D'ARTAGNAN: Counterfactual Video Generation
MEDICAL IMAGE COMPUTING AND COMPUTER ASSISTED INTERVENTION, MICCAI 2022, PT VIII(2022)
摘要
Causally-enabled machine learning frameworks could help clinicians to identify the best course of treatments by answering counterfactual questions. We explore this path for the case of echocardiograms by looking into the variation of the Left Ventricle Ejection Fraction, the most essential clinical metric gained from these examinations. We combine deep neural networks, twin causal networks and generative adversarial methods for the first time to build D'ARTAGNAN (Deep ARtificial Twin-Architecture GeNerAtive Networks), a novel causal generative model. We demonstrate the soundness of our approach on a synthetic dataset before applying it to cardiac ultrasound videos to answer the question: "What would this echocardiogram look like if the patient had a different ejection fraction?". To do so, we generate new ultrasound videos, retaining the video style and anatomy of the original patient, while modifying the Ejection Fraction conditioned on a given input. We achieve an SSIM score of 0.79 and an R2 score of 0.51 on the counterfactual videos. Code and models are available at: https://github.com/HReynaud/dartagnan.
更多查看译文
关键词
Causal inference, Twin networks, Counterfactual video generation
AI 理解论文
溯源树
样例
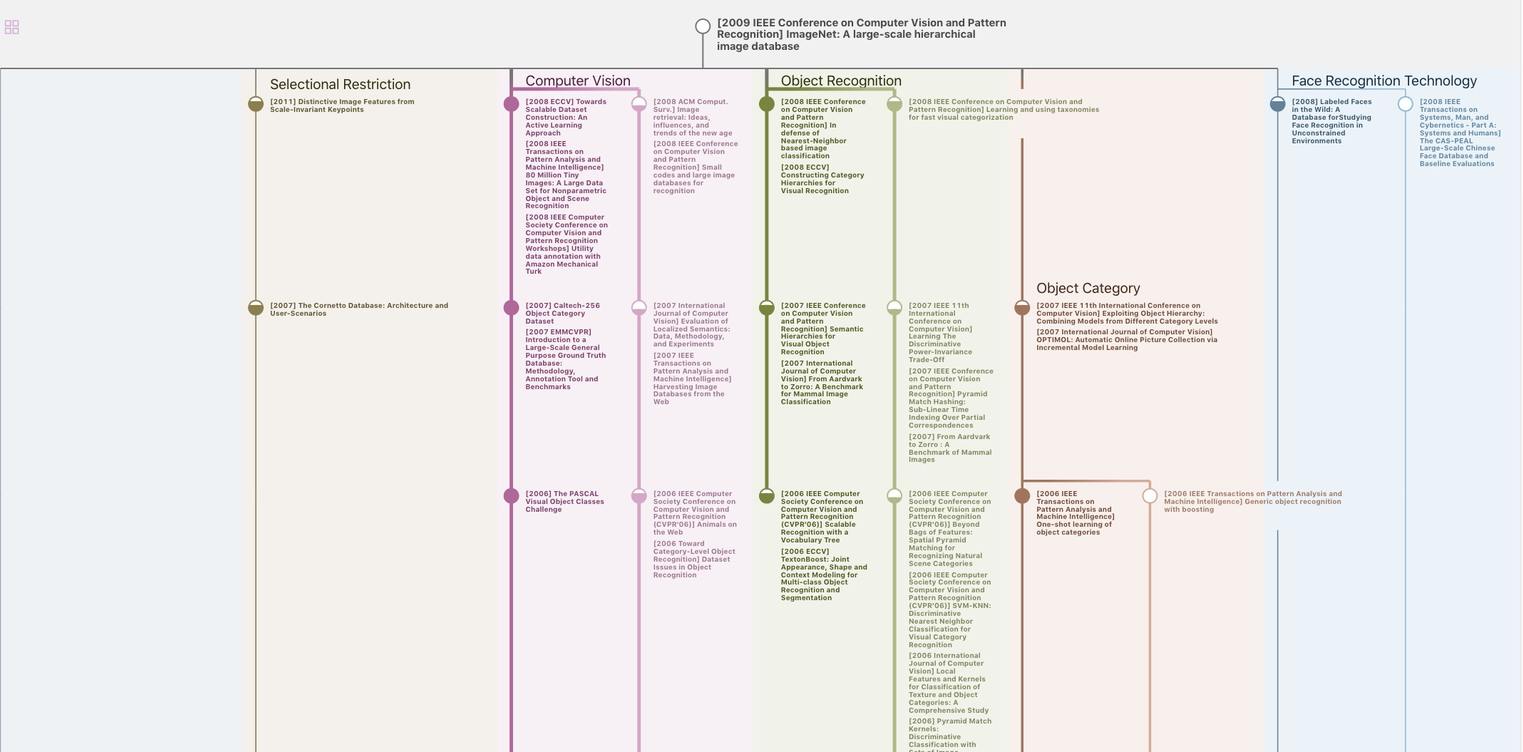
生成溯源树,研究论文发展脉络
Chat Paper
正在生成论文摘要