and experiments demonstrate that the majority of EVs get "privacy-for-free" and that the utility loss caused by the gain in privacy guarantees is minuscule. In addition to providing high QoS, the iterative Bayesian update allows for a private and precise CS occupancy forecast, which is crucial for unforeseen traffic congestion and efficient route planning.
更多查看译文
关键词
Privacy,Data privacy,Quality of service,Standards,Data models,Charging stations,Trajectory,Charging station,electric vehicle,geo-indistinguishability,location privacy,privacy-utility trade-off
AI 理解论文
溯源树
样例
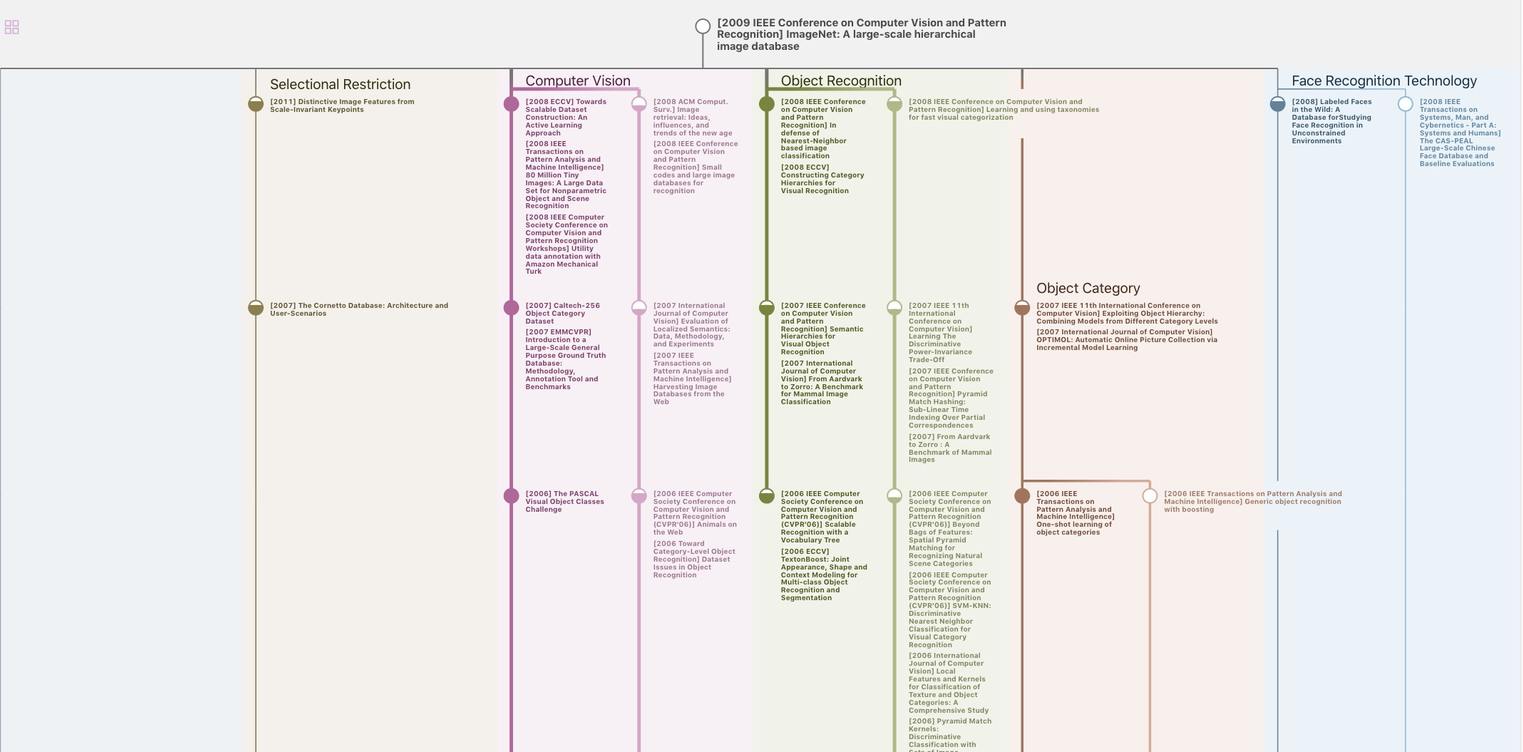
生成溯源树,研究论文发展脉络
Chat Paper
正在生成论文摘要