Estimating Counterfactual Treatment Outcomes Over Time in Complex Multiagent Scenarios
IEEE TRANSACTIONS ON NEURAL NETWORKS AND LEARNING SYSTEMS(2024)
摘要
Evaluation of intervention in a multiagent system, for example, when humans should intervene in autonomous driving systems and when a player should pass to teammates for a good shot, is challenging in various engineering and scientific fields. Estimating the individual treatment effect (ITE) using counterfactual long-term prediction is practical to evaluate such interventions. However, most of the conventional frameworks did not consider the time-varying complex structure of multiagent relationships and covariate counterfactual prediction. This may lead to erroneous assessments of ITE and difficulty in interpretation. Here, we propose an interpretable, counterfactual recurrent network in multiagent systems to estimate the effect of the intervention. Our model leverages graph variational recurrent neural networks (GVRNNs) and theory-based computation with domain knowledge for the ITE estimation framework based on long-term prediction of multiagent covariates and outcomes, which can confirm the circumstances under which the intervention is effective. On simulated models of an automated vehicle and biological agents with time-varying confounders, we show that our methods achieved lower estimation errors in counterfactual covariates and the most effective treatment timing than the baselines. Furthermore, using real basketball data, our methods performed realistic counterfactual predictions and evaluated the counterfactual passes in shot scenarios.
更多查看译文
关键词
Multi-agent systems,Behavioral sciences,Autonomous vehicles,Animals,Timing,Sports,Informatics,Autonomous vehicle,causal inference,deep generative model,multiagent modeling,sports,trajectory data
AI 理解论文
溯源树
样例
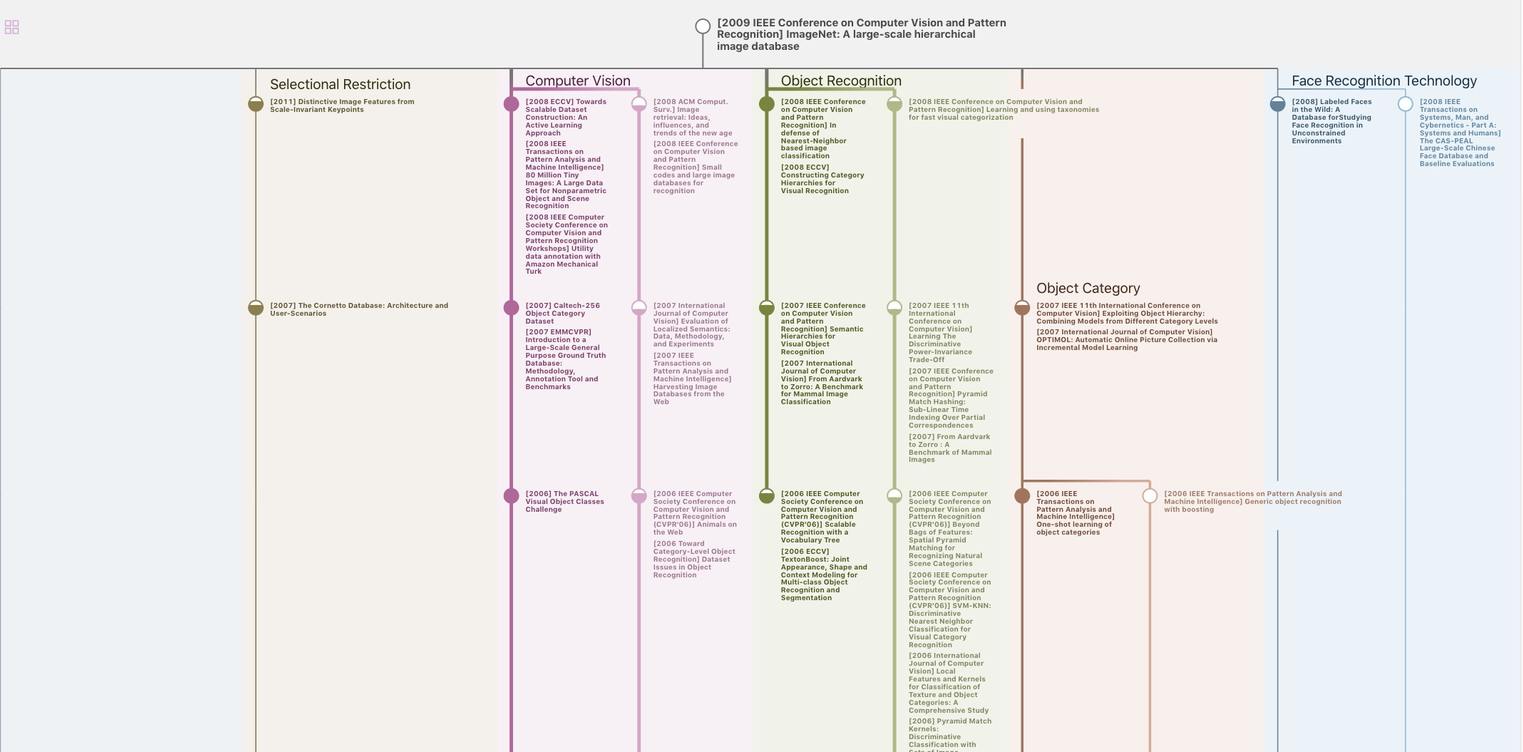
生成溯源树,研究论文发展脉络
Chat Paper
正在生成论文摘要