Torsional Diffusion for Molecular Conformer Generation
NeurIPS 2022(2022)
摘要
Molecular conformer generation is a fundamental task in computational chemistry. Several machine learning approaches have been developed, but none have outperformed state-of-the-art cheminformatics methods. We propose torsional diffusion, a novel diffusion framework that operates on the space of torsion angles via a diffusion process on the hypertorus and an extrinsic-to-intrinsic score model. On a standard benchmark of drug-like molecules, torsional diffusion generates superior conformer ensembles compared to machine learning and cheminformatics methods in terms of both RMSD and chemical properties, and is orders of magnitude faster than previous diffusion-based models. Moreover, our model provides exact likelihoods, which we employ to build the first generalizable Boltzmann generator. Code is available at https://github.com/gcorso/torsional-diffusion.
更多查看译文
关键词
conformer generation,diffusion models,score-based models,molecular structure,equivariance,geometric deep learning,Boltzmann generator
AI 理解论文
溯源树
样例
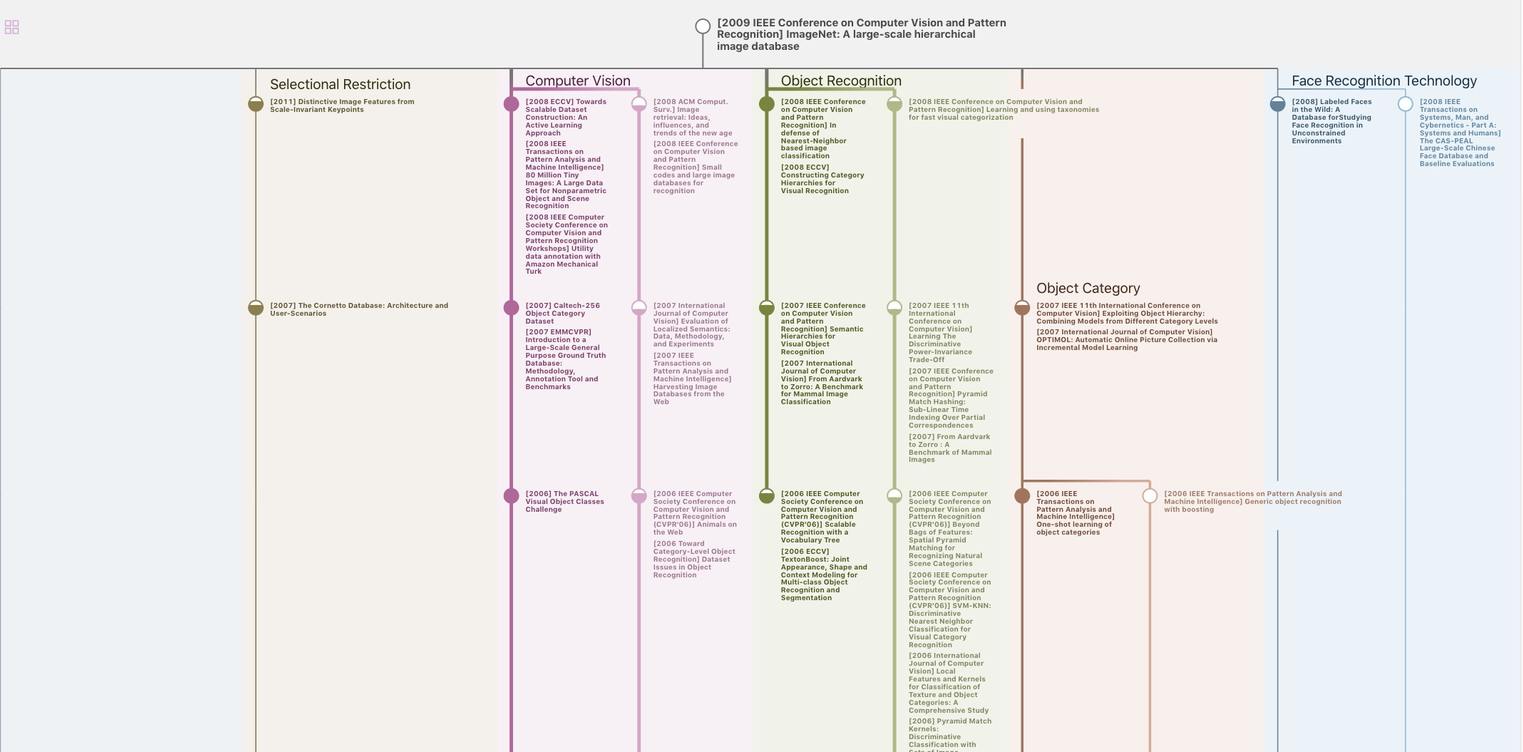
生成溯源树,研究论文发展脉络
Chat Paper
正在生成论文摘要