On Calibration of Graph Neural Networks for Node Classification
IEEE International Joint Conference on Neural Network (IJCNN)(2022)
摘要
Graphs can model real-world, complex systems by representing entities and their interactions in terms of nodes and edges. To better exploit the graph structure, graph neural networks have been developed, which learn entity and edge embeddings for tasks such as node classification and link prediction. These models achieve good performance with respect to accuracy, but the confidence scores associated with the predictions might not be calibrated. That means that the scores might not reflect the ground-truth probabilities of the predicted events, which would be especially important for safety-critical applications. Even though graph neural networks are used for a wide range of tasks, the calibration thereof has not been sufficiently explored yet. We investigate the calibration of graph neural networks for node classification, study the effect of existing post-processing calibration methods, and analyze the influence of model capacity, graph density, and a new loss function on calibration. Further, we propose a topology-aware calibration method that takes the neighboring nodes into account and yields improved calibration compared to baseline methods.
更多查看译文
关键词
Graph neural networks,calibration,node classification
AI 理解论文
溯源树
样例
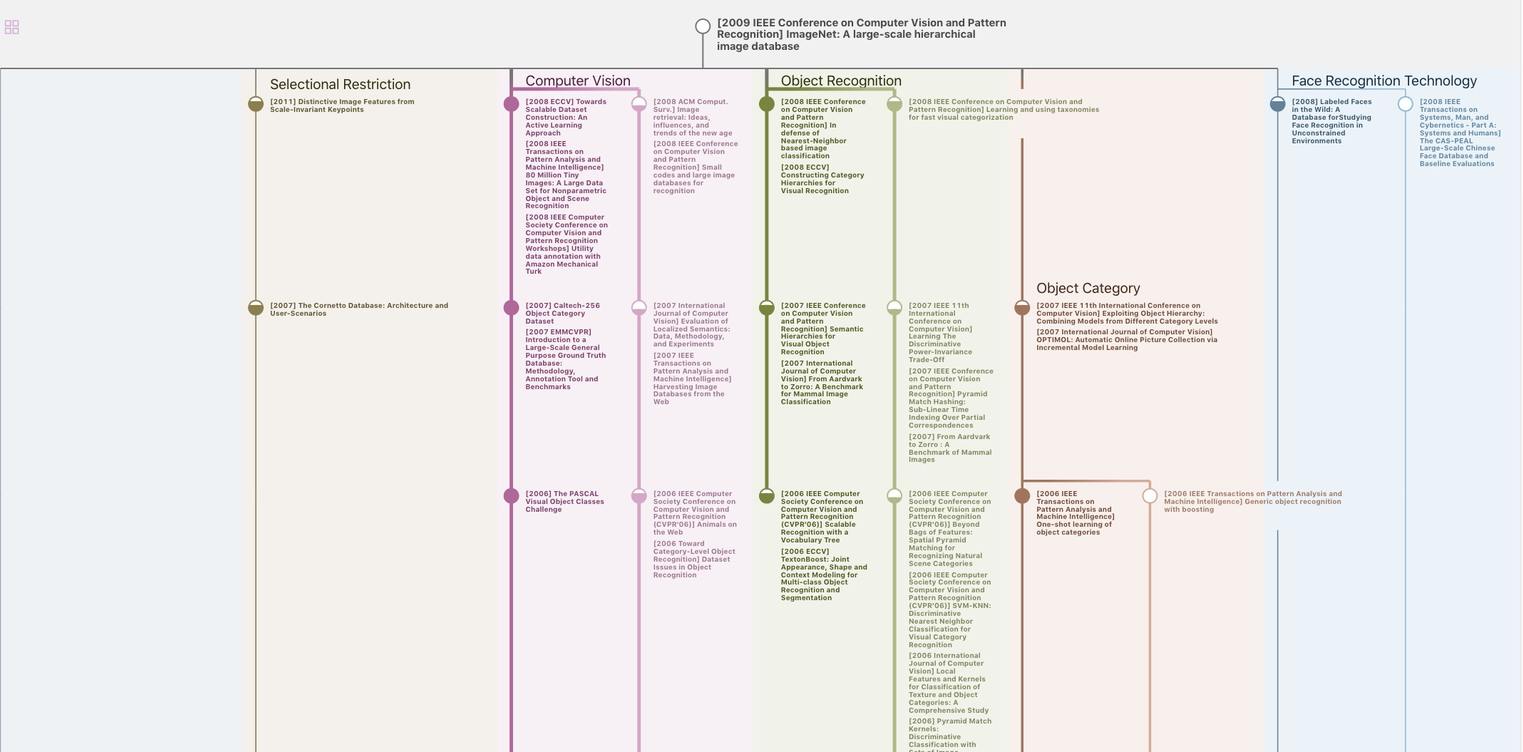
生成溯源树,研究论文发展脉络
Chat Paper
正在生成论文摘要