Model-informed generative adversarial network for learning optimal power flow
IISE TRANSACTIONS(2023)
摘要
The Optimal Power Flow (OPF) problem, as a critical component of power system operations, becomes increasingly difficult to solve due to the variability, intermittency, and unpredictability of renewable energy brought to the power system. Although traditional optimization techniques, such as stochastic and robust optimization approaches, can be leveraged to address the OPF problem, in the face of renewable energy uncertainty, i.e., the dynamic coefficients in the optimization model, their effectiveness in dealing with large-scale problems remains limited. As a result, deep learning techniques, such as neural networks, have recently been developed to improve computational efficiency in solving OPF problems with the utilization of data. However, the feasibility and optimality of the solution may not be guaranteed, and the system dynamics cannot be properly addressed as well. In this article we propose an optimization Model-Informed Generative Adversarial Network (MI-GAN) framework to solve OPF under uncertainty. The main contributions are summarized into three aspects: (i) to ensure feasibility and improve optimality of generated solutions, three important layers are proposed: feasibility filter layer, comparison layer, and gradient-guided layer; (ii) in the GAN-based framework, an efficient model-informed selector incorporating these three new layers is established; and (iii) a new recursive iteration algorithm is also proposed to improve solution optimality and handle the system dynamics. The numerical results on IEEE test systems show that the proposed method is very effective and promising.
更多查看译文
关键词
Generative adversarial network,model-informed generation,optimal power flow,power System,recursive Iteration
AI 理解论文
溯源树
样例
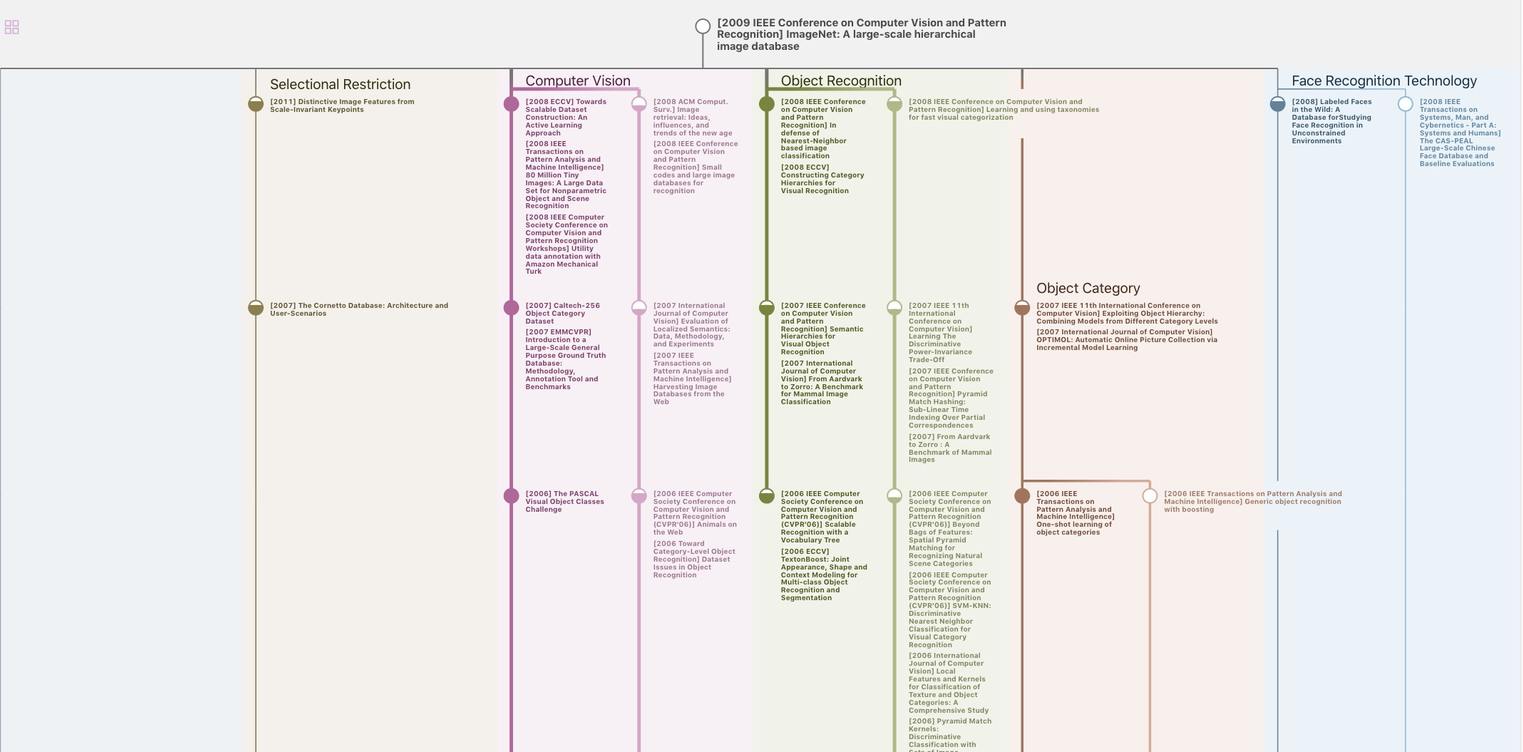
生成溯源树,研究论文发展脉络
Chat Paper
正在生成论文摘要