Learning Soft Constraints From Constrained Expert Demonstrations
ICLR 2023(2022)
摘要
Inverse reinforcement learning (IRL) methods assume that the expert data is generated by an agent optimizing some reward function. However, in many settings, the agent may optimize a reward function subject to some constraints, where the constraints induce behaviors that may be otherwise difficult to express with just a reward function. We consider the setting where the reward function is given, and the constraints are unknown, and propose a method that is able to recover these constraints satisfactorily from the expert data. While previous work has focused on recovering hard constraints, our method can recover cumulative soft constraints that the agent satisfies on average per episode. In IRL fashion, our method solves this problem by adjusting the constraint function iteratively through a constrained optimization procedure, until the agent behavior matches the expert behavior. Despite the simplicity of the formulation, our method is able to obtain good results. We demonstrate our approach on synthetic environments and real world highway driving data.
更多查看译文
关键词
inverse reinforcement learning,constraint learning
AI 理解论文
溯源树
样例
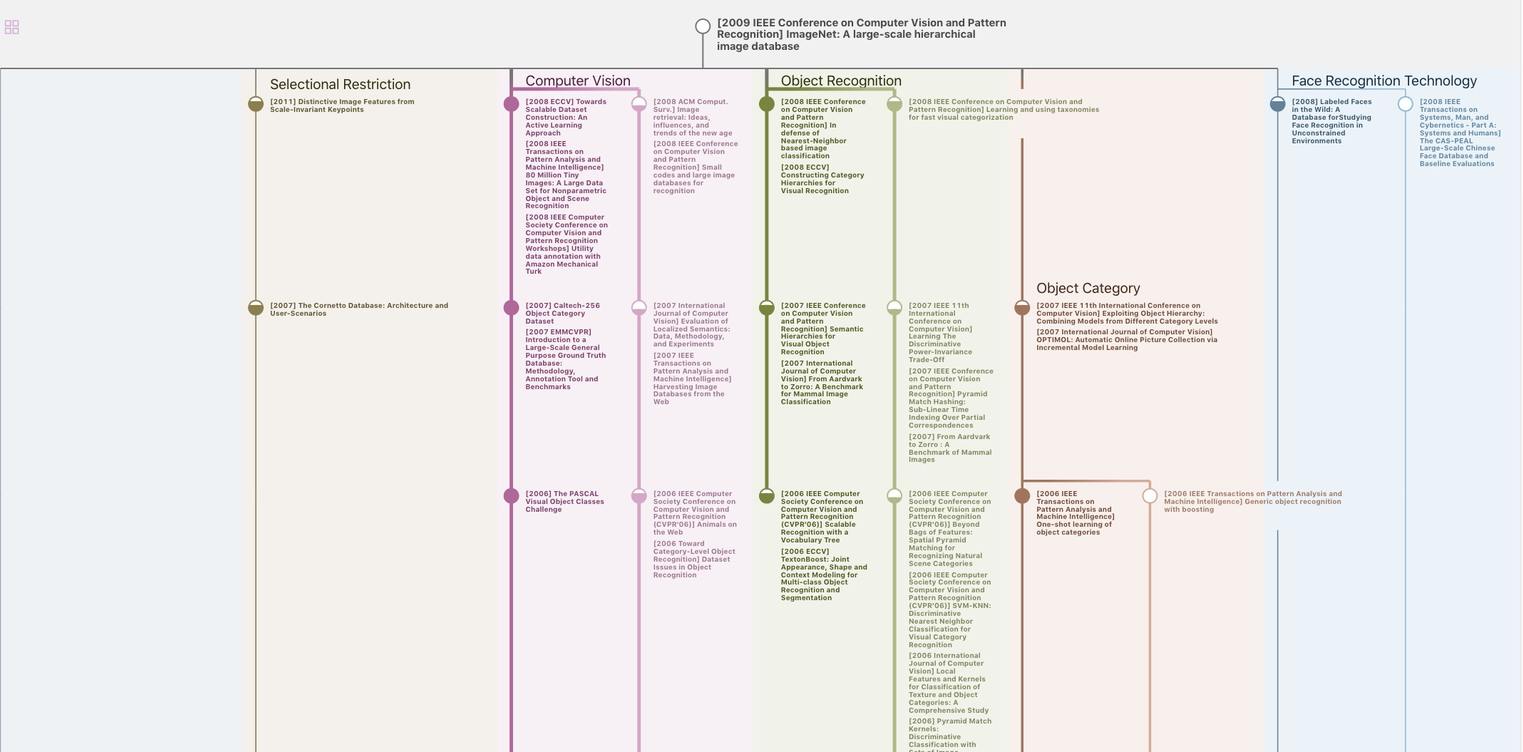
生成溯源树,研究论文发展脉络
Chat Paper
正在生成论文摘要